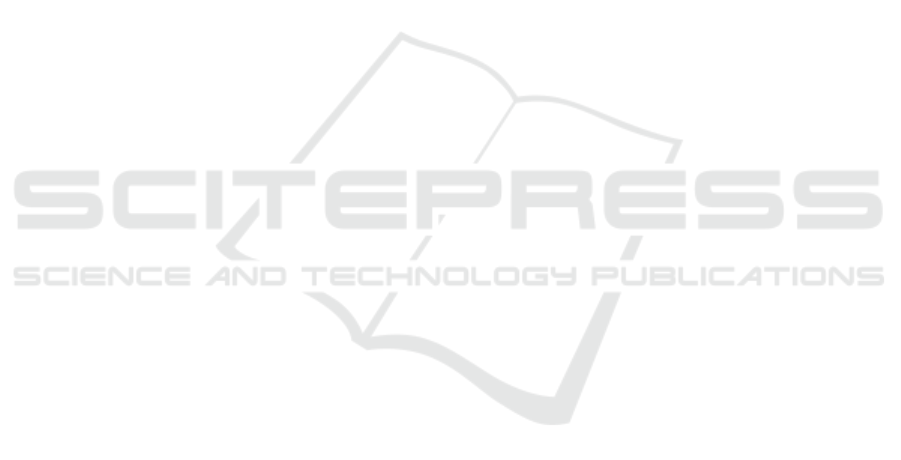
giving users with clear insights into energy data.
This workflow enables efficient data collection,
processing, and visualization, offering users a seam-
less and user-friendly experience for analyzing and
interpreting energy data.
5 CONCLUSION
This work focused on implementing and analyzing
smart components within a digital energy twin for
the Computer Scince and Mathematics faculty build-
ing at OTH Regensburg, aiming to support the de-
velopment of the digital twin and facilitate sustain-
able energy analysis and optimization possibilities.
The comprehensive investigation into the building’s
current energy consumption yielded valuable insights
into energy flows and highlighted areas for potential
improvement. While the analysis revealed intriguing
trends and statistics, many anomalies were only spec-
ulative, as several underlying factors remain unexam-
ined. The analysis covered various levels, though a
more in-depth exploration of specific aspects is still
required. Two main requirements emerged from the
analysis and were further explored:
Forecasting Future Energy Consumption: This
requirement aims to predict future energy usage to an-
ticipate peak loads and take preventive actions. The
SARIMA algorithm was selected for this task, prov-
ing the available data was suitable for forecasting.
While the model showed promising initial results,
the limited historical data suggests that more time is
needed to establish a robust predictive foundation.
Visualization of Forecast and Estimation
Results: The integration of forecast and estimation
visualizations into the Cesium platform’s frontend
was successfully implemented. Although the back-
end was tested locally, it requires further adaptation
for deployment in a live environment.
In conclusion, this work has significantly con-
tributed to the development of the digital energy twin,
providing practical insights to enhance energy effi-
ciency and supporting smart campus’s vision of be-
coming a model for sustainable practices. The project
has laid a strong foundation for future research and
delivered initial functionalities that can be expanded
and refined in subsequent initiatives.
ACKNOWLEDGEMENTS
This work was supported by the Regensburg Center of
Energy and Resources (RCER). Further information
under www.rcer.de
REFERENCES
Afram, A. and Janabi-Sharifi, F. (2014). Theory and appli-
cations of hvac control systems – a review of model
predictive control (mpc). Building and Environment,
72:343–355.
Alghamdi, A., Hu, G., Haider, H., Hewage, K., and Sadiq,
R. (2020). Benchmarking of water, energy, and car-
bon flows in academic buildings: A fuzzy clustering
approach. Sustainability, 12(11):4422.
Burnham, K. P. and Anderson, D. R. (2004). Multi-
model inference. Sociological Methods & Research,
33(2):261–304.
Danish, M. S. S., Senjyu, T., Ibrahimi, A. M., Ahmadi, M.,
and Howlader, A. M. (2019). A managed framework
for energy-efficient buildings. Journal of Building En-
gineering, 21:120–128.
Ding, J., Tarokh, V., and Yang, Y. (2018). Model selec-
tion techniques: An overview. IEEE Signal Process-
ing Magazine, 35(6):16–34.
do Amaral, J., dos Santos, C., Montevechi, J., and Queiroz,
A. (2023). Energy digital twin applications: A re-
view. Renewable and Sustainable Energy Reviews,
188:113891.
Farghali, M., Osman, A. I., Mohamed, I. M., Chen, Z.,
Chen, L., Ihara, I., Yap, P.-S., and Rooney, D. W.
(2023). Strategies to save energy in the context of the
energy crisis: a review. Environmental Chemistry Let-
ters, 21(4):1–37.
Farghali, M., Osman, A. I., Umetsu, K., and Rooney, D. W.
(2022). Integration of biogas systems into a carbon
zero and hydrogen economy: a review. Environmental
Chemistry Letters, 20(5):2853–2927.
Farsi, M., Daneshkhah, A., Hosseinian-Far, A., and Ja-
hankhani, H. (2023). Digital Twin Technologies and
Smart Cities.
Federal Ministry for Housing, U. D. and Construction
(2024). Act on heat planning and the decarbonisation
of heating networks. Accessed: 2024-11-11.
Hafez, F. S., Sa’di, B., Safa-Gamal, M., Taufiq-Yap, Y.,
Alrifaey, M., Seyedmahmoudian, M., Stojcevski, A.,
Horan, B., and Mekhilef, S. (2023). Energy effi-
ciency in sustainable buildings: A systematic review
with taxonomy, challenges, motivations, methodolog-
ical aspects, recommendations, and pathways for fu-
ture research. Energy Strategy Reviews, 45:101013.
Hirschle, J. (2021). Machine Learning f
¨
ur Zeitreihen: Ein-
stieg in Regressions-, ARIMA- und Deep-Learning-
Verfahren mit Python. Hanser.
International Energy Agency (2024). A 10-point plan to re-
duce the european union’s reliance on russian natural
gas – analysis. Version: 10.09.2024.
Invidiata, A., Lavagna, M., and Ghisi, E. (2018). Selecting
design strategies using multi-criteria decision making
to improve the sustainability of buildings. Building
and Environment, 139:58–68.
Khan, S. U., Khan, N., Ullah, F. U. M., Kim, M. J., Lee,
M. Y., and Baik, S. W. (2023). Towards intelligent
building energy management: Ai-based framework
Analysis and Design of Smart Components in Digital Energy Twins
271