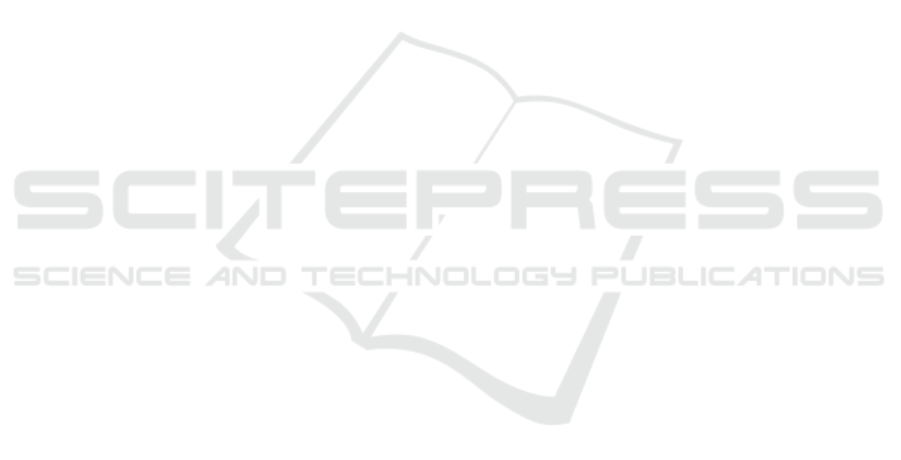
REFERENCES
Bishop, C. (2006). Pattern Recognition and Machine
Learning, volume 16, pages 140–155.
Bommasani, R., Hudson, D., Adeli, E., Altman, R., Arora,
S., Arx, S., Bernstein, M., Bohg, J., Bosselut, A.,
Brunskill, E., Brynjolfsson, E., Buch, S., Card, D.,
Castellon, R., Chatterji, N., Creel, K., Davis, J., Dem-
szky, D., and Liang, P. (2021). On the opportunities
and risks of foundation models.
Brown, T., Mann, B., Ryder, N., Subbiah, M., Kaplan, J. D.,
and Dhariwal, e. a. (2020). Language models are few-
shot learners. In Larochelle, H., Ranzato, M., Hadsell,
R., Balcan, M., and Lin, H., editors, Advances in Neu-
ral Information Processing Systems, volume 33, pages
1877–1901. Curran Associates, Inc. pp. 33-34.
Descovi, G., Maran, V., Ebling, D., and Machado, A.
(2021). Towards a blockchain architecture for animal
sanitary control. In Proceedings of the 23rd Inter-
national Conference on Enterprise Information Sys-
tems - Volume 1: ICEIS,, pages 305–312. INSTICC,
SciTePress.
Dettmers, T., Pagnoni, A., Holtzman, A., and Zettlemoyer,
L. (2024). Qlora: efficient finetuning of quantized
llms. In Proceedings of the 37th International Con-
ference on Neural Information Processing Systems,
NIPS ’23, Red Hook, NY, USA. Curran Associates
Inc.
Ebling, D., Machado, F., Descovi, G., Cardenas, N.,
Machado, G., Maran, V., and Machado, A. (2024). A
distributed processing architecture for disease spread
analysis in the pdsa-rs platform. In Proceedings of the
26th International Conference on Enterprise Informa-
tion Systems - Volume 2: ICEIS, pages 313–320. IN-
STICC, SciTePress.
Esteva, A., Kuprel, B., Novoa, R., Ko, J., Swetter, S., Blau,
H., and Thrun, S. (2017). Dermatologist-level clas-
sification of skin cancer with deep neural networks.
Nature, 542.
Gao, Y., Xiong, Y., Gao, X., Jia, K., Pan, J., Bi, Y.,
Dai, Y., Sun, J., Guo, Q., Wang, M., and Wang, H.
(2023). Retrieval-augmented generation for large lan-
guage models: A survey. ArXiv, abs/2312.10997.
Google Cloud (2023). A three-step design pattern for spe-
cializing llms. Accessed: 2024-09-13.
Gururangan, S., Marasovi
´
c, A., Swayamdipta, S., Lo, K.,
Beltagy, I., Downey, D., and Smith, N. A. (2020).
