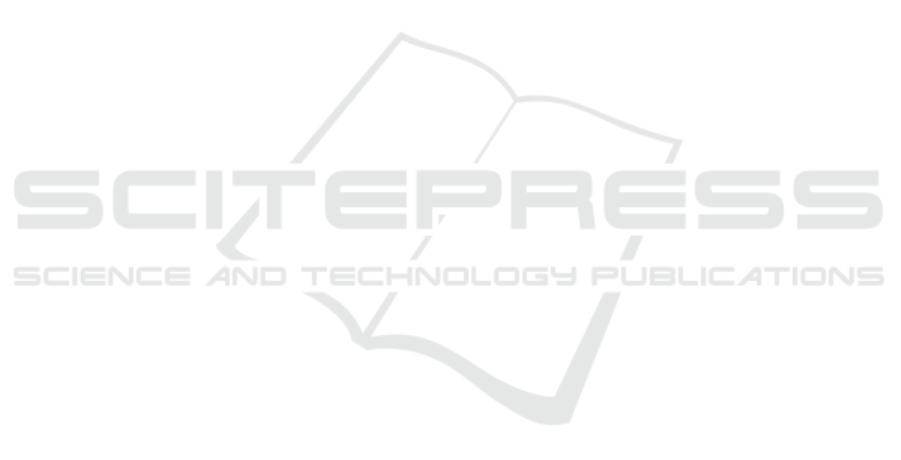
1000. These results indicate that both methods are
promising for selecting serverless providers in scenar-
ios where the criteria are known a priori.
However, further research is needed to assess the
robustness of these methods in more complex scenar-
ios with greater data variability.In this sense, future
work proposes increasing the number of test cases,
thereby generating even greater confidence in hypo-
thetical scenarios with more than 5 providers, adding
more PIs, including qualitative ones, thus having
more features that can add to the end user’s choice, as
well as making comparisons between other MCDMs
to obtain greater reliability in the results obtained.
ACKNOWLEDGMENTS
This work received financial support from the Coordi-
nation for the Improvement of Higher Education Per-
sonnel - CAPES - Brazil (PROAP/AUXPE).
REFERENCES
Akao, Y. (2024). Quality Function Deployment: Integrating
Customer Requirements into Product Design. Produc-
tivity Press, New York.
Baid, D., Goel, P. M., Bhardwaj, P., Singh, A., and Tyagi,
V. (2021). 2. comparative analysis of serverless so-
lutions from public cloud providers. In Information,
Communication and Computing Technology. 2021.
Boucher, T. O., Gogus, O., and Wicks, E. M. (1997).
A comparison between two multiattribute decision
methodologies used in capital investment decision
analysis. The Engineering Economist, 42(3):179–202.
Calderoni, L., Maio, D., and Tullini, L. (2022). Bench-
marking cloud providers on serverless iot back-end
infrastructures. IEEE Internet of Things Journal,
9(16):15255–15269.
Castro, P., Ishakian, V., Muthusamy, V., and Slominski, A.
(2019). The rise of serverless computing. Communi-
cations of the ACM, 62(12):44–54.
Dey, N. S., Reddy, S. P. K., and G, L. (2023). Serverless
computing: Architectural paradigms, challenges, and
future directions in cloud technology. In 2023 7th
International Conference on I-SMAC (IoT in Social,
Mobile, Analytics and Cloud) (I-SMAC), pages 406–
414.
Ishizaka, A. and Nemery, P. (2013). Multi-criteria decision
analysis: methods and software. Wiley, Chichester,
West Sussex, United Kingdom.
Jain, R. (1991). The art of computer systems performance
analysis: techniques for experimental design, mea-
surement, simulation, and modeling. Wiley, New
York.
Jayapriya, J., Selvakumari, K., and Kavitha, S. (2023). 2. on
solving multi-attribute decision making problem using
ahp. E3S web of conferences.
Khanal, D. D. and Maharjan, S. (2024). 1. comparative
security and compliance analysis of serverless com-
puting platforms: Aws lambda, azure functions, and
google cloud functions. Indian Scientific Journal Of
Research In Engineering And Management.
Krishnamurthi, R., Kumar, A., Gill, S. S., and Buyya,
R., editors (2023). Serverless Computing: Princi-
ples and Paradigms, volume 162 of Lecture Notes on
Data Engineering and Communications Technologies.
Springer International Publishing, Cham.
Liberatore, M. J. and Nydick, R. L. (1997). Group decision
making in higher education using the analytic hierar-
chy process. Research in Higher Education, 38:593–
614.
Muzdalifah, L. and Nursiyami, S. (2024). 1. ahp-topsis:
Multi-criteria decision making method with hierarchi-
cal weighting to determine priorities for leak work
drinking water pipe. Mathline.
Norris, G. A. and Marshall, H. E. (1995). Multiat-
tribute decision analysis method for evaluating build-
ings and building systems. Technical Report NIST IR
5663, National Institute of Standards and Technology,
Gaithersburg, MD, USA.
Puyt, R. W., Lie, F. B., and Wilderom, C. P. M. (2023).
The origins of SWOT analysis. Long Range Planning,
56(3).
Rifai, M. (2023). Serverless showdown: Aws lambda
vs azure functions vs google cloud functions.
https://www.pluralsight.com/resources/blog/cloud/
serverless-showdown-aws-lambda-vs-azure-
functions-vs-google-cloud-functions. Accessed:
2024-12-08.
Saaty, T. L. (1990). How to make a decision: The analytic
hierarchy process. European Journal of Operational
Research, 48:9–26.
Saaty, T. L. (2004). Decision making – the analytic hier-
archy and network processes (ahp/anp). Journal of
Systems Science and Systems Engineering, 13:1–35.
Salomon, V. P., MONTEVECHI, J. A., and Pamplona, E. O.
(1999). Justificativas para aplicação do método de
análise hierárquica. Encontro Nacional de Engen-
haria de Produção, 19.
Sonntag, S., Pohl, E., Luttmer, J., Geldermann, J., and Na-
garajah, A. (2024). A conceptual mcda-based frame-
work for machine learning algorithm selection in the
early phase of product development. Proceedings of
the Design Society.
Wen, J., Chen, Z., Jin, X., and Liu, X. (2023). Rise of
the planet of serverless computing: A systematic re-
view. ACM Transactions on Software Engineering and
Methodology, 32(5):1–61.
Wi˛eckowski, J., Kizielewicz, B., Shekhvovtsov, A., and
Sałabun, W. (2023). Towards sustainable cloud ser-
vices: Mcda approach.
ICEIS 2025 - 27th International Conference on Enterprise Information Systems
36