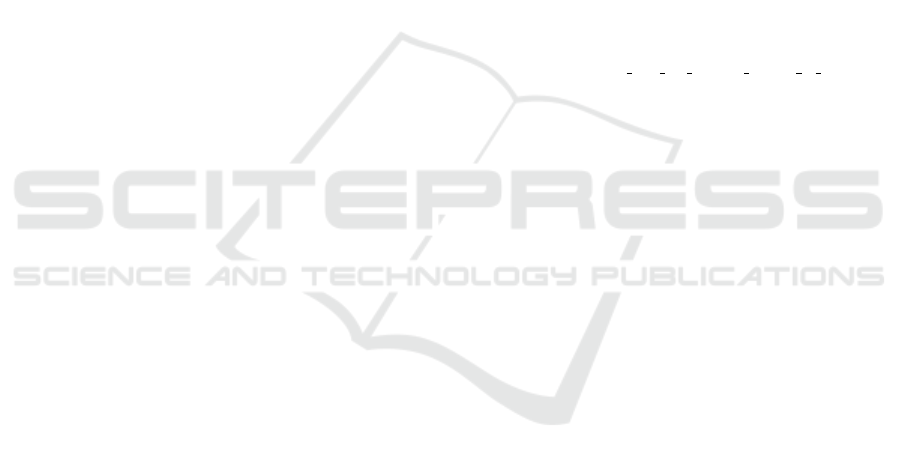
ing of an internet of things platform. In International
Conference on Internet of Things, Big Data and Secu-
rity.
Frappier, M., Fraikin, B., Chossart, R., Chane-Yack-Fa, R.,
and Ouenzar, M. (2010). Comparison of model check-
ing tools for information systems. In Dong, J. S. and
Zhu, H., editors, Formal Methods and Software Engi-
neering, pages 581–596. Springer Berlin Heidelberg.
Gani, K., Bouet, M., Schneider, M., and Toumani, F. (2015).
Using timed automata framework for modeling home
care plans. In 2015 International Conference on Ser-
vice Science (ICSS), pages 1–8.
Gerking, C., Schubert, D., and Bodden, E. (2018). Model
checking the information flow security of real-time
systems. In Engineering Secure Software and Systems
: 10th International Symposium, ESSoS 2018, volume
10953, page 27–43.
Goguen, J. A. and Meseguer, J. (1982). Security policies
and security models. In 1982 IEEE Symposium on
Security and Privacy, pages 11–11.
Klier, M., Moestue, L., Obermeier, A., and Widmann, T.
(2024). Assessing completeness of IoT data: A novel
probabilistic approach. Business & Information Sys-
tems Engineering, pages 1–18.
Kwiatkowska, M. and Parker, D. (2012). Advances in prob-
abilistic model checking. In Software Safety and Se-
curity, pages 126–151. IOS Press.
Larsen, K. G., Pettersson, P., and Yi, W. (1995). Model-
checking for real-time systems. In Reichel, H., editor,
Fundamentals of Computation Theory, pages 62–88.
Springer Berlin Heidelberg.
Lee, G. M. and Truong, N. B. (2016). A reputation and
knowledge based trust service platform for trustwor-
thy social internet of things. In Innovations in Clouds,
Internet and Networks (ICIN).
Li, S. and Xu, L. D. (2017). Securing the Internet of Things.
Syngress Publishing, 1st edition.
Mafamane, R., Ouadou, M., Hassani, A., and Minaoui, K.
(2021). Study of the heterogeneity problem in the in-
ternet of things and cloud computing integration. In
2020 10th International Symposium on Signal, Image,
Video and Communications (ISIVC).
Mainuddin, M., Duan, Z., and Dong, Y. (2021). Net-
work traffic characteristics of IoT devices in smart
homes. In 2021 International Conference on Com-
puter Communications and Networks (ICCCN), pages
1–11. IEEE.
