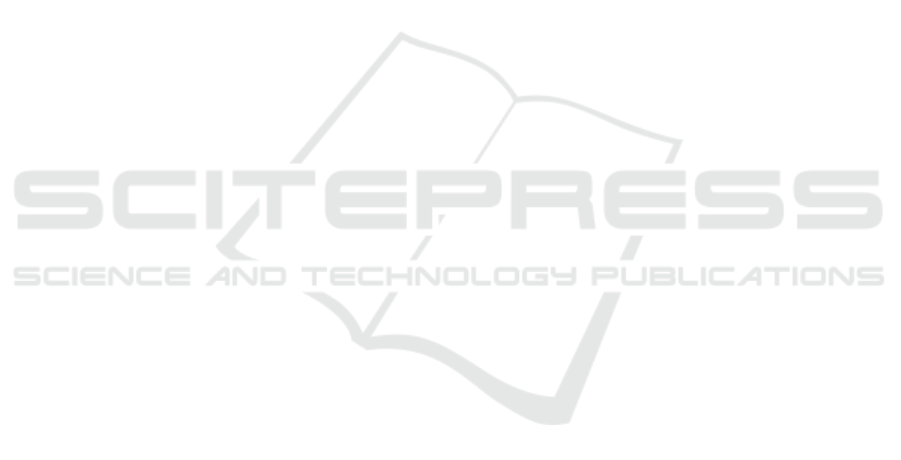
cedures, and expanding the methodology to other dis-
eases. Future studies on the evaluation of PM
2
HC for
subpopulation analysis are needed to advance the sci-
entific body of knowledge.
ACKNOWLEDGEMENTS
We would like to express our gratitude to the physio-
therapists at Fysiotherapie Polman in Enschede (the
Netherlands) for their valuable discussions and in-
sights on the frozen shoulder case study.
REFERENCES
Alrawabdeh, J., Alzu’bi, M., Alzyoud, M., Odeh, N.,
Hamadneh, Y., Mian, H., Mohyuddin, G. R., Kelkar,
A. H., Goodman, A. M., Chakraborty, R., Russler-
Germain, D. A., Mehra, N., Baggio, D., Cliff, E.
R. S., and Al Hadidi, S. (2023). Characteristics of
post hoc subgroup analyses of oncology clinical tri-
als: a systematic review. JNCI Cancer Spectrum,
7(6):pkad100.
Amatya, A. K., Fiero, M. H., Bloomquist, E. W., Sinha,
A. K., Lemery, S. J., Singh, H., Ibrahim, A.,
Donoghue, M., Fashoyin-Aje, L. A., de Claro, R. A.,
Gormley, N. J., Amiri-Kordestani, L., Sridhara, R.,
Theoret, M. R., Kluetz, P. G., Pazdur, R., Beaver, J. A.,
and Tang, S. (2021). Subgroup analyses in oncology
trials: Regulatory considerations and case examples.
Clinical Cancer Research, 27(21):5753–5756.
Aspland, E., Gartner, D., and Harper, P. (2021). Clinical
pathway modelling: a literature review. Health Sys-
tems, 10(1):1–23.
Becker, A.-K., D
¨
orr, M., Felix, S. B., Frost, F., Grabe, H. J.,
Lerch, M. M., Nauck, M., V
¨
olker, U., V
¨
olzke, H., and
Kaderali, L. (2021). From heterogeneous healthcare
data to disease-specific biomarker networks: A hier-
archical bayesian network approach. PLoS computa-
tional biology, 17(2):e1008735.
Bogar
´
ın, A., Cerezo, R., and Romero, C. (2018). Discover-
ing learning processes using inductive miner: A case
study with learning management systems (lmss). Psi-
cothema, 30:322–329.
Campbell, S. K. (2013). Use of care paths to improve pa-
tient management. Physical & Occupational Therapy
in Pediatrics, 33(1):27–38.
