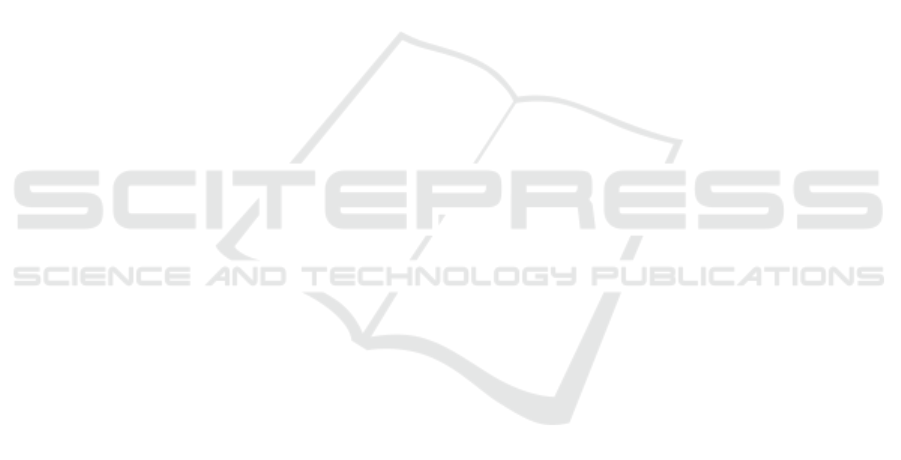
formers for language understanding. arXiv preprint
arXiv:1810.04805.
Gousios, G., Pinzger, M., and Deursen, A. v. (2014). An
exploratory study of the pull-based software develop-
ment model. In Proceedings of the 36th international
conference on software engineering, pages 345–355.
Gousios, G., Storey, M.-A., and Bacchelli, A. (2016). Work
practices and challenges in pull-based development:
The contributor’s perspective. In Proceedings of the
38th International Conference on Software Engineer-
ing, pages 285–296.
Gousios, G. and Zaidman, A. (2014). A dataset for pull-
based development research. In Proceedings of the
11th Working Conference on Mining Software Repos-
itories, pages 368–371.
Gousios, G., Zaidman, A., Storey, M.-A., and Van Deursen,
A. (2015). Work practices and challenges in pull-
based development: The integrator’s perspective. In
2015 IEEE/ACM 37th IEEE International Conference
on Software Engineering, volume 1, pages 358–368.
IEEE.
Jiang, J., Lo, D., He, J., Xia, X., Kochhar, P. S., and Zhang,
L. (2017). Why and how developers fork what from
whom in github. Empirical Software Engineering,
22:547–578.
Kalliamvakou, E., Gousios, G., Blincoe, K., Singer, L., Ger-
man, D., and Damian, D. (2016). An in-depth study
of the promises and perils of mining github. Empirical
Software Engineering, 21(4):2035–2071.
Kalliamvakou, E., Gousios, G., Blincoe, K., Singer, L., Ger-
man, D. M., and Damian, D. (2014). The promises and
perils of mining github. In Proceedings of the 11th
working conference on mining software repositories,
pages 92–101.
Lazar, A., Ritchey, S., and Sharif, B. (2014). Improving
the accuracy of duplicate bug report detection using
textual similarity measures. In Proceedings of the
11th Working Conference on Mining Software Repos-
itories, pages 308–311.
Li, L., Ren, Z., Li, X., Zou, W., and Jiang, H. (2018). How
are issue units linked? empirical study on the linking
behavior in github. In 2018 25th Asia-Pacific Soft-
ware Engineering Conference (APSEC), pages 386–
395. IEEE.
Li, Z., Yin, G., Yu, Y., Wang, T., and Wang, H. (2017).
Detecting duplicate pull-requests in github. In Pro-
ceedings of the 9th Asia-Pacific symposium on inter-
netware, pages 1–6.
Li, Z., Yu, Y., Wang, T., Lei, Y., Wang, Y., and Wang, H.
(2022). To follow or not to follow: Understanding
issue/pull-request templates on github. IEEE Trans-
actions on Software Engineering, 49(4):2530–2544.
Li, Z., Yu, Y., Zhou, M., Wang, T., Yin, G., Lan, L., and
Wang, H. (2020). Redundancy, context, and prefer-
ence: An empirical study of duplicate pull requests
in oss projects. IEEE Transactions on Software Engi-
neering, 48(4):1309–1335.
Ma, X., Wang, Z., Ng, P., Nallapati, R., and Xiang, B.
(2019). Universal text representation from bert: An
empirical study. arXiv preprint arXiv:1910.07973.
Manning, C. and Schutze, H. (1999). Foundations of statis-
tical natural language processing. MIT press.
McClean, K., Greer, D., and Jurek-Loughrey, A. (2021).
Social network analysis of open source software: A
review and categorisation. Information and Software
Technology, 130:106442.
Mombach, T. and Valente, M. T. (2018). Github rest api vs
ghtorrent vs github archive: A comparative study.
Muennighoff, N., Tazi, N., Magne, L., and Reimers, N.
(2022). Mteb: Massive text embedding benchmark.
arXiv preprint arXiv:2210.07316.
Reimers, N. and Gurevych, I. (2019). Sentence-bert: Sen-
tence embeddings using siamese bert-networks. arXiv
preprint arXiv:1908.10084.
Runeson, P., Alexandersson, M., and Nyholm, O. (2007).
Detection of duplicate defect reports using natural lan-
guage processing. In 29th International Conference
on Software Engineering (ICSE’07), pages 499–510.
IEEE.
Taylor, P. S., Greer, D., Sage, P., Coleman, G., McDaid,
K., Lawthers, I., and Corr, R. (2006). Applying
an agility/discipline assessment for a small software
organisation. In Product-Focused Software Process
Improvement: 7th International Conference, PRO-
FES 2006, Amsterdam, The Netherlands, June 12-14,
2006. Proceedings 7, pages 290–304. Springer.
Wang, Q., Xu, B., Xia, X., Wang, T., and Li, S. (2019).
Duplicate pull request detection: When time matters.
In Proceedings of the 11th Asia-Pacific symposium on
internetware, pages 1–10.
Wang, W., Wei, F., Dong, L., Bao, H., Yang, N., and Zhou,
M. (2020). Minilm: Deep self-attention distillation for
task-agnostic compression of pre-trained transform-
ers.
Wessel, M., Vargovich, J., Gerosa, M. A., and Treude, C.
(2023). Github actions: the impact on the pull request
process. Empirical Software Engineering, 28(6):1–35.
Yu, Y., Li, Z., Yin, G., Wang, T., and Wang, H. (2018). A
dataset of duplicate pull-requests in github. In Pro-
ceedings of the 15th international conference on min-
ing software repositories, pages 22–25.
Zhang, X., Chen, Y., Gu, Y., Zou, W., Xie, X., Jia, X., and
Xuan, J. (2018). How do multiple pull requests change
the same code: A study of competing pull requests
in github. In 2018 IEEE International Conference on
Software Maintenance and Evolution (ICSME), pages
228–239. IEEE.
Zhou, S., Vasilescu, B., and Kästner, C. (2019). What the
fork: A study of inefficient and efficient forking prac-
tices in social coding. In Proceedings of the 2019 27th
ACM joint meeting on european software engineering
conference and symposium on the foundations of soft-
ware engineering, pages 350–361.
Detecting Duplicate Effort in GitHub Contributions
529