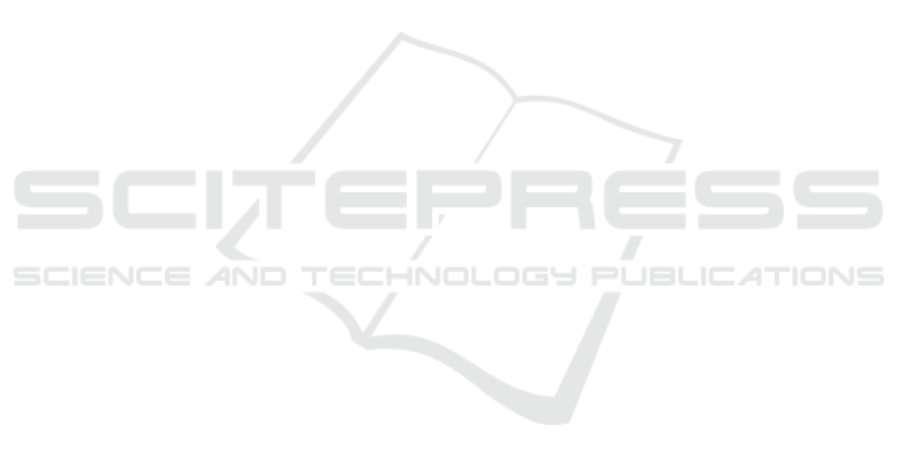
able feature learning for networks. In Proceedings
of the 22nd ACM SIGKDD International Conference
on Knowledge Discovery and Data Mining, page
855–864. Association for Computing Machinery.
Hochreiter, S. and Schmidhuber, J. (1997). Long short-term
memory. Neural Comput., 9(8):1735–1780.
Huaiyu Wan, Yan Lin, S. G. and Lin, Y. (2022). Pre-
training time-aware location embeddings from spatial-
temporal trajectories. IEEE Transactions on Knowl-
edge and Data Engineering, 34(11):5510–5523.
Jiawei Jiang, Dayan Pan, H. R. X. J. C. L. and Wang,
J. (2023). Self-supervised trajectory representation
learning with temporal regularities and travel seman-
tics. In 39th IEEE International Conference on Data
Engineering, ICDE 2023, Anaheim, CA, USA, April
3-7, 2023, pages 843–855. IEEE.
Joshua Robinson, Ching-Yao Chuang, S. S. and Jegelka, S.
(2020). Contrastive learning with hard negative sam-
ples. ArXiv, abs/2010.04592.
Kingma, D. P. and Ba, J. (2015). Adam: A method for
stochastic optimization. In 3rd International Confer-
ence on Learning Representations, ICLR 2015, San
Diego, CA, USA, May 7-9, 2015, Conference Track
Proceedings.
Meng-xiang Wang, Wang-Chien Lee, T.-y. F. and Yu, G.
(2019). Learning embeddings of intersections on road
networks. In Proceedings of the 27th ACM SIGSPA-
TIAL International Conference on Advances in Ge-
ographic Information Systems, pages 309–318, New
York, NY, USA. Association for Computing Machin-
ery.
Ning Wu, Xin Wayne Zhao, J. W. and Pan, D. (2020).
Learning effective road network representation with
hierarchical graph neural networks. In Proceedings
of the 26th ACM SIGKDD International Conference
on Knowledge Discovery & Data Mining, pages 6–
14, New York, NY, USA. Association for Computing
Machinery.
Peng Han, Jin Wang, D. Y. S. S. and Zhang, X. (2021). A
graph-based approach for trajectory similarity compu-
tation in spatial networks. In Proceedings of the 27th
ACM SIGKDD Conference on Knowledge Discovery
and Data Mining, pages 556–564, Virtual Event, Sin-
gapore. Association for Computing Machinery.
Petar Velickovic, Guillem Cucurull, A. C. A. R. P. L. and
Bengio, Y. (2017). Graph attention networks. ArXiv,
abs/1710.10903.
Philip Bachman, R. D. H. and Buchwalter, W. (2019).
Learning Representations by Maximizing Mutual In-
formation Across Views, pages 1–11. Curran Asso-
ciates Inc., Red Hook, NY, USA.
Scarselli, F., Gori, M., Tsoi, A. C., Hagenbuchner, M.,
and Monfardini, G. (2009). The graph neural net-
work model. IEEE Transactions on Neural Networks,
20(1):61–80.
Ting Chen, Simon Kornblith, M. N. and Hinton, G. (2020).
A simple framework for contrastive learning of visual
representations. In Proceedings of the 37th Interna-
tional Conference on Machine Learning, pages 1–11.
JMLR.org.
Tobias Skovgaard Jepsen, C. S. J. and Nielsen, T. D. (2019).
Graph convolutional networks for road networks. In
Proceedings of the 27th ACM SIGSPATIAL Interna-
tional Conference on Advances in Geographic Infor-
mation Systems, pages 460–463, New York, NY, USA.
Association for Computing Machinery.
Velickovic, P., Fedus, W., Hamilton, W. L., Li
`
o, P., Bengio,
Y., and Hjelm, R. D. (2019). Deep graph infomax. In
7th International Conference on Learning Represen-
tations, ICLR 2019, New Orleans, LA, USA, May 6-9,
2019. OpenReview.net.
Xiucheng Li, Kaiqi Zhao, G. C. C. S. J. and Wei, W. (2018).
Deep representation learning for trajectory similarity
computation. In 2018 IEEE 34th International Con-
ference on Data Engineering (ICDE), pages 617–628.
IEEE.
Yangxin Lin, P. W. and Ma, M. (2017). Intelligent trans-
portation system (its): Concept, challenge and oppor-
tunity. In 2017 IEEE 3rd International Conference on
Big Data Security on Cloud (BigDataSecurity), IEEE
International Conference on High Performance and
Smart Computing (HPSC), and IEEE International
Conference on Intelligent Data and Security (IDS),
pages 167–172. IEEE.
Yannis Kalantidis, Mert B
¨
ulent Sariyildiz, N. P. P. W. and
Larlus, D. (2020). Hard negative mixing for con-
trastive learning. In Advances in Neural Information
Processing Systems 33: Annual Conference on Neural
Information Processing Systems 2020, NeurIPS 2020,
December 6-12, 2020, virtual. NeurIPS.
Yile Chen, Xiucheng Li, G. C. Z. B. C. L. Y. L. A. K. C.
and Ellison, R. (2021). Robust road network repre-
sentation learning: When traffic patterns meet travel-
ing semantics. In Proceedings of the 30th ACM Inter-
national Conference on Information and Knowledge
Management, pages 211–220, Virtual Event, Queens-
land, Australia. Association for Computing Machin-
ery.
Yoshua Bengio, A. C. and Vincent, P. (2013). Representa-
tion learning: A review and new perspectives. IEEE
Transactions on Pattern Analysis and Machine Intel-
ligence, 35(8):1798–1828.
Yu Zheng, Lizhu Zhang, X. X. and Ma, W.-Y. (2009). Min-
ing interesting locations and travel sequences from
GPS trajectories. In Proceedings of the 18th Inter-
national Conference on World Wide Web, pages 791–
800, Madrid, Spain. Association for Computing Ma-
chinery.
Zhenyu Mao, Ziyue Li, D. L. L. B. and Zhao, R. (2022).
Jointly contrastive representation learning on road net-
work and trajectory. In Proceedings of the 31st ACM
International Conference on Information & Knowl-
edge Management, pages 1501–1510, New York, NY,
USA. Association for Computing Machinery.
Time-Aware Contrastive Representation Learning for Road Network and Trajectory
505