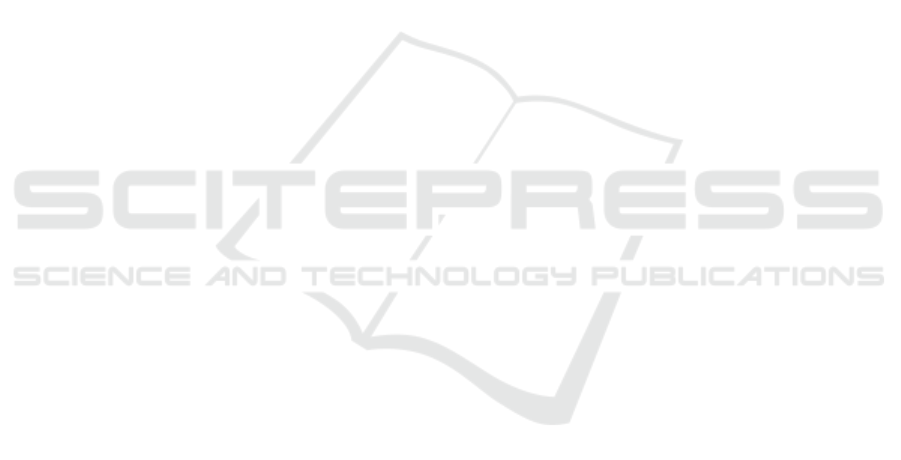
in figure 4 and 5 above show the topic distributions
for authors in the two datasets: 20 Newsgroups and
NIPS. Each row represents an author, while each col-
umn corresponds to a topic. The intensity of the color
indicates the strength of association between the au-
thor and the respective topic. In the 20 Newsgroups
dataset, we see some authors strongly aligned with
particular topics, as indicated by the darker shades.
Similarly, the NIPS dataset heatmap reveals vary-
ing topic preferences among the authors, showcasing
some strong associations to specific topics, especially
by authors such as Oriol Vinyals and Fangjian Guo.
These visualizations help understand the thematic fo-
cus of different authors.
5 CONCLUSION
We proposed ABLiMA, an author-topic modeling ap-
proach, by integrating the Beta-Liouville, allowing
greater flexibility in capturing the variability and spar-
sity of author-specific thematic focus. Through ex-
periments, the model demonstrated its ability to ex-
tract meaningful topic distributions, reflected in co-
herent topic clusters and insightful author-topic rela-
tionships. Visualizations like heatmaps and coherence
scores further validated the effectiveness of the model
in distinguishing distinct topic preferences among au-
thors. Future work could focus on optimizing hy-
perparameter estimation techniques and incorporating
automatic inference of the optimal number of topics
such as Dirichlet Process-based models.
REFERENCES
Ali, S. and Bouguila, N. (2019). Variational learning of
beta-liouville hidden markov models for infrared ac-
tion recognition. In 2019 IEEE/CVF Conference on
Computer Vision and Pattern Recognition Workshops
(CVPRW), pages 898–906.
Bakhtiari, A. S. and Bouguila, N. (2014). Online learning
for two novel latent topic models. In Linawati, Ma-
hendra, M. S., Neuhold, E. J., Tjoa, A. M., and You,
I., editors, Information and Communication Technol-
ogy - Second IFIP TC5/8 International Conference,
ICT-EurAsia 2014, Proceedings, volume 8407 of Lec-
ture Notes in Computer Science, pages 286–295, Bali,
Indonesia. Springer.
Bakhtiari, A. S. and Bouguila, N. (2016). A latent beta-
liouville allocation model. Expert Systems with Appli-
cations, 45:260–272.
Blei, D. M. and Lafferty, J. D. (2007). A correlated topic
model of science. The Annals of Applied Statistics,
1:17–35.
Blei, D. M., Ng, A. Y., and Jordan, M. I. (2003). Latent
dirichlet allocation. Journal of Machine Learning Re-
search, 3(Jan):993–1022.
Bouguila, N. (2009). A model-based approach for discrete
data clustering and feature weighting using map and
stochastic complexity. IEEE Transactions on Knowl-
edge and Data Engineering, 21(12):1649–1664.
Bouguila, N. (2012). Infinite liouville mixture models with
application to text and texture categorization. Pattern
Recognit. Lett., 33(2):103–110.
Chang, J., Gerrish, S., Wang, C., Boyd-Graber, J. L., and
Blei, D. M. (2009). Reading tea leaves: How humans
interpret topic models. In Advances in Neural Infor-
mation Processing Systems (NIPS), pages 288–296.
Ennajari, H., Bouguila, N., and Bentahar, J. (2021). Com-
bining knowledge graph and word embeddings for
spherical topic modeling. IEEE Transactions on
Neural Networks and Learning Systems, 34(7):3609–
3623.
Epaillard, E. and Bouguila, N. (2016). Proportional data
modeling with hidden markov models based on gen-
eralized dirichlet and beta-liouville mixtures applied
to anomaly detection in public areas. Pattern Recog-
nit., 55:125–136.
Fan, W. and Bouguila, N. (2013a). Learning finite beta-
liouville mixture models via variational bayes for pro-
portional data clustering. In Rossi, F., editor, Pro-
ceedings of the 23rd International Joint Conference
on Artificial Intelligence (IJCAI), pages 1323–1329,
Beijing, China. IJCAI/AAAI.
Fan, W. and Bouguila, N. (2013b). Online learning of
a dirichlet process mixture of beta-liouville distri-
butions via variational inference. IEEE Transac-
tions on Neural Networks and Learning Systems,
24(11):1850–1862.
Fan, W. and Bouguila, N. (2015). Expectation propaga-
tion learning of a dirichlet process mixture of beta-
liouville distributions for proportional data cluster-
ing. Engineering Applications of Artificial Intelli-
gence, 43:1–14.
Griffiths, T. L. and Steyvers, M. (2004). Finding scientific
topics. Proceedings of the National Academy of Sci-
ences, 101(suppl 1):5228–5235.
Luo, Z., Amayri, M., Fan, W., and Bouguila, N. (2023).
Cross-collection latent beta-liouville allocation model
training with privacy protection and applications.
Appl. Intell., 53(14):17824–17848.
Mimno, D., Wallach, H. M., Talley, E., Leenders, M., and
McCallum, A. (2012). Optimizing semantic coher-
ence in topic models. In Proceedings of the Confer-
ence on Empirical Methods in Natural Language Pro-
cessing (EMNLP), pages 262–272.
Rosen-Zvi, M., Griffiths, T., Steyvers, M., and Smyth, P.
(2004). The author-topic model for authors and doc-
uments. In Proceedings of the 20th Conference on
Uncertainty in Artificial Intelligence, pages 487–494.
Zamzami, N. and Bouguila, N. (2020). High-dimensional
count data clustering based on an exponential approx-
imation to the multinomial beta-liouville distribution.
Inf. Sci., 524:116–135.
ICEIS 2025 - 27th International Conference on Enterprise Information Systems
258