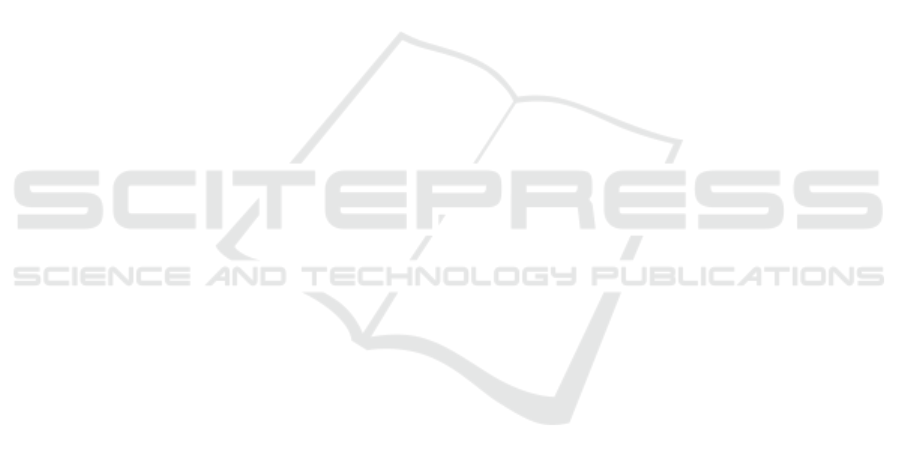
Batarseh, F. A., Mohod, R., Kumar, A., and Bui, J. (2021).
The application of artificial intelligence in software
engineering: a review challenging conventional wis-
dom.
Birru, H. (2024). Exploring the use of llms in agile technical
documentation writing. Master’s thesis, M
¨
alardalen
University, V
¨
aster
˚
as, Sweden.
Bosch, J. (2014). Continuous Software Engineering: An
Introduction, page 3–13. Springer International Pub-
lishing.
Chen, M., Tworek, J., Jun, H., Yuan, Q., Pinto, H. P. d. O.,
Kaplan, J., Edwards, H., Burda, Y., Joseph, N., Brock-
man, G., Ray, A., Puri, R., Krueger, G., Petrov, M.,
Khlaaf, H., Sastry, G., Mishkin, P., Chan, B., Gray, S.,
Ryder, N., Pavlov, M., Power, A., Kaiser, L., Bavar-
ian, M., Winter, C., Tillet, P., Such, F. P., Cummings,
D., Plappert, M., Chantzis, F., Barnes, E., Herbert-
Voss, A., Guss, W. H., Nichol, A., Paino, A., Tezak,
N., Tang, J., Babuschkin, I., Balaji, S., Jain, S., Saun-
ders, W., Hesse, C., Carr, A. N., Leike, J., Achiam,
J., Misra, V., Morikawa, E., Radford, A., Knight, M.,
Brundage, M., Murati, M., Mayer, K., Welinder, P.,
McGrew, B., Amodei, D., McCandlish, S., Sutskever,
I., and Zaremba, W. (2021). Evaluating large language
models trained on code.
Chui, M., Manyika, J., and Miremadi, M. (2016). Where
machines could replace humans-and where they can’t
(yet). The McKinsey Quarterly, pages 1–12.
Dau, A. T. V., Guo, J. L. C., and Bui, N. D. Q. (2023). Doc-
checker: Bootstrapping code large language model
for detecting and resolving code-comment inconsis-
tencies.
Feng, Z., Guo, D., Tang, D., Duan, N., Feng, X., Gong, M.,
Shou, L., Qin, B., Liu, T., Jiang, D., and Zhou, M.
(2020). Codebert: A pre-trained model for program-
ming and natural languages.
Gadesha, V. and Kavlakoglu, E. (2024). What is prompt
chaining? — IBM — ibm.com. https://www.ibm.
com/topics/prompt-chaining. [Accessed 14-05-2024].
Gao, S., Wen, X.-C., Gao, C., Wang, W., Zhang, H., and
Lyu, M. R. (2023). What makes good in-context
demonstrations for code intelligence tasks with llms?
In 2023 38th IEEE/ACM International Conference on
Automated Software Engineering (ASE). IEEE.
