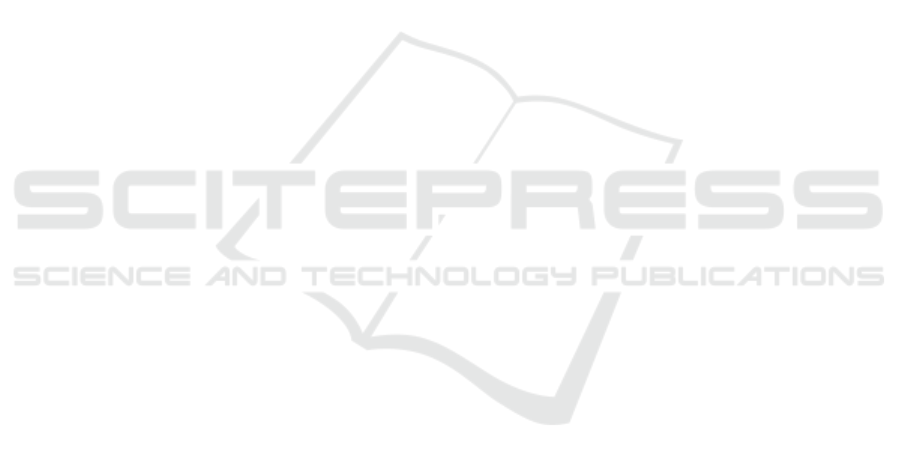
7 LESSONS LEARNED
During the development and testing of the Big Data
Fortaleza platform, several valuable lessons were
learned, significantly contributing to improving the
development process and quality assurance. Here are
some of the key lessons that emerged:
1. Invest in Testing Processes to Ensure Data
Quality. Data quality is a crucial aspect of the
success of a Big Data platform. During the sys-
tem testing, it became evident that investing time
and resources in ensuring data quality is neces-
sary. This includes identifying, cleaning, stan-
dardizing formats, and ensuring data integrity and
consistency, as poor data quality can compromise
the effectiveness of analysis and decision-making.
In general, it is observed that such activities are
the responsibility of the data team, and there is no
strong culture related to test coding.
2. Need for Data Simulation. An important lesson
learned during the development and validation of
the Big Data Fortaleza system was the need for
data simulation, in addition to using accurate data,
to effectively validate the dashboards present in
the system. This approach allowed a more com-
prehensive verification of the dashboard function-
alities, ensuring that they could handle a variety
of scenarios and data volumes.
3. Security Testing Should Be Conducted Contin-
uously from the Start of the Project. Data pro-
tection policies are crucial in Big Data projects,
especially those dealing with sensitive data. This
includes penetration testing, vulnerability analy-
sis, data encryption, and restricted access policies
to protect the integrity and confidentiality of the
information stored and processed by the platform.
4. Integration and Unit Tests Are Vital to Ensure
the Reliability of Business Rules. Since the sys-
tem deals with sensitive data, conducting integra-
tion tests is crucial. These tests are designed to
verify whether the different components of the
system interact correctly with each other and with
the database, ensuring the integrity and proper
functioning of the application as a whole.
5. Assertive Communication Between Teams
Should Be a Priority. Support and communica-
tion between developers and data scientists were
essential for the testing team to perform their work
effectively and contribute significantly to the suc-
cess of the Big Data Fortaleza project. Constant
communication with the development team al-
lowed for continuous information exchange about
bugs that should be prioritized, recurring failures,
and knowledge transfer. Data scientists, in turn,
helped the testing team better understand the data
analysis requirements and identify possible incon-
sistencies. This collaboration resulted in a more
comprehensive testing approach, ensuring early
detection of issues and delivering a high-quality
final product.
REFERENCES
Aydos, M., Aldan, C¸ ., Cos¸kun, E., and Soydan, A.
(2022). Security testing of web applications: A sys-
tematic mapping of the literature. Journal of King
Saud University-Computer and Information Sciences,
34(9):6775–6792.
Bourque, P. and Fairley, R. (2014). SWEBOK: Guide to the
software engineering body of knowledge. IEEE Com-
puter Society, Los Alamitos, CA, version 3.0 edition.
Costa, A., Freitas, L., Cavalcante, D., Oliveira, V., Lelli, V.,
Santos, I., Oliveira, P., Nogueira, T., and Andrade, R.
(2024). Especificac¸
˜
ao de requisitos em um projeto de
big data no setor p
´
ublico. In Anais do XXVII Con-
gresso Ibero-Americano em Engenharia de Software,
pages 417–420, Porto Alegre, RS, Brasil. SBC.
Daase, C., Staegemann, D., and Turowski, K. (2024). Over-
coming the complexity of quality assurance for big
data systems: An examination of testing methods. In
IoTBDS, pages 358–369, Magdeburg, Germany. Insti-
tute of Technical and Business Information Systems.
de Oliveira, I., Lima, J. M., Cristhian, S., Santos, I. S., and
Andrade, R. (2024). Quality of big data systems: a
systematic review of practices methods and tools. In
SBQS 2024 - Trilha de Trabalhos T
´
ecnicos.
Nasir, N., Imtiaz, S., Imtiaz, S., and Nabeel, M. (2022).
Testing framework for big data: A case study of tele-
com sector of pakistan.
OWASP (2023). Owasp testing guide.
Punn, N. S., Agarwal, S., Syafrullah, M., and Adiyarta, K.
(2019). Testing big data application. In 2019 6th Inter-
national Conference on Electrical Engineering, Com-
puter Science and Informatics (EECSI). IEEE.
Rivero, L., Diniz, J., Silva, G., Borralho, G., Braz, G.,
Paiva, A., Alves, E., and Oliveira, M. (2020). De-
ployment of a machine learning system for predicting
lawsuits against power companies: Lessons learned
from an agile testing experience for improving soft-
ware quality. In Anais do XIX Simp
´
osio Brasileiro de
Qualidade de Software, pages 294–303, Porto Alegre,
RS, Brasil. SBC.
Santos, I., Oliveira, P., Oliveira, V., Nogueira, T., Dan-
tas, A., Menescal, L.,
´
Elcio Batista, and Andrade, R.
(2023). Big data fortaleza: Plataforma inteligente para
pol
´
ıticas p
´
ublicas baseadas em evid
ˆ
encias. In Anais do
XI Workshop de Computac¸
˜
ao Aplicada em Governo
Eletr
ˆ
onico, pages 200–211, Porto Alegre, RS, Brasil.
SBC.
Schwaber, K. and Sutherland, J. (2020). The Scrum Guide
– the definitive guide to scrum: The rules of the game.
Big Data Fortaleza Platform: Quality Improvement with Testing Process
235