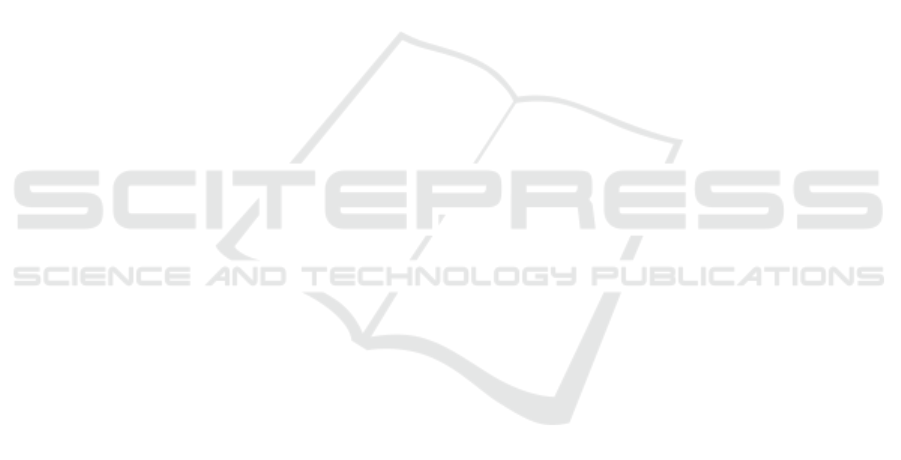
ACL 2024, pages 12186–12215, Bangkok, Thailand.
Association for Computational Linguistics.
Khan, M. P. and O’Sullivan, E. D. (2024). A comparison
of the diagnostic ability of large language models in
challenging clinical cases. Frontiers in Artificial In-
telligence, 7:1379297.
Kocbek, P. et al. (2022). Generating Extremely Short Sum-
maries from the Scientific Literature to Support De-
cisions in Primary Healthcare: A Human Evaluation
Study. In Michalowski, M., Abidi, S. S. R., and Abidi,
S., editors, Artificial Intelligence in Medicine, pages
373–382, Cham. Springer International Publishing.
Koutsouleris, N. et al. (2022). From promise to practice:
towards the realisation of AI-informed mental health
care. The Lancet Digital Health, 4(11):e829–e840.
Publisher: Elsevier.
Krippendorff, K. (2018). Content Analysis: An Introduc-
tion to Its Methodology. SAGE Publications. Google-
Books-ID: nE1aDwAAQBAJ.
Lai, T. et al. (2024). Supporting the Demand on Men-
tal Health Services with AI-Based Conversational
Large Language Models (LLMs). BioMedInformat-
ics, 4(1):8–33. Number: 1 Publisher: Multidisci-
plinary Digital Publishing Institute.
Lawrence, H. R. et al. (2024). The Opportunities and Risks
of Large Language Models in Mental Health. JMIR
Mental Health, 11(1):e59479. Company: JMIR Men-
tal Health Distributor: JMIR Mental Health Institu-
tion: JMIR Mental Health Label: JMIR Mental Health
Publisher: JMIR Publications Inc., Toronto, Canada.
Li, A. et al. (2024). Understanding the Therapeutic Re-
lationship between Counselors and Clients in On-
line Text-based Counseling using LLMs. In Al-
Onaizan, Y., Bansal, M., and Chen, Y.-N., editors,
Findings of the Association for Computational Lin-
guistics: EMNLP 2024, pages 1280–1303, Miami,
Florida, USA. Association for Computational Lin-
guistics.
Lin, C.-Y. (2004). ROUGE: A Package for Automatic
Evaluation of Summaries. In Text Summarization
Branches Out, pages 74–81, Barcelona, Spain. Asso-
ciation for Computational Linguistics.
Liu, J. M. et al. (2023). ChatCounselor: A Large
Language Models for Mental Health Support.
arXiv:2309.15461.
