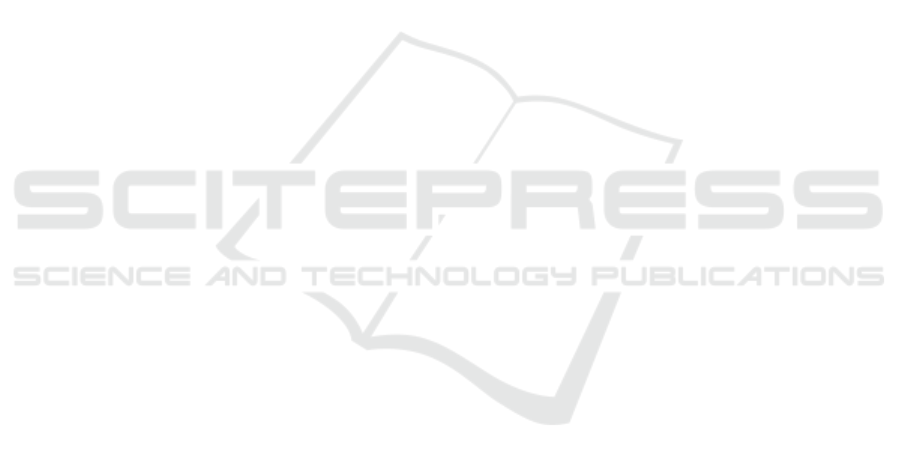
Ding, Q., Ding, D., Wang, Y., Guan, C., and Ding, B.
(2023). Unraveling the landscape of large language
models: a systematic review and future perspectives.
Journal of Electronic Business & Digital Economics.
Doewes, A., Kurdhi, N., and Saxena, A. (2023). Evaluat-
ing quadratic weighted kappa as the standard perfor-
mance metric for automated essay scoring. In Pro-
ceedings of the 16th International Conference on Ed-
ucational Data Mining, pages 103–113. International
Educational Data Mining Society (IEDMS).
Galhardi, L. and Brancher, J. (2018). Machine learning ap-
proach for automatic short answer grading: A system-
atic review. pages 380–391.
Godbole, V., Dahl, G. E., Gilmer, J., Shallue, C. J., and
Nado, Z. (2023). Deep learning tuning playbook. Ver-
sion 1.0.
Haller, S., Aldea, A., Seifert, C., and Strisciuglio, N. (2022).
Survey on automated short answer grading with deep
learning: from word embeddings to transformers.
Hu, E. J., Shen, Y., Wallis, P., Allen-Zhu, Z., Li, Y., Wang,
S., Wang, L., and Chen, W. (2021). Lora: Low-rank
adaptation of large language models.
Kortemeyer, G. (2023). Performance of the pre-trained
large language model gpt-4 on automated short an-
swer grading.
Kumar, Y., Aggarwal, S., Mahata, D., Shah, R. R., Ku-
maraguru, P., and Zimmermann, R. (2020). Get it
scored using autosas – an automated system for scor-
ing short answers.
Latif, E. and Zhai, X. (2024). Fine-tuning chatgpt for au-
tomatic scoring. Computers and Education: Artificial
Intelligence, 6:100210.
Loshchilov, I. and Hutter, F. (2019). Decoupled weight de-
cay regularization.
Mohler, M., Bunescu, R., and Mihalcea, R. (2011). Learn-
ing to grade short answer questions using seman-
tic similarity measures and dependency graph align-
ments. In Lin, D., Matsumoto, Y., and Mihalcea, R.,
editors, Proceedings of the 49th Annual Meeting of
the Association for Computational Linguistics: Hu-
man Language Technologies, pages 752–762, Port-
land, Oregon, USA. Association for Computational
Linguistics.
Page, E. B. (1966). The imminence of... grading essays by
computer. The Phi Delta Kappan, 47(5):238–243.
Reizinger, P., Ujv
´
ary, S., M
´
esz
´
aros, A., Kerekes, A., Bren-
del, W., and Husz
´
ar, F. (2024). Position: Understand-
ing llms requires more than statistical generalization.
In Forty-first International Conference on Machine
Learning.
Saha, S., Dhamecha, T. I., Marvaniya, S., Foltz, P., Sind-
hgatta, R., and Sengupta, B. (2019). Joint multi-
domain learning for automatic short answer grading.
arXiv preprint arXiv:1902.09183.
Shallue, C. J., Lee, J., Antognini, J. M., Sohl-Dickstein, J.,
Frostig, R., and Dahl, G. E. (2018). Measuring the
effects of data parallelism on neural network training.
CoRR, abs/1811.03600.
Sung, C., Dhamecha, T. I., and Mukhi, N. (2019). Improv-
ing short answer grading using transformer-based pre-
training. In Isotani, S., Mill
´
an, E., Ogan, A., Hast-
ings, P., McLaren, B., and Luckin, R., editors, Artifi-
cial Intelligence in Education, pages 469–481, Cham.
Springer International Publishing.
Taghipour, K. and Ng, H. T. (2016). A neural approach to
automated essay scoring. In Proceedings of the 2016
conference on empirical methods in natural language
processing, pages 1882–1891.
Tashu, T. M., Maurya, C. K., and Horvath, T. (2022). Deep
learning architecture for automatic essay scoring.
Tornqvist, M., Mahamud, M., Mendez Guzman, E., and
Farazouli, A. (2023). ExASAG: Explainable frame-
work for automatic short answer grading. In Kochmar,
E., Burstein, J., Horbach, A., Laarmann-Quante, R.,
Madnani, N., Tack, A., Yaneva, V., Yuan, Z., and
Zesch, T., editors, Proceedings of the 18th Workshop
on Innovative Use of NLP for Building Educational
Applications (BEA 2023), pages 361–371, Toronto,
Canada. Association for Computational Linguistics.
Vaswani, A., Shazeer, N., Parmar, N., Uszkoreit, J., Jones,
L., Gomez, A. N., Kaiser, L., and Polosukhin, I.
(2023). Attention is all you need.
Wei, J., Bosma, M., Zhao, V. Y., Guu, K., Yu, A. W., Lester,
B., Du, N., Dai, A. M., and Le, Q. V. (2021). Fine-
tuned language models are zero-shot learners. arXiv
preprint arXiv:2109.01652.
Zhang, A., Lipton, Z. C., Li, M., and Smola, A. J. (2023a).
Dive into Deep Learning, pages 551–552. Cambridge
University Press. https://D2L.ai.
Zhang, S., Dong, L., Li, X., Zhang, S., Sun, X., Wang, S.,
Li, J., Hu, R., Zhang, T., Wu, F., and Wang, G. (2024).
Instruction tuning for large language models: A sur-
vey.
Zhang, Y., Cui, L., Cai, D., Huang, X., Fang, T., and Bi,
W. (2023b). Multi-task instruction tuning of llama for
specific scenarios: A preliminary study on writing as-
sistance. arXiv preprint arXiv:2305.13225.
Zhao, Z., Fan, W., Li, J., Liu, Y., Mei, X., Wang, Y., Wen,
Z., Wang, F., Zhao, X., Tang, J., et al. (2023). Recom-
mender systems in the era of large language models
(llms). arXiv preprint arXiv:2307.02046.
Evaluating the Potential of LLMs for Better Short Answer Scoring
119