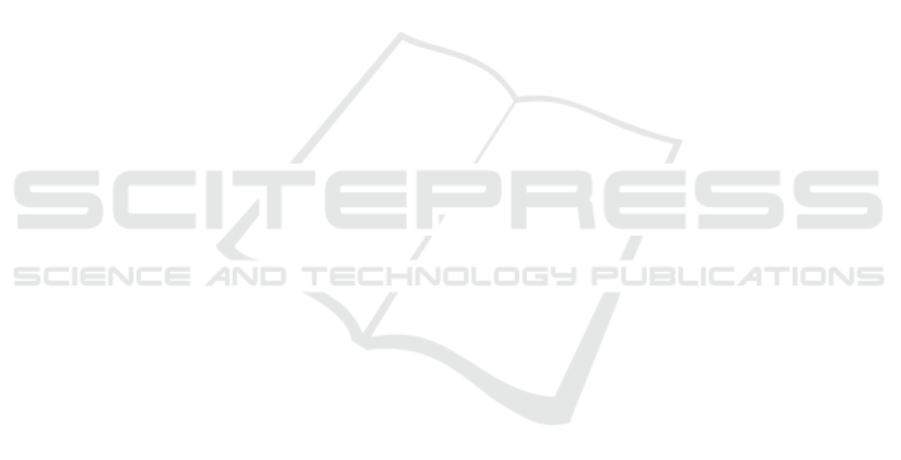
Despite the potential of Big Data in government
decision-making, several challenges hinder its practi-
cal implementation. The heterogeneity and inconsis-
tency of data from diverse sources, lack of standard-
ization in collection methods, and the need for scal-
able, automated data pipelines increase operational
costs and compromise analysis quality. This work
proposes a robust framework integrating automation,
preprocessing, validation techniques, and standard-
ization practices to improve the quality and usability
of both textual and geographic data.
The remainder of this paper is structured as fol-
lows: Section 2 reviews related works, discussing
methodologies relevant to data processing challenges.
Section 3 presents the foundational concepts of Data
Science and the key stages of the project’s imple-
mentation. Section 4 details the cloud computing ar-
chitecture, emphasizing scalability, security, and op-
erational advantages. Section 5 describes the func-
tional and non-functional requirements necessary for
efficient Big Data management in government sys-
tems. Section 6 explores the main challenges encoun-
tered and the solutions implemented. Section 7 eval-
uates the system’s applicability, discusses technical
and operational constraints, and presents case stud-
ies demonstrating its impact. Finally, Section 8 con-
cludes with a summary of findings and future research
directions.
2 RELATED WORK
This section presents works that incorporate various
Data Science and Big Data methods, focusing on their
application to decision-making and the development
of new applications. Sarker (2021) discusses the rel-
evance of advanced data analysis methods across dif-
ferent sectors, emphasizing their impact on decision-
making, operational optimization, and trend forecast-
ing. While it highlights the importance of customized
applications in healthcare, smart cities, and cyber-
security, it does not specifically address government
data standardization and harmonization.
Freitas et al. (2023) propose a data warehousing
environment for crime data analysis, supporting pub-
lic security managers in strategic decision-making.
The study identifies key challenges, such as data het-
erogeneity, lack of standardization, and the need for
advanced extraction, transformation, and visualiza-
tion techniques.
Fugini and Finocchi (2020) focus on documental
Big Data processing, introducing an Enterprise Con-
tent Management (ECM) system enhanced with ma-
chine learning for classification and information ex-
traction. Their work defines quality metrics—Textual
Quality Confidence, Classification Confidence, and
Extraction Confidence—to assess system accuracy
and efficiency. These indicators contribute to data in-
tegrity and consistency but do not fully address het-
erogeneous governmental data integration.
Behringer et al. (2023) present SDRank, a deep
learning-based approach for ranking data sources by
similarity, optimizing semantic pattern recognition
and automated data selection. While this technique
improves efficiency and scalability in large-scale data
processing, it does not tackle structural inconsisten-
cies in government datasets.
Furtado et al. (2023) investigate digital transfor-
mation in smart governance, analyzing how Big Data
tools can support policies aimed at vulnerable pop-
ulations. However, their study does not explore the
technical challenges of integrating and standardizing
multiple governmental data sources.
These studies provide valuable insights into Big
Data applications, yet they lack a detailed ar-
chitectural perspective on handling heterogeneous
and inconsistent government data. This work ad-
dresses these gaps by proposing a scalable integration
pipeline, combining automation, entity name match-
ing, and address standardization to enhance data qual-
ity and usability in public sector applications.
3 DATA SCIENCE, BIG DATA AND
PROJECT STAGES
Big Data refers to large, heterogeneous datasets that
exceed the processing capabilities of conventional
methods due to their dynamic and complex nature.
These datasets exhibit characteristics such as vol-
ume, velocity, variety, veracity, variability, and value,
requiring specialized techniques for their manage-
ment. In this study, Big Data encompasses diverse
governmental datasets, used to build analytical tools
that support municipal decision-making. Given these
characteristics, a key challenge is ensuring data stor-
age, cataloging, and availability for decision-making
processes.
Data Science, an interdisciplinary field integrat-
ing statistics, mathematics, and computer science, en-
ables the extraction of valuable insights to support
data-driven decisions (Wu et al., 2021). Identify-
ing patterns, trends, and hidden relationships within
data is complex but essential for predictions, process
optimization, and strategic decision-making (Sarker,
2021). Transforming raw data into actionable knowl-
edge involves several critical stages, as illustrated in
Figure 1. The data acquisition stage involves col-
Handling Inconsistent Government Data: From Acquisition to Entity Name Matching and Address Standardization
287