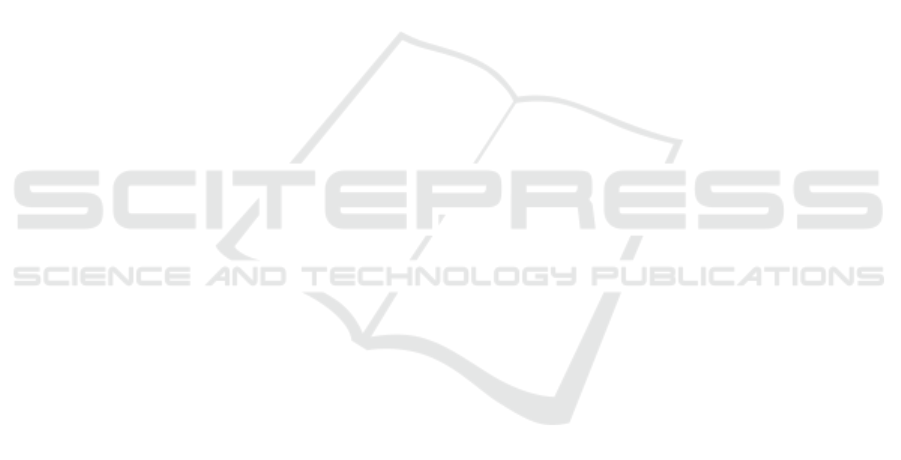
mission model (CTM)–based congestion propaga-
tion model where the average trip velocity (AJV)
is used to calculate bottleneck. Bottleneck identi-
fication method for Kaohsiung city is proposed in
(Yue et al., 2018) where causal congestion tree is
used on loop detector data. In addition, a map
data-driven bottleneck identification approach is pre-
sented in (Mirzahossein et al., 2024) for Tehran
city. Besides these, wavelet-based bottleneck detec-
tion for Washington (Ke et al., 2018), congestion
propagation-based method for Taipei (Li et al., 2020),
and bottleneck detection approach for Wisconsin (Jin
et al., 2012) are proposed. Though these existing ap-
proaches provide valuable insights, their dependency
on sophisticated technology, high costs, or lack of
adaptability to highly congested, resource-limited ur-
ban settings makes them unsuitable for Dhaka city.
In addition, there is minimal infrastructure to collect
traffic congestion data for Dhaka city. Apart from the
lack of traffic data, every city has a unique road lay-
out and transportation system (Rahman and Nower,
2023). These factors significantly impact traffic con-
gestion, as a result, we cannot simply apply other
bottleneck detection approaches in our capital city
Dhaka.
Dhaka is the fifth most congested city in the world
with an estimated 17 million people living in an area
of 1,528 square kilometers. According to the Asian
Development Bank (ADB), average speeds in some
places have dropped to as low as 7–10 km/h dur-
ing peak hours because of severe traffic congestion
(Moshiur Rahman and Nower, 2024). As a result,
bottlenecks, as the most contributing factor to traf-
fic congestion, are necessary to identify for this city.
Bottlenecks are created by several factors in this city:
inadequate road infrastructure, an ever-growing num-
ber of private vehicles, poor traffic management, etc.
These choke points not only slow down traffic but
also lead to ripple effects across the entire network,
where even a minor delay at a bottleneck can cre-
ate significant congestion downstream. The cumula-
tive effect of these bottlenecks exacerbates the con-
gestion problem, making it challenging to maintain
consistent traffic flow throughout the city (Hossain
and Nower, 2022). Thus we need a cost-effective,
adaptable method for bottleneck identification tai-
lored to the constraints and unique congestion pat-
terns of Dhaka, aiming to bridge the gap left by ex-
isting research methods.
To address this gap, this study proposes a novel
urban traffic bottleneck identification approach based
on Congestion Spreading Graph (CSG) and Maxi-
mal Spanning Tree (MST) analysis using traffic in-
tensity data calculated from Google Map snapshots.
The proposed approach collects traffic data using our
previously developed tool (Hossain and Nower, 2022)
by processing Google map snapshots. By leveraging
traffic data and advanced algorithms, our approach
aims to identify specific bottleneck points accurately
throughout Dhaka’s road network. This data-driven
solution can offer affordable solutions and actionable
insights to policymakers and urban planners, help-
ing them to create targeted interventions that address
Dhaka’s unique traffic challenges more effectively.
Our primary contributions are as follows:
• A novel methodology is developed using a combi-
nation of snapshot processing, graphical models,
maximal spanning trees, and Markov analysis to
model and analyze congestion spreading in urban
roads. It offers an effective way to quantify both
the spread of congestion and the congestion costs
of individual road links.
• Validation using SUMO demonstrates that the
proposed method identifies Dhaka city’s bottle-
necks properly.
2 RELATED WORK
With the increase in urban dynamics, the urban trans-
port system is becoming more crucial in the citizen’s
daily life. The bottleneck, the most critical road seg-
ment is one of the main reasons for traffic congestion.
Thus, these critical road segments, or bottlenecks,
must be identified in road networks. Once the conges-
tion on the identified bottlenecks is reduced by using
these sophisticated traffic control/management tech-
niques, the overall traffic network’s conditions will
be effectively and efficiently improved. The existing
bottleneck identification studies on different cities are
highlighted in this section.
Numerous methods have been developed to iden-
tify traffic bottlenecks in different transport network
settings. (Long et al., 2008) introduced a congestion
propagation model for urban networks using the cell
transmission model (CTM), incorporating link and
node models to simulate flow propagation and iden-
tify bottlenecks. The model estimates average jour-
ney velocity (AJV) and demonstrates, through sim-
ulations on the Sioux Falls network, that increasing
traffic demand is a key factor in bottleneck forma-
tion, influenced by link position, flow composition,
and network demand. While their approach identified
bottlenecks, the study was limited by a lack of real-
time data and relied heavily on predefined thresholds
which can be highly varied from city to city. More-
over, this method cannot effectively represent traffic
VEHITS 2025 - 11th International Conference on Vehicle Technology and Intelligent Transport Systems
126