Mirzakhmedova, N. et al. (2024). Are Large Language
Models Reliable Argument Quality Annotators? In
Cimiano, P. et al., editors, Robust Argumentation
Machines, pages 129–146, Cham. Springer Nature
Switzerland.
Papineni, K. et al. (2002). Bleu: a Method for Automatic
Evaluation of Machine Translation. In Isabelle, P.,
Charniak, E., and Lin, D., editors, Proceedings of
the 40th Annual Meeting of the Association for Com-
putational Linguistics, pages 311–318, Philadelphia,
Pennsylvania, USA. Association for Computational
Linguistics.
Rao, A., Aithal, S., and Singh, S. (2024). Single-Document
Abstractive Text Summarization: A Systematic Liter-
ature Review. ACM Comput. Surv., 57(3):60:1–60:37.
Rudolph, E., Engert, N., and Albrecht, J. (2024). An AI-
Based Virtual Client for Educational Role-Playing in
the Training of Online Counselors:. In Proceedings of
the 16th International Conference on Computer Sup-
ported Education, pages 108–117, Angers, France.
SCITEPRESS - Science and Technology Publications.
Shahriar, S. et al. (2024). Putting GPT-4o to the Sword:
A Comprehensive Evaluation of Language, Vision,
Speech, and Multimodal Proficiency. Applied Sci-
ences, 14(17):7782. Number: 17 Publisher: Multi-
disciplinary Digital Publishing Institute.
Singh, M. et al. (2023). CodeFusion: A Pre-trained Diffu-
sion Model for Code Generation. arXiv:2310.17680
version: 3.
Tam, T. Y. C. et al. (2024). A framework for human eval-
uation of large language models in healthcare derived
from literature review. npj Digital Medicine, 7(1):1–
20. Publisher: Nature Publishing Group.
Temsah, M.-H. et al. (2024). OpenAI o1-Preview vs. Chat-
GPT in Healthcare: A New Frontier in Medical AI
Reasoning. Cureus, 16(10):e70640.
Vowels, L. M., Francois-Walcott, R. R. R., and Darwiche,
J. (2024). AI in relationship counselling: Evaluating
ChatGPT’s therapeutic capabilities in providing rela-
tionship advice. Computers in Human Behavior: Ar-
tificial Humans, 2(2):100078.
Wu, Y. et al. (2024). Less is More for Long Document Sum-
mary Evaluation by LLMs. In Graham, Y. and Purver,
M., editors, Proceedings of the 18th Conference of
the European Chapter of the Association for Compu-
tational Linguistics (Volume 2: Short Papers), pages
330–343, St. Julian’s, Malta. Association for Compu-
tational Linguistics.
Xu, X. et al. (2024). Mental-LLM: Leveraging Large Lan-
guage Models for Mental Health Prediction via Online
Text Data. Proc. ACM Interact. Mob. Wearable Ubiq-
uitous Technol., 8(1):31:1–31:32.
Yin, Y.-J., Chen, B.-Y., and Chen, B. (2024). A Novel LLM-
based Two-stage Summarization Approach for Long
Dialogues. arXiv:2410.06520.
Zhang, H., Yu, P. S., and Zhang, J. (2024a). A
Systematic Survey of Text Summarization: From
Statistical Methods to Large Language Models.
arXiv:2406.11289.
Zhang, R. and Tetreault, J. (2019). This Email Could
Save Your Life: Introducing the Task of Email Sub-
ject Line Generation. In Korhonen, A., Traum, D.,
and M
`
arquez, L., editors, Proceedings of the 57th An-
nual Meeting of the Association for Computational
Linguistics, pages 446–456, Florence, Italy. Associ-
ation for Computational Linguistics.
Zhang, T. et al. (2019). BERTScore: Evaluating Text Gen-
eration with BERT. In International Conference on
Learning Representations.
Zhang, T. et al. (2024b). Benchmarking Large Language
Models for News Summarization. Transactions of the
Association for Computational Linguistics, 12:39–57.
Comparing Large Language Models for Automated Subject Line Generation in e-Mental Health: A Performance Study
77