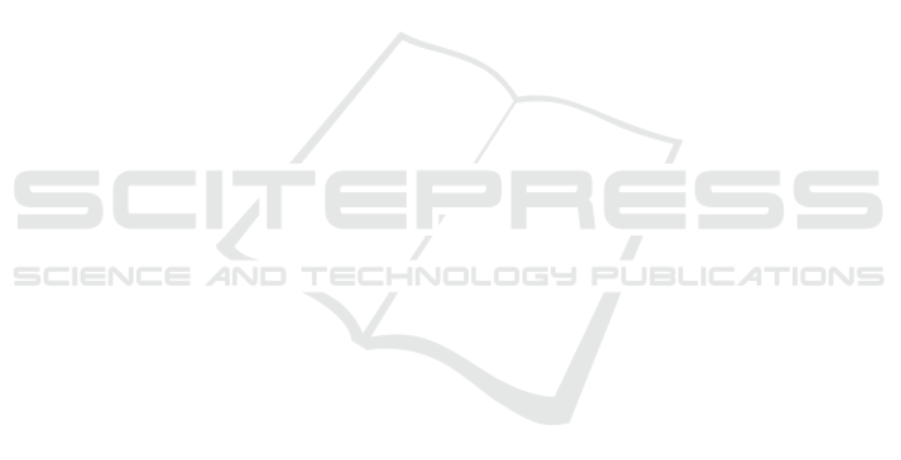
ments, WACI-HJ achieves gains of 2% in CPU, 27%
in RAM, and 18% in I/O utilization. Testing with a
real-world dataset shows a 54% improvement in PCR
and 10% in QET. Energy-efficiency experiments con-
firm gains of 1% in CPU, 38% in RAM, and 49% in
I/O. By optimizing data access and incorporating pre-
dictive capabilities, WACI-HJ reduces query latency
and conserves energy, making it ideal for resource-
constrained edge applications.
REFERENCES
Google Colab. Available: https://colab.research.google.
com/ Accessed: June, 2024.
IoT Data Growth. Available: https://www.gartner.com/en/
documents/3996804 Accessed: June, 2024.
IoT Devices Growth. Available: https://www.statista.com/
statistics/1183457/iot-connected-devices-worldwide/
#:
∼
:text=The%20number%20of%20Internet%20of,
billion%20IoT%20devices%20in%202030. Ac-
cessed: June, 2024.
MARTA Dataset. Available: https://data.world/
brentbrewington/marta-hackathon Accessed: June,
2024.
MonetDB. Available: https://www.monetdb.org/ Accessed:
June, 2024.
TPC-D Dataset. Available: https://github.com/gunaprsd/
SkewedDataGenerator Accessed: June, 2024.
TPC-H Dataset. Available: http://www.tpc.org/tpch/ Ac-
cessed: June, 2024.
VScode. Available: https://code.visualstudio.com/ Ac-
cessed: June, 2024.
Awaysheh, F. M., Tommasini, R., and Awad, A. (2023). Big
data analytics from the rich cloud to the frugal edge.
In 2023 IEEE International Conference on Edge Com-
puting and Communications (EDGE), pages 319–329.
IEEE.
Barber, R., Lohman, G., Pandis, I., Raman, V., Sidle, R.,
Attaluri, G., Chainani, N., Lightstone, S., and Sharpe,
D. (2014). Memory-efficient hash joins. Proceedings
of the VLDB Endowment, 8(4):353–364.
Bilal, K., Khalid, O., Erbad, A., and Khan, S. U. (2018).
Potentials, trends, and prospects in edge technologies:
Fog, cloudlet, mobile edge, and micro data centers.
Computer Networks, 130:94–120.
Calheiros, R. N., Masoumi, E., Ranjan, R., and Buyya, R.
(2014). Workload prediction using arima model and
its impact on cloud applications’ qos. IEEE transac-
tions on cloud computing, 3(4):449–458.
Chen, S., Ailamaki, A., Gibbons, P. B., and Mowry, T. C.
(2007). Improving hash join performance through
prefetching. ACM Transactions on Database Systems
(TODS), 32(3):17–es.
Gandhi, K. and Bhise, M. (2019). Affinity-based fragmen-
tation for sensor data. In 2019 IEEE 16th India Coun-
cil International Conference (INDICON), pages 1–4.
IEEE.
Gandhi, K., Pandat, A., and Bhise, M. (2021). Ex-
periments on static data summarization techniques.
In 2021 IEEE International Women in Engineering
(WIE) Conference on Electrical and Computer Engi-
neering (WIECON-ECE), pages 17–20. IEEE.
Li, G., Yao, Y., Wu, J., Liu, X., Sheng, X., and Lin, Q.
(2020). A new load balancing strategy by task alloca-
tion in edge computing based on intermediary nodes.
EURASIP Journal on Wireless Communications and
Networking, 2020:1–10.
Li, Y. and Xu, W. (2021). Utilizing the column imprints
to accelerate no-partitioning hash joins in large-scale
edge systems. Transactions on Emerging Telecommu-
nications Technologies, 32(6):1–17.
Ma, L., Van Aken, D., Hefny, A., Mezerhane, G., Pavlo,
A., and Gordon, G. J. (2018). Query-based workload
forecasting for self-driving database management sys-
tems. In Proceedings of the 2018 International Con-
ference on Management of Data, pages 631–645.
Meruje Ferreira, L. M., Coelho, F., and Pereira, J. (2024).
Databases in edge and fog environments: A survey.
ACM Computing Surveys, 56(11):1–40.
Patel, M. and Bhise, M. (2023). Muar: Maximizing uti-
lization of available resources for query processing.
In 2023 IEEE/ACM 23rd International Symposium
on Cluster, Cloud and Internet Computing Workshops
(CCGridW), pages 176–183. IEEE.
Schmidt, T., Kipf, A., Horn, D., Saxena, G., and Kraska,
T. (2024). Predicate caching: Query-driven secondary
indexing for cloud data warehouses. In Proceedings
of the 2024 ACM SIGMOD International Conference
on Management of Data, pages 347–359.
Shah, K., Gandhi, A., Gandhi, K., and Bhise, M. (2024).
Workload prediction for edge computing. In Pro-
ceedings of the 25th International Conference on Dis-
tributed Computing and Networking, pages 286–291.
Sun, X. and Ansari, N. (2017). Green cloudlet network:
A distributed green mobile cloud network. IEEE net-
work, 31(1):64–70.
Symeonides, M., Trihinas, D., Georgiou, Z., Pallis, G., and
Dikaiakos, M. (2019). Query-driven descriptive ana-
lytics for iot and edge computing. In 2019 IEEE In-
ternational Conference on Cloud Engineering (IC2E),
pages 1–11. IEEE.
Vitorovic, A., Elseidy, M., and Koch, C. (2016). Load bal-
ancing and skew resilience for parallel joins. In 2016
IEEE 32nd International Conference on Data Engi-
neering (ICDE), pages 313–324. Ieee.
Xu, R., Palanisamy, B., and Joshi, J. (2018). Queryguard:
Privacy-preserving latency-aware query optimization
for edge computing. In 2018 17th IEEE International
Conference On Trust, Security And Privacy In Com-
puting And Communications/12th IEEE International
Conference On Big Data Science And Engineering
(TrustCom/BigDataSE), pages 1097–1106. IEEE.
ICEIS 2025 - 27th International Conference on Enterprise Information Systems
266