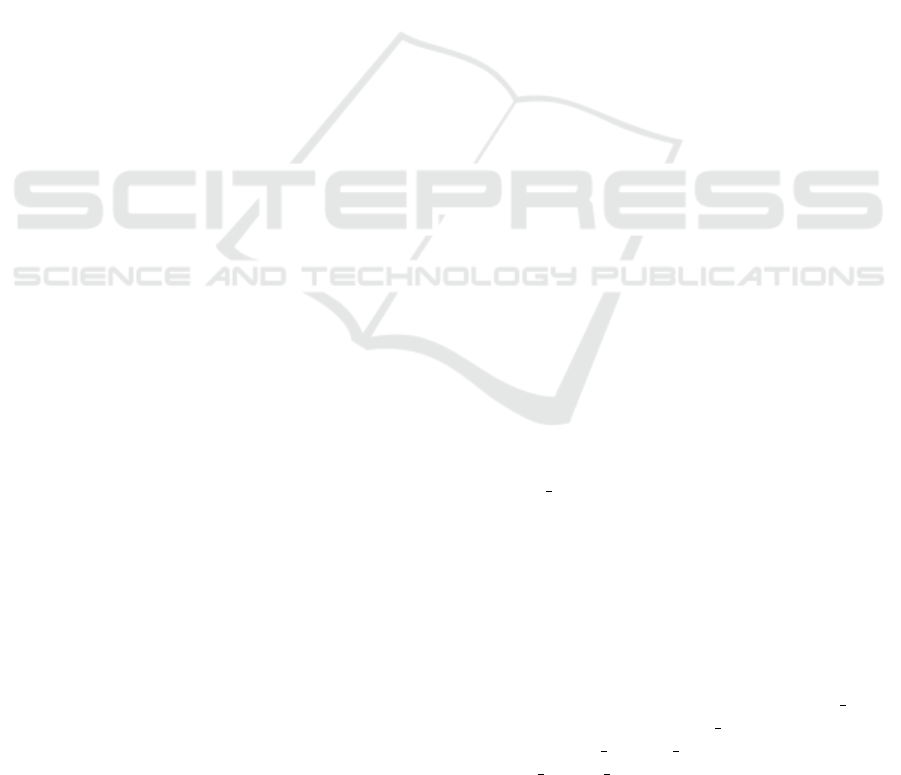
posed outcomes and discusses their possible applica-
tion, showcasing it in the design of a decision tree.
Section 8 concludes the paper and discusses the di-
rection for future work.
2 RELATED WORK
Automated model selection is a topic in ML re-
search that has attracted much interest.In 1994, Yumi
Iwasaki and Alon Y. Levy proposed an algorithm
for selecting model fragments automatically for sim-
ulation (Iwasaki and Levy, 1994). They designed
the algorithm based on relevance reasoning, which is
used to determine which phenomenon can affect the
query (Iwasaki and Levy, 1994). In 2010, Vincent
Calcagno designed and implemented an R package
glmulti to select generalized linear models automat-
ically (Calcagno and de Mazancourt, 2010). In 2016,
Gustavo Malkomes et al. employed Bayesian opti-
mization for automated model selection (Malkomes
et al., 2016). They constructed a novel kernel be-
tween models to explain a given dataset (Malkomes
et al., 2016). Lars Kotthoff et al. released the source
of Auto-WEKA, the addition of automatic selection
technology to the original platform (Kotthoff et al.,
2017). They used the Bayesian optimization method
to help users identify the best approach for their par-
ticular datasets. In recent years, Abdelhak Bentaleb
et al. proposed a kind of Automated Model selection
technique, which is used for predicting network band-
width (Bentaleb et al., 2020).
The automated model selection technique is also
a valuable topic in the time-series data area. In
2020, Yuanxiangyin et al. presented an automated
model selection framework to find the most suitable
model for time series anomaly detection by invoking
a pre-trained model selector and a parameter estima-
tor (Ying et al., 2020). In 2022, Chunnan Wang et
al. proposed an algorithm, AutoTS, which is used
for designing a suitable forecasting model for the
given time-series dataset. They constructed a search
space at first, then employed a two-stage pruning
and a knowledge graph analysis method (Wang et al.,
2022). In 2023, Shehan Saleem and Sapna Kumara-
pathirage created a framework for automated model
selection in natural language processing (Saleem and
Kumarapathirage, 2023). They conducted trials on
two models (BOWRF and FastText) to select the best-
performing models and evaluated the performance by
F1 macro and time (Saleem and Kumarapathirage,
2023). Amazon Web Services released AutoGluton-
TimeSeries, which is a part of AutoGluton framework
(Shchur et al., 2023). It combines classic statistic and
deep learning models with an ensembling technique
and helps users achieve time-series forecasting more
efficiently and simply.
Although several automated model selection tech-
niques have been proposed, most target various areas,
not specifically time-series data. Furthermore, those
techniques that focus on time-series data do not con-
sider the characteristics of time-series datasets, and
they only train the models without this information.
In this work, we fill this gap by conducting experi-
ments and acquiring several outcomes that can be ap-
plied to time-series data forecasting.
3 METHODOLOGY OF
EXPERIMENTS
To extract valuable outcomes for designing an auto-
mated model-selection technique for time-series fore-
casting, we devised a four-step methodology: review
of models, collection and review of datasets, selec-
tion of models and datasets, conduct experiments and
analyze results.
In the following, we give details about the first
three steps of our methodology. The rest of the steps
are summarized in the following sections.
• Review of Models. Various models can be used
for time-series data forecasting. The Autore-
gressive and Moving Average (ARMA) model
is a meaningful way to study time series (Mon-
dal et al., 2014). Based on this, the ARIMA,
one of the most popular algorithms in time-series
data prediction, was proposed (Box and Tiao,
1975).Besides, DL models are available for time-
series data forecasting as well, such as LSTM,
GRU, and Convolutional Neural Network (CNN).
There are also some variations of these models,
like Bidirectional LSTM, Bidirectional GRU, and
CNN LSTM, which are an upgrade of traditional
ones.
• Collection and Review of Datasets. Beyond the
models, we considered the possible characteris-
tics of time-series datasets, especially those time-
series-specific characteristics. A prevalent one
is related to whether the dataset is stationary or
not. Also, time-series datasets may exhibit sea-
sonality. After a thorough search, we obtained
six complete time-series datasets: AEP hourly
(Robikscube, 2023), Air Passengers (Peixeiro,
2022), Steel industry data (csafrit2, 2023), Cana-
dian climate history (bmonikraj, 2023), micro-
data (Peixeiro, 2022), and DailyDelhiClimate
(sumanthvrao, 2023).
ICEIS 2025 - 27th International Conference on Enterprise Information Systems
814