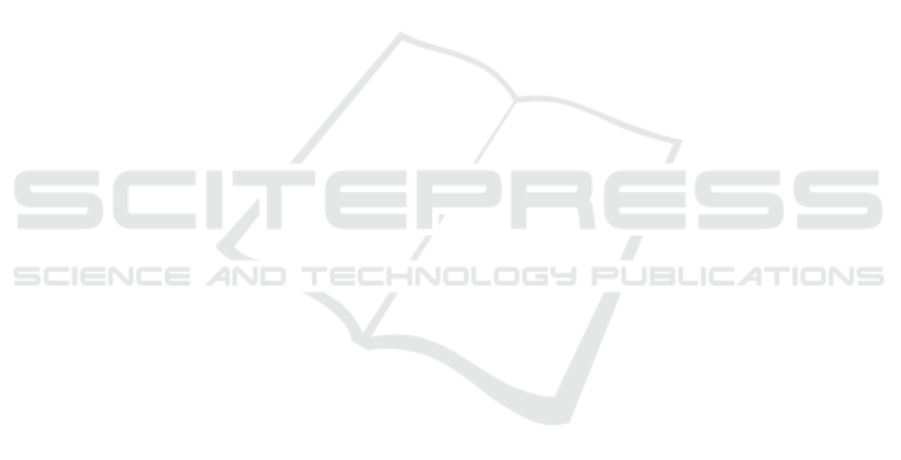
Carion, N., Massa, F., Synnaeve, G., Usunier, N., Kir-
illov, A., and Zagoruyko, S. (2020). End-to-end ob-
ject detection with transformers. In Computer Vi-
sion – 16th European Conference, Proceedings Part I,
volume 12346, pages 213–229. Springer International
Publishing and Imprint Springer.
Ester, M., Kriegel, H.-P., Sander, J., and Xu, X. (1996).
A density-based algorithm for discovering clusters in
large spatial databases with noise. In KDD’96: Pro-
ceedings of the Second International Conference on
Knowledge Discovery and Data Mining, pages 226–
231. AAAI Press.
Ettinger, S., Cheng, S., Caine, B., Liu, C., Zhao, H., Prad-
han, S., Chai, Y., Sapp, B., Qi, C., Zhou, Y., Yang, Z.,
Chouard, A., Sun, P., Ngiam, J., Vasudevan, V., Mc-
Cauley, A., Shlens, J., and Anguelov, D. (2021). Large
scale interactive motion forecasting for autonomous
driving : The waymo open motion dataset. In 2021
IEEE/CVF International Conference on Computer Vi-
sion, Proceedings, pages 9690–9699. IEEE.
Gilles, T., Sabatini, S., Tsishkou, D., Stanciulescu, B.,
and Moutarde, F. (2021). Home: Heatmap output
for future motion estimation. In 2021 IEEE Interna-
tional Intelligent Transportation Systems Conference
(ITSC), pages 500–507. IEEE.
Gilles, T., Sabatini, S., Tsishkou, D., Stanciulescu, B.,
and Moutarde, F. (2022a). Gohome: Graph-oriented
heatmap output for future motion estimation. In 2022
IEEE International Conference on Robotics and Au-
tomation (ICRA), pages 9107–9114. IEEE.
Gilles, T., Sabatini, S., Tsishkou, D., Stanciulescu, B., and
Moutarde, F. (2022b). THOMAS: Trajectory heatmap
output with learned multi-agent sampling. In Interna-
tional Conference on Learning Representations.
Goodfellow, I., Pouget-Abadie, J., Mirza, M., Xu, B.,
Warde-Farley, D., Ozair, S., Courville, A., and Ben-
gio, Y. (2020). Generative adversarial networks. Com-
mun. ACM, 63:139–144.
Gulzar, M., Muhammad, Y., and Muhammad, N. (2021). A
survey on motion prediction of pedestrians and vehi-
cles for autonomous driving. IEEE Access, 9:137957–
137969.
Guo, Y., Kalidindi, V. V., Arief, M., Wang, W., Zhu, J.,
Peng, H., and Zhao, D. (2019). Modeling multi-
vehicle interaction scenarios using gaussian random
field. In 2019 IEEE Intelligent Transportation Sys-
tems Conference, pages 3974–3980. IEEE.
