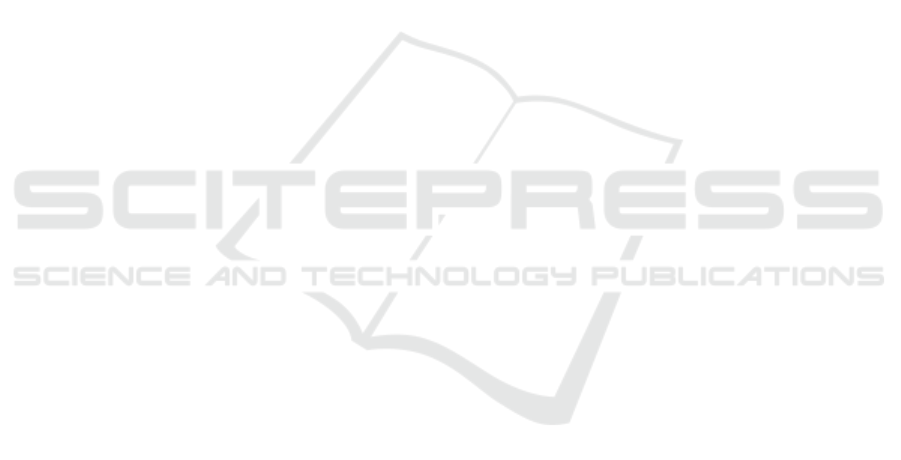
iobank, physiotoolkit, and physionet: components of
a new research resource for complex physiologic sig-
nals. circulation, 101(23):e215–e220.
Hemakom, A., Atiwiwat, D., and Israsena, P. (2023). Ecg
and eeg based detection and multilevel classification
of stress using machine learning for specified genders:
A preliminary study. Plos one, 18(9):e0291070.
Hochreiter, S. and Schmidhuber, J. (1997). Long short-term
memory. Neural computation, 9(8):1735–1780.
Jiang, J.-R., Kao, J.-B., and Li, Y.-L. (2021). Semi-
supervised time series anomaly detection based on
statistics and deep learning. Applied Sciences,
11(15):6698.
Law, S. M. (2019). Stumpy: A powerful and scalable
python library for time series data mining. Journal
of Open Source Software, 4(39):1504.
Li, H. and Boulanger, P. (2020). A survey of heart anomaly
detection using ambulatory electrocardiogram (ecg).
Sensors, 20(5):1461.
Linardi, M., Zhu, Y., Palpanas, T., and Keogh, E. (2020).
Matrix profile goes mad: variable-length motif and
discord discovery in data series. Data Mining and
Knowledge Discovery, 34:1022–1071.
Madrid, F., Imani, S., Mercer, R., Zimmerman, Z., Shak-
ibay, N., and Keogh, E. (2019). Matrix profile
xx: Finding and visualizing time series motifs of all
lengths using the matrix profile. In 2019 IEEE ICBK,
pages 175–182. IEEE.
Makowski, D., Pham, T., Lau, Z. J., Brammer, J. C.,
Lespinasse, F., Pham, H., Sch
¨
olzel, C., and Chen,
S. A. (2021). Neurokit2: A python toolbox for neu-
rophysiological signal processing. Behavior research
methods, pages 1–8.
Malhotra, P., Ramakrishnan, A., Anand, G., Vig, L., Agar-
wal, P., and Shroff, G. (2016). Lstm-based encoder-
decoder for multi-sensor anomaly detection. arXiv
preprint arXiv:1607.00148.
Malhotra, P., Vig, L., Shroff, G., Agarwal, P., et al. (2015).
Long short term memory networks for anomaly detec-
tion in time series. In ESANN, volume 2015, page 89.
McInnes, L., Healy, J., and Astels, S. (2017). hdbscan: Hi-
erarchical density based clustering. J. Open Source
Softw., 2(11):205.
Moody, G. B. and Mark, R. G. (2001). The impact of
the mit-bih arrhythmia database. IEEE engineering
in medicine and biology magazine, 20(3):45–50.
Mueen, A. (2014). Time series motif discovery: dimen-
sions and applications. Wiley Int. Rev. Data Min. and
Knowl. Disc., 4(2):152–159.
Noering, F. K.-D., Schroeder, Y., Jonas, K., and Klawonn,
F. (2021). Pattern discovery in time series using au-
toencoder in comparison to nonlearning approaches.
Integrated Computer-Aided Engineering, 28(3):237–
256.
Torkamani, S. and Lohweg, V. (2017). Survey on time series
motif discovery. WIREs Data Mining and Knowledge
Discovery, 7(2):e1199. e1199 DMKD-00255.R2.
Toyoda, M., Sakurai, Y., and Ishikawa, Y. (2013). Pattern
discovery in data streams under the time warping dis-
tance. The VLDB Journal, 22:295–318.
Wang, H., Luo, Z., Yip, J. W., Ye, C., and Zhang, M. (2023).
Ecggan: A framework for effective and interpretable
electrocardiogram anomaly detection. In Proceedings
of the 29th ACM SIGKDD, pages 5071–5081.
Wang, H., Zhou, Y., Zhou, B., Niu, X., Zhang, H., and
Wang, Z. (2021). Interactive ecg annotation: An arti-
ficial intelligence method for smart ecg manipulation.
Information Sciences, 581:42–59.
Wu, L., Jiang, Z., Meulendijks, E. R., Baylan, U., Waas,
I. S., Bugiani, M., Tuinman, P. R., Fronczek, J., He-
unks, L. M., de Groot, J. R., van Rossum, A. C.,
Niessen, H. W., and Krijnen, P. A. (2023). Atrial
inflammation and microvascular thrombogenicity are
increased in deceased covid-19 patients. Cardiovas-
cular Pathology, 64:107524.
Yeh, C.-C. M., Chen, H., Fan, Y., Dai, X., Zheng, Y., Lai,
V., Wang, J., Zhuang, Z., Wang, L., Zhang, W., et al.
(2023). Ego-network transformer for subsequence
classification in time series data. In 2023 IEEE In-
ternational Conference on Big Data (BigData), pages
1242–1247. IEEE.
Yeh, C.-C. M., Zhu, Y., Ulanova, L., Begum, N., Ding, Y.,
Dau, H. A., Silva, D. F., Mueen, A., and Keogh, E.
(2016). Matrix profile i: all pairs similarity joins for
time series: a unifying view that includes motifs, dis-
cords and shapelets. In 2016 IEEE 16th ICDM, pages
1317–1322. Ieee.
ICEIS 2025 - 27th International Conference on Enterprise Information Systems
250