Haiduc, S., Aponte, J., and Marcus, A. (2010a). Supporting
program comprehension with source code summariza-
tion. In Proceedings of the 32nd ACM/IEEE Interna-
tional Conference on Software Engineering - Volume
2, ICSE ’10. ACM.
Haiduc, S., Aponte, J., Moreno, L., and Marcus, A. (2010b).
On the use of automated text summarization tech-
niques for summarizing source code. In 2010 17th
Working Conference on Reverse Engineering. IEEE.
Holscher, E. (2024). Docs as Code — writethe-
docs.org. https://www.writethedocs.org/guide/docs-
as-code/. [Accessed 28-01-2024].
Hu, X., Li, G., Xia, X., Lo, D., Lu, S., and Jin, Z. (2018).
Summarizing source code with transferred api knowl-
edge. In Proceedings of the Twenty-Seventh Inter-
national Joint Conference on Artificial Intelligence,
IJCAI-2018. International Joint Conferences on Arti-
ficial Intelligence Organization.
Husain, H., Wu, H.-H., Gazit, T., Allamanis, M., and
Brockschmidt, M. (2019). Codesearchnet challenge:
Evaluating the state of semantic code search.
Ji, Z., Lee, N., Frieske, R., Yu, T., Su, D., Xu, Y., Ishii, E.,
Bang, Y. J., Madotto, A., and Fung, P. (2023). Survey
of hallucination in natural language generation. ACM
Computing Surveys, 55(12):1–38.
Khan, J. Y. and Uddin, G. (2022). Automatic code docu-
mentation generation using gpt-3.
Khan, J. Y. and Uddin, G. (2023). Combining contexts from
multiple sources for documentation-specific code ex-
ample generation.
Li, C., Wang, J., Zhu, K., Zhang, Y., Hou, W., Lian, J.,
and Xie, X. (2023). Emotionprompt: Leveraging psy-
chology for large language models enhancement via
emotional stimulus. ArXiv, abs/2307.11760.
Liu, Z., Xia, X., Lo, D., Yan, M., and Li, S. (2023). Just-
in-time obsolete comment detection and update. IEEE
Transactions on Software Engineering, 49(1):1–23.
Lu, J., Yu, L., Li, X., Yang, L., and Zuo, C. (2023).
Llama-reviewer: Advancing code review automa-
tion with large language models through parameter-
efficient fine-tuning. In 2023 IEEE 34th International
Symposium on Software Reliability Engineering (IS-
SRE), pages 647–658.
Luo, Q., Ye, Y., Liang, S., Zhang, Z., Qin, Y., Lu, Y., Wu,
Y., Cong, X., Lin, Y., Zhang, Y., Che, X., Liu, Z., and
Sun, M. (2024). Repoagent: An llm-powered open-
source framework for repository-level code documen-
tation generation.
Mastropaolo, A., Cooper, N., Palacio, D. N., Scalabrino,
S., Poshyvanyk, D., Oliveto, R., and Bavota, G.
(2023). Using transfer learning for code-related
tasks. IEEE Transactions on Software Engineering,
49(4):1580–1598.
Meta (2024). Introducing Meta Llama 3: The most capable
openly available LLM to date — ai.meta.com. https:
//ai.meta.com/blog/meta-llama-3/. [Accessed 03-06-
2024].
Moore, J., Gelman, B., and Slater, D. (2019). A convo-
lutional neural network for language-agnostic source
code summarization. In Proceedings of the 14th In-
ternational Conference on Evaluation of Novel Ap-
proaches to Software Engineering, ENASE 2019,
page 15–26, Setubal, PRT. SCITEPRESS - Science
and Technology Publications, Lda.
Moreno, L., Aponte, J., Sridhara, G., Marcus, A., Pollock,
L., and Vijay-Shanker, K. (2013). Automatic genera-
tion of natural language summaries for java classes. In
2013 21st International Conference on Program Com-
prehension (ICPC). IEEE.
Nguyen-Duc, A., Cabrero-Daniel, B., Przybylek, A., Arora,
C., Khanna, D., Herda, T., Rafiq, U., Melegati, J.,
Guerra, E., Kemell, K.-K., Saari, M., Zhang, Z., Le,
H., Quan, T., and Abrahamsson, P. (2023). Genera-
tive artificial intelligence for software engineering – a
research agenda.
Supporting Automated Documentation Updates in Continuous Software Development with Large Language Models
105