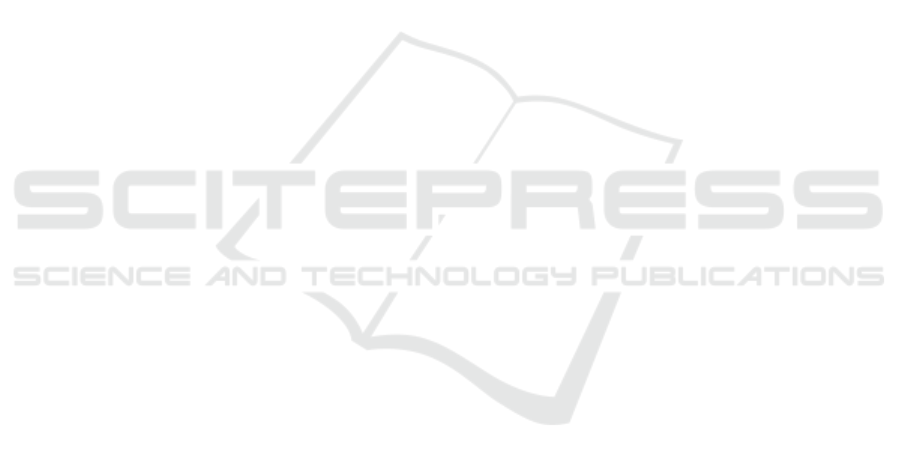
Lawrence, L. E. M., Guo, B., Yang, K., Echeverria, V.,
Kang, Z., Bathala, V., Li, C., Huang, W., Aleven, V.,
and Rummel, N. (2022). Co-designing ai-based or-
chestration tools to support dynamic transitions: De-
sign narratives through conjecture mapping. In Inter-
national Conference on Computer-Supported Collab-
orative Learning 2022, Hiroshima, Japan, pages 139–
146. International Society of the Learning Sciences.
Lin, Y. L., Lee, M. W., and Hsiao, I. H. (2018). An ex-
ploratory study on programming orchestration tech-
nology. In Proceedings of the 22nd Pacific Asia
Conference on Information Systems - Opportunities
and Challenges for the Digitized Society, Yokohama,
Japan, Proceedings of the 22nd Pacific Asia Con-
ference on Information Systems - Opportunities and
Challenges for the Digitized Society: Are We Ready?,
PACIS 2018. Association for Information Systems.
22nd Pacific Asia Conference on Information Systems
- Opportunities and Challenges for the Digitized Soci-
ety.
Lipponen, L. (2002). Exploring foundations for computer-
supported collaborative learning. In CSCL ’02: Pro-
ceedings of the Conference on Computer Support for
Collaborative Learning: Foundations for a CSCL
Community, Boulder Colorado, pages 72–81. Rout-
ledge, International Society of the Learning Sciences.
Liu, A. L. and Nesbit, J. C. (2020). Dashboards for
computer-supported collaborative learning. Machine
learning paradigms: Advances in learning analytics,
pages 157–182.
Martinez-Maldonado, R. (2019). A handheld classroom
dashboard: Teachers’ perspectives on the use of real-
time collaborative learning analytics. International
Journal of Computer-Supported Collaborative Learn-
ing, 14:383–411.
Olsen, J. K., Rummel, N., and Aleven, V. (2021). Design-
ing for the co-orchestration of social transitions be-
tween individual, small-group and whole-class learn-
ing in the classroom. International Journal of Artifi-
cial Intelligence in Education, 31(1):24–56.
Page, M., Mckenzie, J., Bossuyt, P., Boutron, I., and Hoff-
mann, T. (2021). The prisma 2020 statement: an up-
dated guideline for reporting systematic reviews. Sys-
tematic reviews, 10(1):1–11.
Praharaj, S., Scheffel, M., Schmitz, M., Specht, M., and
Drachsler, H. (2022). Towards collaborative conver-
gence: Quantifying collaboration quality with auto-
mated co-located collaboration analytics. In LAK22:
12th International Learning Analytics and Knowledge
Conference, Online, USA, page 358–369, New York,
NY, USA. Association for Computing Machinery.
Sedrakyan, G., Malmberg, J., Verbert, K., Jarvela, S., and
Kirschner, P. A. (2020). Linking learning behavior
analytics and learning science concepts: Designing a
learning analytics dashboard for feedback to support
learning regulation. Computers in Human Behavior,
107:105512.
Serrano Iglesias, S., Spikol, D., Bote Lorenzo, M. L.,
Ouhaichi, H., Gomez Sanchez, E., and Vogel, B.
(2021). Adaptable smart learning environments sup-
ported by multimodal learning analytics. In Pro-
ceedings of the LA4SLE 2021 Workshop : Learn-
ing Analytics for Smart Learning Environments co-
located with the 16th European Conference on Tech-
nology Enhanced Learning 2021 (ECTEL 2021), On-
line (Bozen-Bolzano, Italy), pages 24–30.
Silva, L., Mendes, A., Gomes, A., and Fortes, G. (2023).
Fostering regulatory processes using computational
scaffolding. International Journal of Computer-
Supported Collaborative Learning, 18(1):67–100.
Worsley, M., Anderson, K., Melo, N., and Jang, J. (2021).
Designing analytics for collaboration literacy and stu-
dent empowerment. Journal of Learning Analytics,
8(1):30–48.
Yang, K. B., Echeverria, V., Lu, Z., Mao, H., Holstein, K.,
Rummel, N., and Aleven, V. (2023). Pair-up: proto-
typing human-ai co-orchestration of dynamic transi-
tions between individual and collaborative learning in
the classroom. In Proceedings of the 2023 CHI con-
ference on human factors in computing systems CHI
2023, Hamburg, Germany. Association for Comput-
ing Machinery.
Yang, K. B., Echeverria, V., Wang, X., Lawrence, L., Hol-
stein, K., Rummel, N., and Aleven, V. (2021). Ex-
ploring policies for dynamically teaming up students
through log data simulation. International Educa-
tional Data Mining Society.
Yang, K. B., Lu, Z., Echeverria, V., Sewall, J., Lawrence,
L., Rummel, N., and Aleven, V. (2022). Technology
ecosystem for orchestrating dynamic transitions be-
tween individual and collaborative ai-tutored problem
solving. In International Conference on Artificial In-
telligence in Education, AIED 2022, Springer, Cham,
pages 673–678. Springer.
Zamecnik, A., Kovanovic, V., Grossmann, G., Joksimovic,
S., Jolliffe, G., Gibson, D., and Pardo, A. (2022).
Team interactions with learning analytics dashboards.
Computers and Education, 185:104514.
A Systematic Literature Review of Adaptive Collaborative Systems Based on Dashboards
399