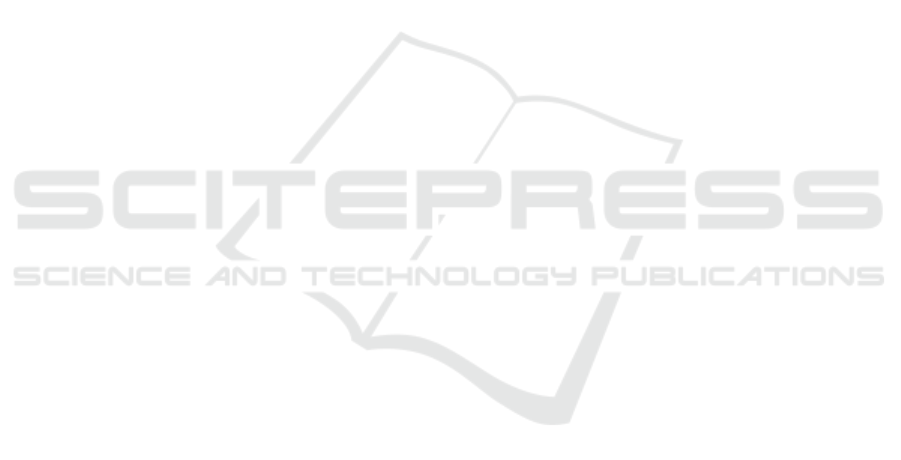
Deng, J., Dong, W., Socher, R., Li, L.-J., Li, K., and Fei-
Fei, L. (2009). Imagenet: A large-scale hierarchical
image database. In 2009 IEEE conference on com-
puter vision and pattern recognition, pages 248–255.
Ieee.
Dosovitskiy, A. (2020). An image is worth 16x16 words:
Transformers for image recognition at scale. arXiv
preprint arXiv:2010.11929.
Fan, X., Zhou, J., Jiang, X., Xin, M., and Hou, L. (2024).
Csap-unet: Convolution and self-attention paralleling
network for medical image segmentation with edge
enhancement. Computers in Biology and Medicine,
172:108265.
He, K., Gan, C., Li, Z., Rekik, I., Yin, Z., Ji, W., Gao, Y.,
Wang, Q., Zhang, J., and Shen, D. (2023). Transform-
ers in medical image analysis. Intelligent Medicine,
3(1):59–78.
He, K., Zhang, X., Ren, S., and Sun, J. (2016). Deep resid-
ual learning for image recognition. In Proceedings of
the IEEE conference on computer vision and pattern
recognition, pages 770–778.
Isensee, F., Petersen, J., Klein, A., Zimmerer, D., Jaeger,
P. F., Kohl, S., Wasserthal, J., Koehler, G., Norajitra,
T., Wirkert, S., et al. (2018). nnu-net: Self-adapting
framework for u-net-based medical image segmenta-
tion. arXiv preprint arXiv:1809.10486.
Ju, J., Li, J., Chang, Z., Liang, Y., Guan, Z., Xu, P., and
Xie, F. (2023). Incorporating multi-stage spatial vi-
sual cues and active localization offset for pancreas
segmentation. Pattern Recognition Letters.
Kleeff, J., Korc, M., Apte, M., La Vecchia, C., Johnson,
C. D., Biankin, A. V., Neale, R. E., Tempero, M., Tu-
veson, D. A., Hruban, R. H., et al. (2016). Pancreatic
cancer. Nature reviews Disease primers, 2(1):1–22.
Li, Q., Liu, X., He, Y., Li, D., and Xue, J. (2023). Temper-
ature guided network for 3d joint segmentation of the
pancreas and tumors. Neural Networks.
Li, Z., Pan, H., Zhu, Y., and Qin, A. (2020). Pgd-unet: A
position-guided deformable network for simultaneous
segmentation of organs and tumors. In 2020 Interna-
tional Joint Conference on Neural Networks (IJCNN).
IEEE.
