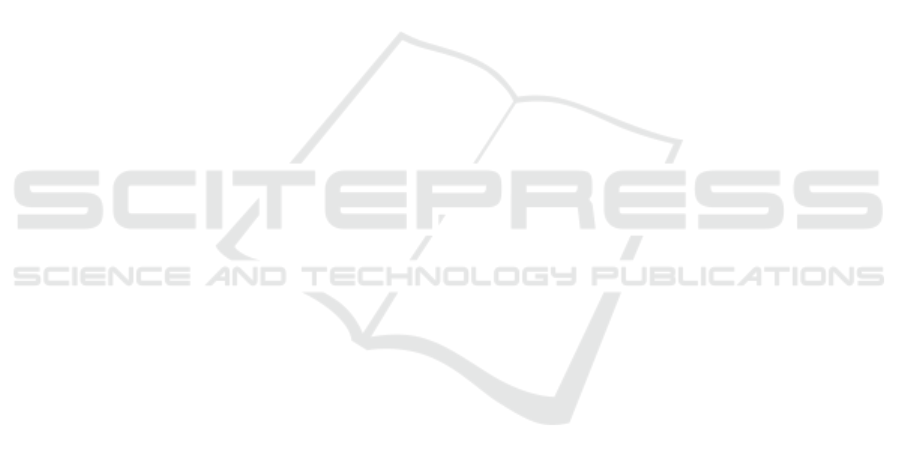
on the perceived ease of use, perceived usefulness,
and intention to use (see: Section 5). More specifi-
cally, perceived usefulness was rated the highest (see:
Section 5.2), while the perceived ease of use and in-
tention to use, though slightly lower, still produced
positive results (see: Sections 5.1 and 5.3). From
these findings, we derive that the GenArch method
has at least a moderately high level of perceived ef-
ficacy (see: Section 5.4). This suggests that the
GenArch method effectively guides the integration of
ChatGPT into the target BA product design process,
while enhancing the efficiency of this process. To an-
swer the Main Research Question, we conclude that
the GenArch method provides a structured approach
for the timely and effective utilization of ChatGPT
in the design process of target BA products. The
GenArch method positively influences the efficiency
of this design process. In summary, this research
underscores the potential of LLM tools to enhance
BA design. The findings suggest that the GenArch
method can contribute to the success of DT projects
by providing an approach that integrates ChatGPT.
Future research could include additional experi-
ments focusing on other activities or BA products that
are part of the GenArch method. This would provide a
more comprehensive understanding of how GenArch
can be utilized and optimized. Some of these addi-
tional experiments could also be executed with the
use of a pre-trained language model to observe if
results improve. Exploring strategies for adoption
of the GenArch method within organizations could
be another subject for future research. This could
positively influence the practical applicability of the
method and support a more effective integration of
GenArch in different organizational contexts. Com-
parative studies could be conducted to determine if
and how the GenArch method needs to be adapted
when utilized with an alternative LLM tool. This
could also help identify the most effective LLM tools
for specific BA design tasks or contexts. Lastly, other
potential application areas for LLM tools within DT
could be explored.
ACKNOWLEDGEMENTS
We would like to sincerely thank the Digital Trans-
formation team of KPMG Netherlands for their sup-
port during the conduction of this research, including
the provisioning of access to company documenta-
tion, domain experts, and the support provided during
the experiment.
REFERENCES
Abrah
˜
ao, S., Insfran, E., Cars
´
ı, J. A., Genero, M., and Piat-
tini, M. (2009). Evaluating the ability of novice ana-
lysts to understand requirements models. In Interna-
tional conference on quality software, pages 290–295.
IEEE.
Aguinis, H., Beltran, J. R., and Cope, A. (2024). How to
use generative AI as a HRM assistant. Organizational
Dynamics, 53(1):101029.
Alliance, T. A. (2001). Principles behind the Agile Mani-
festo. https://agilemanifesto.org/principles.html. Ac-
cessed: 13-05-2024.
Bains, C. (2023). AI prompt engineering: learn how not
to ask a chatbot a silly question. https://tinyurl.com/
4jtax73a. Accessed: 14-02-2024.
Bera, P., Wautelet, Y., and Poels, G. (2023). On the use
of ChatGPT to support agile software development.
In International Conference on Advanced Information
Systems Engineering, pages 1–9. CEUR.
Bouwman, H., Houtum van, H., Janssen, M., and Versteeg,
G. (2011). Business architectures in the public sector:
experiences from practice. Communications of the As-
sociation for Information Systems, 29:411–426.
Brinkkemper, S. (1996). Method engineering: engineer-
ing of information systems development methods and
tools. Information and Software Technology, 38:275–
280.
Chuma, E. L. and Oliveira, G. G. D. (2023). Generative
AI for business decision-making: a case of ChatGPT.
Management Science and Business Decisions, 3(1):5–
11.
Ebert, C. and Louridas, P. (2023). Generative AI for soft-
ware practitioners. IEEE Software, 40(4):30–38.
Eliot, L. (2023). Upping your prompt engineering super-
powers via target-your-response techniques when us-
ing generative AI. https://tinyurl.com/466p4h37. Ac-
cessed: 14-02-2024.
Ferrini, M. (2023). Generative AI: just a zip file of the
web? https://assets.kpmg.com/content/dam/kpmg/ch/
pdf/generative-ai.pdf. Accessed: 26-02-2024.
Fitzgerald, M., Kruschwitz, N., Bonnet, D., and Welch,
M. (2013). Embracing digital technology: A new
strategic imperative. MIT Sloan Management Review,
55(2):1–12.
Greefhorst, D. and Proper, H. A. (2011). Architecture Prin-
ciples, volume 4. Springer.
Group, T. O. (2022). Phase B: Business Architecture.
https://pubs.opengroup.org/architecture/togaf92-doc/
arch/index.html. Accessed: 01-02-2024.
Heale, R. and Twycross, A. (2018). What is a case study?
Evidence-Based Nursing, 21(1):7–8.
Hohpe, G. (2017). The Architect Elevator — Visiting
the upper floors. https://martinfowler.com/articles/
architect-elevator.html. Accessed: 16-04-2024.
Korzynski, P., Mazurek, G., Krzypkowska, P., and Kurasin-
ski, A. (2023). Artificial intelligence prompt en-
gineering as a new digital competence: Analy-
sis of generative AI technologies such as Chat-
ICEIS 2025 - 27th International Conference on Enterprise Information Systems
720