McCall, M., Zeng, E., Shezan, F. H., Yang, M., Bauer,
L., Bichhawat, A., Cobb, C., Jia, L., and Tian, Y.
(2023). Towards usable security analysis tools for
Trigger-Action programming. In Nineteenth Sympo-
sium on Usable Privacy and Security (SOUPS 2023),
pages 301–320, Anaheim, CA. USENIX Association.
Neagle, C. (2015). Smart refrigerator
hack exposes gmail login credentials.
https://www.networkworld.com/article/2976270/smart-
refrigerator-hack-exposes-gmail-login-
credentials.html. Accessed: 2023-10-10.
Ngo, T. and Nguyen, N. (2019). Secure information flow
for IoT applications. JOIV : International Journal on
Informatics Visualization, 3:192–197.
Nguyen, D. T., Song, C., Qian, Z., Krishnamurthy, S. V.,
Colbert, E. J. M., and Mcdaniel, P. (2018). IoTSan:
fortifying the safety of IoT systems. Proceedings of
the 14th International Conference on emerging Net-
working EXperiments and Technologies.
Nunes, D., Zhang, P., and S
´
a Silva, J. (2015). A survey on
human-in-the-loop applications towards an internet of
all. IEEE Communications Surveys & Tutorials.
Park, M., Oh, H., and Lee, K. (2019). Security risk mea-
surement for information leakage in iot-based smart
homes from a situational awareness perspective. Sen-
sors, 19(9).
Pradeep, P. and Kant, K. (2022). Conflict detection and
resolution in IoT systems: A survey. IoT, 3(1):191–
218.
Radovici, A., Culic, I., Rosner, D., and Oprea, F. (2020).
A model for the remote deployment, update, and safe
recovery for commercial sensor-based IoT systems.
Sensors, 20(16).
Reddit (2018). Roomba sets off motion sensor at night?
https://www.reddit.com/r/homeautomation/comments/
92ahq3/roomba sets off motion sensor at night/.
Accessed: 2023-10-10.
Sabelfeld, A. and Myers, A. C. (2003). Language-based
information-flow security. IEEE J. Sel. Areas Com-
mun., 21:5–19.
Schneider, F. B. (2000). Enforceable security policies. ACM
Trans. Inf. Syst. Secur., 3(1):30–50.
Sha, L., Xiao, F., Chen, W., and Sun, J. (2018). Iiot-
sidefender: Detecting and defense against the sensi-
tive information leakage in industry iot. World Wide
Web, 21:59–88.
Sobin, C. (2020). A survey on architecture, protocols and
challenges in IoT. Wireless Personal Communica-
tions, 112(3):1383–1429.
Stevens, C., Alhanahnah, M., Yan, Q., and Bagheri, H.
(2020). Comparing formal models of iot app coordi-
nation analysis. In Proceedings of the 3rd ACM SIG-
SOFT International Workshop on Software Security
from Design to Deployment, page 3–10. Association
for Computing Machinery.
Uppsala and Aalborg (2020). Uppaal. https://uppaal.org/.
Accessed: 2023-10-18.
Wang, Q., Hassan, W. U., Bates, A., and Gunter, C. A.
(2018). Fear and logging in the internet of things. In
Network and Distributed System Security Symposium.
Yu, D., Li, P., Chen, Y., Ma, Y., and Chen, J. (2020). A
time-efficient multi-protocol probe scheme for fine-
grain IoT device identification. Sensors, 20.
Zetter, K. (2014). Hospital networks are leak-
ing data, leaving critical devices vulnerable.
https://www.wired.com/2014/06/hospital-networks-
leaking-data/. Accessed: 2023-10-10.
Zhang, L. (2017). Fragmentation in IoT
– one roadblock in IoT deployment.
https://www.cleantech.com/fragmentation-in-iot-
one-roadblock-in-iot-deployment/. Accessed:
2023-10-18.
IoTBDS 2025 - 10th International Conference on Internet of Things, Big Data and Security
116