Liu, S., Yuan, X., Hu, R., Liang, S., Feng, S., Ai, Y., and
Zhang, Y. (2019). Automatic pancreas segmentation
via coarse location and ensemble learning. IEEE Ac-
cess, 8:2906–2914.
Mahmoudi, T., Kouzahkanan, Z., and Radmard, A. (2022).
Segmentation of pancreatic ductal adenocarcinoma
(pdac) and surrounding vessels in ct images using
deep convolutional neural networks and texture de-
scriptors. Scientific Reports.
Mo, J., Zhang, L., Wang, Y., and Huang, H. (2020). It-
erative 3d feature enhancement network for pancreas
segmentation from ct images. Neural Computing and
Applications, 32:12535–12546.
Moradi, N., Doshantapeh, A. G., Sangi, S., Aligholizadeh,
M., Asadian, A., Abdolmohammadi, G., Ghare-
bakhshi, F., Abdolmohammadi, G., and Molaee, H.
(2022). 4. an ecological study of the incidence and
mortality rates of pancreatic cancer in 2020: explor-
ing gender disparities worldwide. Journal of renal en-
docrinology.
Petersen, G. M. (2016). Familial pancreatic cancer. In Sem-
inars in oncology, volume 43, pages 548–553. Else-
vier.
Ronneberger, O., Fischer, P., and Brox, T. (2015). U-
net: Convolutional networks for biomedical image
segmentation. In Medical image computing and
computer-assisted intervention–MICCAI 2015: 18th
international conference, Munich, Germany, October
5-9, 2015, proceedings, part III 18, pages 234–241.
Springer.
Seufferlein, T. and Kestler, A. M. R. (2023). 1. [exocrine
pancreatic cancer - what is new in the update of the s3
guideline?]. Deutsche Medizinische Wochenschrift.
Stoffel, E. M., Brand, R. E., and Goggins, M. (2023). Pan-
creatic cancer: Changing epidemiology and new ap-
proaches to risk assessment, early detection, and pre-
vention. Gastroenterology, 164(5):752–765. Person-
alizing GI Cancer Risk Assessment and Management:
The Future is Now.
Su, Y., Liu, Q., Xie, W., and Hu, P. (2022). Yolo-
logo: A transformer-based yolo segmentation model
for breast mass detection and segmentation in digital
mammograms. Computer Methods and Programs in
Biomedicine, 221:106903.
Taha, A. A. and Hanbury, A. (2015). Metrics for evaluating
3d medical image segmentation: analysis, selection,
and tool. BMC Medical Imaging, 15(1):29.
Ture
ˇ
cková, A., Ture
ˇ
cek, T., et al. (2020). Improving ct im-
age tumor segmentation through deep supervision and
attentional gates. Frontiers in Robotics and AI.
Vaswani, A. (2017). Attention is all you need. arXiv
preprint arXiv:1706.03762.
Wang, T., Lan, J., Han, Z., Hu, Z., Huang, Y., Deng, Y.,
Zhang, H., Wang, J., Chen, M., Jiang, H., et al. (2022).
O-net: a novel framework with deep fusion of cnn and
transformer for simultaneous segmentation and classi-
fication. Frontiers in neuroscience, 16:876065.
Yang, X., Chen, Y., Yue, X., Ma, C., and Yang, P. (2021).
Local linear embedding based interpolation neural
network in pancreatic tumor segmentation. Applied
Intelligence.
Zhao, Z. and Liu, W. (2020). Pancreatic cancer: a review of
risk factors, diagnosis, and treatment. Technology in
cancer research & treatment, 19:1533033820962117.
Zhou, Q. and Melton, D. A. (2018). Pancreas regeneration.
Nature, 557(7705):351–358.
Zhu, Z., Liu, C., Yang, D., Yuille, A., et al. (2019). V-nas:
Neural architecture search for volumetric medical im-
age segmentation. In 2019 International Conference
on Computer Vision (ICCV). IEEE.
ICEIS 2025 - 27th International Conference on Enterprise Information Systems
522