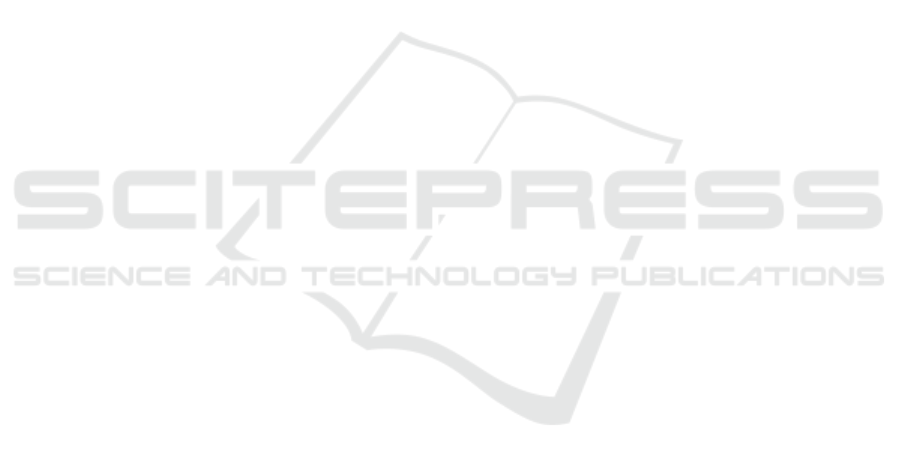
marks that can be detected in practice using exist-
ing technology, but the imprecision of the position
of these landmarks has been artificially defined. In
the longer term, our goal is to use the measurements
made by individual vehicles as crowd-sourced data
for improving the map. In such a system, the vehicles
send all the data that they obtained during a day to a
central server that will consolidate and process them
in order to produce a new landmarks map that will
be sent back to all vehicles. The goal is to improve
on the work of (Stoven-Dubois, 2022) to handle city-
scale maps. Eventually, this will lead to a methodol-
ogy for the creation of precise landmark maps from
coarse ones without resorting to expensive solutions
like GNSS RTK.
REFERENCES
Alsayed, Z., Bresson, G., Nashashibi, F., and Verroust-
Blondet, A. (2015). PML-SLAM: a solution for local-
ization in large-scale urban environments. In PPNIV-
IROS 2015.
Bresson, G., Alsayed, Z., Yu, L., and Glaser, S. (2017).
Simultaneous localization and mapping: A survey of
current trends in autonomous driving. IEEE Transac-
tions on Intelligent Vehicles, 2(3):194–220.
Breuel, T. M. (2003). Implementation techniques for geo-
metric branch-and-bound matching methods. Com-
puter Vision and Image Understanding, 90(3):258–
294.
B
¨
uchner, M., Z
¨
urn, J., Todoran, I.-G., Valada, A., and
Burgard, W. (2023). Learning and aggregating lane
graphs for urban automated driving. In Proceedings
of the IEEE/CVF Conference on Computer Vision and
Pattern Recognition, pages 13415–13424.
Chalvatzaras, A., Pratikakis, I., and Amanatiadis, A. A.
(2022). A survey on map-based localization tech-
niques for autonomous vehicles. IEEE Transactions
on intelligent vehicles, 8(2):1574–1596.
Chen, S., Zhang, N., and Sun, H. (2020). Collaborative lo-
calization based on traffic landmarks for autonomous
driving. In 2020 IEEE International Symposium on
Circuits and Systems (ISCAS), pages 1–5. IEEE.
Dissanayake, G., Williams, S. B., Durrant-Whyte, H., and
Bailey, T. (2002). Map management for efficient si-
multaneous localization and mapping (SLAM). Au-
tonomous Robots, 12:267–286.
Durrant-Whyte, H. and Bailey, T. (2006). Simultaneous lo-
calization and mapping: part I. IEEE robotics & au-
tomation magazine, 13(2):99–110.
Elghazaly, G., Frank, R., Harvey, S., and Safko, S. (2023).
High-definition maps: Comprehensive survey, chal-
lenges and future perspectives. IEEE Open Journal
of Intelligent Transportation Systems.
He, L., Jiang, S., Liang, X., Wang, N., and Song, S.
(2022). Diff-net: Image feature difference based high-
definition map change detection for autonomous driv-
ing. In 2022 International Conference on Robotics
and Automation (ICRA), pages 2635–2641. IEEE.
Kalman, R. E. (1960). A new approach to linear filtering
and prediction problems. Journal of Basic Engineer-
ing, 82(1):35–45.
Kumar, D. and Muhammad, N. (2023). A survey on local-
ization for autonomous vehicles. IEEE Access.
Li, L., Wang, R., and Zhang, X. (2021). A tutorial review
on point cloud registrations: principle, classification,
comparison, and technology challenges. Mathemati-
cal Problems in Engineering, 2021(1):9953910.
Nashashibi, F. (2000).
𝑅𝑇
𝑚𝑎 𝑝𝑠: a framework for proto-
typing automotive multi-sensor applications. In IEEE
Proceedings of the IEEE Intelligent Vehicles Sympo-
sium 2000, pages 99–103. IEEE.
Neira, J., Tard
´
os, J. D., and Castellanos, J. A. (2003). Lin-
ear time vehicle relocation in SLAM. In ICRA, pages
427–433.
Qu, X., Soheilian, B., and Paparoditis, N. (2018). Landmark
based localization in urban environment. ISPRS Jour-
nal of Photogrammetry and Remote Sensing, 140:90–
103.
Ruan, J., Li, B., Wang, Y., and Sun, Y. (2023). Slamesh:
Real-time lidar simultaneous localization and mesh-
ing. In 2023 IEEE International Conference on
Robotics and Automation (ICRA), pages 3546–3552.
IEEE.
Stoven-Dubois, A. (2022). Robust Crowdsourced Mapping
for Landmarks-based Vehicle Localization. PhD the-
sis, Universit
´
e Clermont Auvergne.
Sun, S., Jelfs, B., Ghorbani, K., Matthews, G., and Gilliam,
C. (2022). Landmark management in the application
of radar SLAM. In 2022 Asia-Pacific Signal and In-
formation Processing Association Annual Summit and
Conference (APSIPA ASC), pages 903–910. IEEE.
Weishaupt, F., Tilly, J. F., Appenrodt, N., Fischer, P., Dick-
mann, J., and Heberling, D. (2024). Landmark-based
vehicle self-localization using automotive polarimet-
ric radars. IEEE Transactions on Intelligent Trans-
portation Systems.
Zhang, Y., Severinsen, O. A., Leonard, J. J., Carlone,
L., and Khosoussi, K. (2023). Data-association-
free landmark-based SLAM. In 2023 IEEE In-
ternational Conference on Robotics and Automation
(ICRA), pages 8349–8355. IEEE.
Z
¨
urn, J., Vertens, J., and Burgard, W. (2021). Lane graph
estimation for scene understanding in urban driving.
IEEE Robotics and Automation Letters, 6(4):8615–
8622.
Landmark-Based Geopositioning with Imprecise Map
481