Gupta, A., Johnson, J., Fei-Fei, L., Savarese, S., and
Alahi, A. (2018). Social gan: Socially acceptable
trajectories with generative adversarial networks. In
2018 IEEE/CVF Conference on Computer Vision and
Pattern Recognition, Proceedings, pages 2255–2264.
IEEE.
Huang, R., Zhuo, G., Xiong, L., Lu, S., and Tian, W. (2023).
A review of deep learning-based vehicle motion pre-
diction for autonomous driving. Sustainability, page
14716.
Huang, Y., Du, J., Yang, Z., Zhou, Z., Zhang, L., and Chen,
H. (2022). A survey on trajectory-prediction methods
for autonomous driving. IEEE Transactions on Intel-
ligent Vehicles, 7(3):652–674.
Hussain, R. and Zeadally, S. (2019). Autonomous cars: Re-
search results, issues, and future challenges. IEEE
Communications Surveys & Tutorials, 21(2):1275–
1313.
Jain, A., Casas, S., Liao, R., Xiong, Y., Feng, S., Segal,
S., and Urtasun, R. (2019). Discrete residual flow for
probabilistic pedestrian behavior prediction. In Con-
ference on Robot Learning.
Karle, P., Geisslinger, M., Betz, J., and Lienkamp, M.
(2022). Scenario understanding and motion predic-
tion for autonomous vehicles—review and compari-
son. IEEE Transactions on Intelligent Transportation
Systems, 23(10):16962–16982.
Kosaraju, V., Sadeghian, A., Mart
´
ın-Mart
´
ın, R., Reid,
I., Rezatofighi, S. H., and Savarese, S. (2019).
Social-BiGAT: multimodal trajectory forecasting us-
ing bicycle-GAN and graph attention networks. Cur-
ran Associates Inc.
Lan, Z., Jiang, Y., Mu, Y., Chen, C., and Li, S. E. (2024).
SEPT: Towards efficient scene representation learning
for motion prediction. In The Twelfth International
Conference on Learning Representations.
Lange, B., Itkina, M., and Kochenderfer, M. J. (2021). At-
tention augmented convlstm for environment predic-
tion. 2021 IEEE/RSJ International Conference on
Intelligent Robots and Systems (IROS), pages 1346–
1353.
Lefevre, S., Vasquez, D., and Laugier, C. (2014). A survey
on motion prediction and risk assessment for intelli-
gent vehicles. Robomech Journal, 1.
Lin, L., Lin, X., Lin, T., Huang, L., Xiong, R., and
Wang, Y. (2024). Eda: Evolving and distinct an-
chors for multimodal motion prediction. Proceed-
ings of the AAAI Conference on Artificial Intelligence,
38(4):3432–3440.
Loshchilov, I. and Hutter, F. (2019). Decoupled weight
decay regularization. In International Conference on
Learning Representations.
Luo, K., Casas, S., Liao, R., Yan, X., Xiong, Y., Zeng, W.,
and Urtasun, R. (2021). Safety-oriented pedestrian oc-
cupancy forecasting. In 2021 IEEE/RSJ International
Conference on Intelligent Robots and Systems (IROS),
pages 1015–1022.
Mahjourian, R., Kim, J., Chai, Y., Tan, M., Sapp, B.,
and Anguelov, D. (2022). Occupancy flow fields
for motion forecasting in autonomous driving. IEEE
Robotics and Automation Letters, 7(2):5639–5646.
Mangalam, K., An, Y., Girase, H., and Malik, J. (2021).
From goals, waypoints & paths to long term human
trajectory forecasting. In 2021 IEEE/CVF Interna-
tional Conference on Computer Vision, Proceedings,
pages 15213–15222. IEEE.
McLachlan, G. J. and Basford, K. E. (1988). Mixture
models: Inference and applications to clustering, vol-
ume 84. Dekker.
Mohamed, A., Zhu, D., Vu, W., Elhoseiny, M., and Claudel,
C. (2022). Social-implicit: Rethinking trajectory pre-
diction evaluation and the effectiveness of implicit
maximum likelihood estimation. In Computer Vision
Exploring Shared Gaussian Occupancies for Tracking-Free, Scene-Centric Pedestrian Motion Prediction in Autonomous Driving
111