Don’t stop pretraining: Adapt language models to do-
mains and tasks. In Jurafsky, D., Chai, J., Schluter,
N., and Tetreault, J., editors, Proceedings of the 58th
Annual Meeting of the Association for Computational
Linguistics, pages 8342–8360, Online. Association
for Computational Linguistics.
Izacard, G. and Grave, E. (2021). Leveraging passage re-
trieval with generative models for open domain ques-
tion answering. In Merlo, P., Tiedemann, J., and Tsar-
faty, R., editors, Proceedings of the 16th Conference
of the European Chapter of the Association for Com-
putational Linguistics: Main Volume, pages 874–880,
Online. Association for Computational Linguistics.
Karpukhin, V., Oguz, B., Min, S., Lewis, P., Wu, L.,
Edunov, S., Chen, D., and Yih, W.-t. (2020). Dense
passage retrieval for open-domain question answer-
ing. In Webber, B., Cohn, T., He, Y., and Liu,
Y., editors, Proceedings of the 2020 Conference on
Empirical Methods in Natural Language Processing
(EMNLP), pages 6769–6781, Online. Association for
Computational Linguistics.
LeCun, Y., Bengio, Y., and Hinton, G. (2015). Deep learn-
ing. Nature, 521:436–44.
Lewis, P., Perez, E., Piktus, A., Petroni, F., Karpukhin,
V., Goyal, N., K
¨
uttler, H., Lewis, M., Yih,
W.-t., Rockt
¨
aschel, T., Riedel, S., and Kiela,
D. (2020). Retrieval-augmented generation for
knowledge-intensive nlp tasks. In Proceedings of the
34th International Conference on Neural Information
Processing Systems, NIPS ’20, Red Hook, NY, USA.
Curran Associates Inc.
Minist
´
erio da Agricultura, Pecu
´
aria e Abastecimento, S. d.
D. A. (2001). Instruc¸
˜
ao normativa nº 44, de 23 de
agosto de 2001. Accessed: 2024-10-23.
Minist
´
erio da Agricultura, Pecu
´
aria e Abastecimento, S. d.
D. A. (2003). Instruc¸
˜
ao normativa nº 78, de 3 de
novembro de 2003. Accessed: 2024-10-23.
Radford, A. and Narasimhan, K. (2018). Improving lan-
guage understanding by generative pre-training.
Sari, Y. and Indrabudiman, A. (2024). The role of artificial
intelligence (ai) in financial risk management. For-
mosa Journal of Sustainable Research, 3:2073–2082.
Schneider, R., Machado, F., Trois, C., Descovi, G., Maran,
V., and Machado, A. (2024). Speeding up the simu-
lation animals diseases spread: A study case on r and
python performance in pdsa-rs platform. In Proceed-
ings of the 26th International Conference on Enter-
prise Information Systems - Volume 2: ICEIS, pages
651–658. INSTICC, SciTePress.
Touvron, H., Lavril, T., Izacard, G., Martinet, X., Lachaux,
M.-A., Lacroix, T., Rozi
`
ere, B., Goyal, N., Hambro,
E., Azhar, F., Rodriguez, A., Joulin, A., Grave, E.,
and Lample, G. (2023). Llama: Open and efficient
foundation language models.
Vaswani, A., Shazeer, N., Parmar, N., Uszkoreit, J., Jones,
L., Gomez, A. N., Kaiser, L. u., and Polosukhin,
I. (2017). Attention is all you need. In Guyon,
I., Luxburg, U. V., Bengio, S., Wallach, H., Fer-
gus, R., Vishwanathan, S., and Garnett, R., editors,
Advances in Neural Information Processing Systems,
volume 30. Curran Associates, Inc.
Zhang, S., Dong, L., Li, X., Zhang, S., Sun, X., Wang, S.,
Li, J., Hu, R., Zhang, T., Wu, F., et al. (2023). In-
struction tuning for large language models: A survey.
arXiv preprint arXiv:2308.10792.
ICEIS 2025 - 27th International Conference on Enterprise Information Systems
500