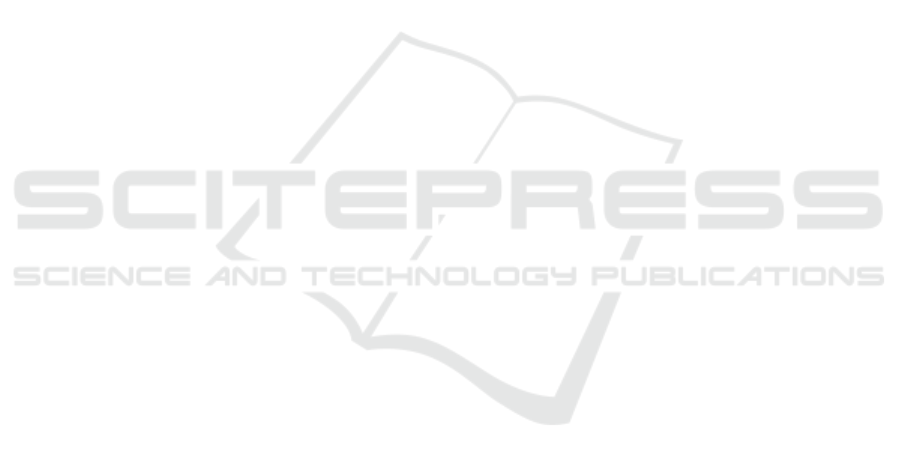
a higher coverage than many municipalities in Brazil
and worldwide, the coverage was only 62.1%, below
the 70% suggested by (Ramos et al., 2018). This work
also shows that more than half of the equipment in the
Northeast region is installed in the capitals. Thus, the
poor distribution of equipment is one of the reasons
the coverage does not reach the desired percentage.
This fact is also demonstrated by (Corrêa et al., 2011),
as the authors state that the poor distribution of mam-
mography units directly impacts the low production
of the equipment. In addition to inadequate distribu-
tion, (Corrêa et al., 2011) point out that, for women
who need to travel, the distance traveled is one of
the factors influencing their decision to undergo the
exam. The authors also state that, in 2015, the Min-
istry of Health established a maximum distance of 60
km to be traveled to perform the exam.
The Mammography Unit Location-Allocation
Problem (MULAP) is proposed in light of these is-
sues, such as the inefficient distribution of the mam-
mography units, the significant impact of women’s
travel on coverage rates, and the importance of this
exam for successful disease treatment. The MULAP
objective is to improve the location of mammography
units and, thus, increase the coverage rate of mam-
mography exams. (Souza et al., 2019) used two math-
ematical formulations to solve MULAP. In one, full
demand fulfillment for municipalities is considered,
while the second formulation allows for partial de-
mand fulfillment. In the conducted experiments, sce-
narios were considered where the existing mammog-
raphy units were kept in their current locations and
scenarios where there was the possibility of relocat-
ing those equipment. The results showed that relo-
cating the equipment would result in greater coverage
than that presented by the State Health Department of
Rondônia, Brazil. (Sá et al., 2019), using the formu-
lation of (Souza et al., 2019), addressed MULAP in
an instance of the State of Espírito Santo, considering
two scenarios: one keeping the existing location and
the other allowing the relocation of the mammogra-
phy units. The authors showed that the existing loca-
tion met half of the state’s demand, using 63.1% of
the capacity of the mammography units, while relo-
cating the equipment would allow for 83.5% demand
coverage, increasing the equipment usage to 99.9%.
(Souza et al., 2020) used the same model and devel-
oped a Variable Neighborhood Search (VNS) algo-
rithm to solve an instance based on data from the State
of Minas Gerais. In this case, the results obtained,
both from the mathematical formulation and the pro-
posed algorithm, showed superior coverage compared
to the existing location of the mammography units.
(de Campos et al., 2020) addressed MULAP using a
Simulated Annealing algorithm. The algorithm was
applied to instances from the state of Minas Gerais,
considering partial demand fulfillment. In this way,
a municipality is covered even if its demand is not
fully met. Additionally, that work considered the ac-
quisition of new equipment. The results indicated
that, even under these conditions, not all municipal-
ities had their demands fully met. Other studies in the
literature, such as (de Assis et al., 2022), (de Cam-
pos et al., 2022), and (de Campos et al., 2024), also
address MULAP. In all these works, full demand cov-
erage is not achieved due to factors such as the max-
imum distance constraint between the demand point
and the equipment’s host municipality and/or the ab-
sence of hospital infrastructure.
(Jewett et al., 2018) showed that the demand for
the screenings is inversely proportional to the dis-
tance traveled to perform it. In this sense, (De Mil
et al., 2019) suggested using Mobile Mammography
Units (MMU) to serve women living in remote areas
where the installation of a fixed unit is unfeasible.
(Rosa et al., 2020) proposed a constructive heuristic
algorithm to address the Mobile Mammography Unit
Routing Problem (MMURP). In (Rosa et al., 2020),
MMUs were routed to serve 444 locations, aiming to
maximize the coverage of the demand and minimize
the total distance traveled. In (Rosa et al., 2021), the
authors presented an Iterated Greedy Search (IGS) al-
gorithm to route 56 MMUs, departing from two de-
pots, to serve 579 municipalities.
The aforementioned studies addressed MULAP
and MMURP separately. In the present work, the ob-
jective is to solve them through a sequential proce-
dure. Initially, it is proposed to generate a solution
for MULAP and then, based on the municipalities not
served or partially served in this solution, generate a
solution via MMURP. To solve these two problems,
the formulation by (Souza et al., 2019) will be ap-
plied for the solution of MULAP, and based on the re-
sults obtained, a constructive heuristic algorithm will
be applied to route the MMUs to meet the remaining
demand from MULAP. This solution procedure is ap-
plied to a case study addressing three scenarios in the
State of Minas Gerais. The first scenario considers the
freedom to relocate fixed mammography equipment.
The second scenario considers the current location of
fixed equipment and does not restrict the service of
municipalities to their health micro-regions. Finally,
the third scenario considers the current location of
fixed equipment and restricts the service of munici-
palities to their health micro-regions.
The remainder of this work is organized as fol-
lows. Section 2 describes the problems addressed.
Section 3 presents the application scenarios for MU-
ICEIS 2025 - 27th International Conference on Enterprise Information Systems
524