Chen, R. J., Wang, J. J., Williamson, D. F., Chen, T. Y.,
Lipkova, J., Lu, M. Y., Sahai, S., and Mahmood, F.
(2023). Algorithmic fairness in artificial intelligence
for medicine and healthcare. Nature Biomedical En-
gineering, 7(6):719–742.
Dasaradharami Reddy, K. and Gadekallu, T. R. (2023).
A comprehensive survey on federated learning tech-
niques for healthcare informatics. Computational In-
telligence and Neuroscience, 2023(1):8393990.
de Boer, T. R., Arntzen, R. J., Bekker, R., Buurman, B. M.,
Willems, H. C., and van der Mei, R. D. (2024). Pro-
cess mining on national health care data for the dis-
covery of patient journeys of older adults. Journal
of the American Medical Directors Association, page
105333.
D’Orsi, G. M., Via, A. G., Frizziero, A., and Oliva, F.
(2012). Treatment of adhesive capsulitis: A review.
Muscles, ligaments and tendons journal, 2(2):70–78.
Evans-Lacko, S., Jarrett, M., McCrone, P., and Thornicroft,
G. (2010). Facilitators and barriers to implementing
clinical care pathways. BMC Health Services Re-
search, 10:1–6.
Goldberger, A., Amaral, L., Glass, L., Hausdorff, J.,
Ivanov, P., Mark, R., Mietus, J., Moody, G., Peng, C.,
and Stanley, H. (2000). PhysioBank, PhysioToolkit,
and PhysioNet: Components of a new research re-
source for complex physiologic signals. circulation,
101(23):e215–e220.
Gonzalez, R. A. and Sol, H. G. (2012). Validation and de-
sign science research in information systems. In Re-
search methodologies, innovations and philosophies
in software systems engineering and information sys-
tems, pages 403–426. IGI Global.
Guo, C. and Chen, J. (2023). Big data analytics in health-
care. In Knowledge technology and systems: Toward
establishing knowledge systems science, pages 27–70.
Springer.
Guzzo, A., Rullo, A., and Vocaturo, E. (2022). Process
mining applications in the healthcare domain: A com-
prehensive review. Wiley Interdisciplinary Reviews:
Data Mining and Knowledge Discovery, 12(2):e1442.
Huang, Z., Lu, X., Duan, H., and Fan, W. (2013). Summa-
rizing clinical pathways from event logs. Journal of
Biomedical Informatics, 46(1):111–127.
Ivanov, S. Y., Kalenkova, A. A., and Aalst, W. M. P. (2015).
BPMNDiffViz: A tool for BPMN models comparison.
1418:35–39.
Johnson, A., Bulgarelli, L., Pollard, T., Horng, S., Celi,
L. A., and Mark, R. (2021). MIMIC-IV (version 1.0).
Johnson, A. E., Bulgarelli, L., Shen, L., Gayles, A., Sham-
mout, A., Horng, S., Pollard, T. J., Hao, S., Moody,
B., Gow, B., et al. (2023). Mimic-iv, a freely acces-
sible electronic health record dataset. Scientific Data,
10(1):1.
Koorevaar, R., Riet, E., Ipskamp, M., and Bulstra, S.
(2017). Incidence and prognostic factors for post-
operative frozen shoulder after shoulder surgery: A
prospective cohort study. Archives of Orthopaedic and
Trauma Surgery, 137.
Ma, F., Ye, M., Luo, J., Xiao, C., and Sun, J. (2021). Ad-
vances in mining heterogeneous healthcare data. In
Proceedings of the 27th ACM SIGKDD Conference on
Knowledge Discovery & Data Mining, pages 4050–
4051.
Mans, R. S., Schonenberg, M. H., Song, M., van der Aalst,
W. M. P., and Bakker, P. J. M. (2009). Applica-
tion of process mining in healthcare–a case study in
a dutch hospital. In Biomedical Engineering Systems
and Technologies, pages 425–438. Springer.
ICEIS 2025 - 27th International Conference on Enterprise Information Systems
276