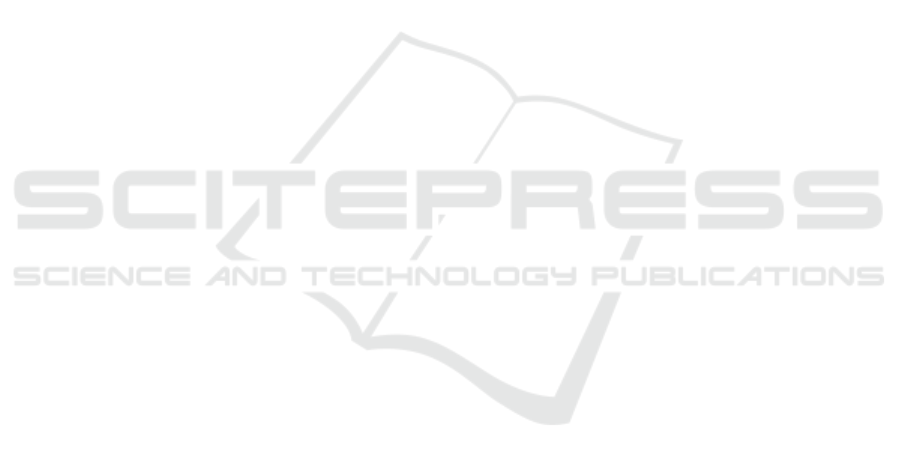
reaching this point it is essential to have well formed
learning approaches that are actually capable to ad-
dress the problem in a reliable manner. The current
incremental expansion on PRNG learning is proposed
to provide stepping stones such that robust security
evaluation may be provided for complex PRNGs in
the context of IoT in the future.
7 CONCLUSION
The present paper examines the manner in which ex-
isting literature approaches for ANN learning on LF-
SRs scale in the case of higher LFSR degrees. More-
over, it formulates a novel Geffe learning task to miti-
gate the excessive use of domain knowledge in previ-
ous approaches. While the results are highly accurate,
challenges are identified in terms of the number of
models that need to be trained for various Geffe con-
figurations. To address these challenges, the present
paper capitalizes on model visualization corroborated
with the ANN ability towards the perfect learning
of deterministic functions to derive an optimization.
A wide discussion ensues on the implications of the
aforementioned concepts for the problem of learning
from PRNGs and the solution is regarded in the con-
text of improving IoT evaluation of PRNG security by
means of neural networks.
REFERENCES
Amigo, G., Dong, L., and Ii, R. J. M. (2021). Forecast-
ing pseudo random numbers using deep learning. In
2021 15th International Conference on Signal Pro-
cessing and Communication Systems (ICSPCS), pages
1–7. IEEE.
Boanca, S. (2024). Exploring patterns and assessing the se-
curity of pseudorandom number generators with ma-
chine learning. In ICAART (3), pages 186–193.
Boanc
˘
a, S. (2024). Optimizations for learning from linear
feedback shift register variations with artificial neural
networks. In IFIP International Conference on Artifi-
cial Intelligence Applications and Innovations, pages
197–210. Springer.
Cui, L., Yang, S., Chen, F., Ming, Z., Lu, N., and Qin, J.
(2018). A survey on application of machine learning
for internet of things. International Journal of Ma-
chine Learning and Cybernetics, 9:1399–1417.
Feng, Y. and Hao, L. (2020). Testing randomness using
artificial neural network. IEEE Access, 8:163685–
163693.
Fischer, T. (2018). Testing cryptographically secure pseudo
random number generators with artificial neural net-
works. In 2018 17th IEEE International Confer-
ence On Trust, Security And Privacy In Computing
And Communications/12th IEEE International Con-
ference On Big Data Science And Engineering (Trust-
Com/BigDataSE), pages 1214–1223. IEEE.
Gohr, A. (2019). Improving attacks on round-reduced
speck32/64 using deep learning. In Advances in
Cryptology–CRYPTO 2019: 39th Annual Interna-
tional Cryptology Conference, Santa Barbara, CA,
USA, August 18–22, 2019, Proceedings, Part II 39,
pages 150–179. Springer.
Gupta, S., Singh, P., Shrotriya, N., and Baweja, T. (2021).
Lfsr next bit prediction through deep learning. Journal
of Informatics Electrical and Electronics Engineering
(JIEEE), 2(2):1–9.
Hussain, F., Hussain, R., Hassan, S. A., and Hossain, E.
(2020). Machine learning in iot security: Current so-
lutions and future challenges. IEEE Communications
Surveys & Tutorials, 22(3):1686–1721.
Ince, K. (2024). Exploring the potential of deep learning
and machine learning techniques for randomness anal-
ysis to enhance security on iot. International Journal
of Information Security, 23(2):1117–1130.
John Labelle (2020). Everyone Talks About Insecure Ran-
domness, But Nobody Does Anything About It. https:
//www.airza.net/2020/11/09/everyone-talks-about-i
nsecure-randomness-but-nobody-does-anything-abo
ut-it.html. Online; accessed 21 November 2022.
Kant, S. and Khan, S. S. (2006). Analyzing a class of
pseudo-random bit generator through inductive ma-
chine learning paradigm. Intelligent Data Analysis,
10(6):539–554.
Kant, S., Kumar, N., Gupta, S., Singhal, A., and Dhasmana,
R. (2009). Impact of machine learning algorithms
on analysis of stream ciphers. In 2009 Proceeding
of international conference on methods and models in
computer science (ICM2CS), pages 251–258. IEEE.
Kietzmann, P., Schmidt, T. C., and W
¨
ahlisch, M. (2021). A
guideline on pseudorandom number generation (prng)
in the iot. ACM Computing Surveys (CSUR), 54(6):1–
38.
Kim, J. and Kim, H. (2021). Length of pseudorandom bi-
nary sequence required to train artificial neural net-
work without overfitting. IEEE Access, 9:125358–
125365.
L’Ecuyer, P. and Simard, R. (2007). Testu01: Ac li-
brary for empirical testing of random number gener-
ators. ACM Transactions on Mathematical Software
(TOMS), 33(4):1–40.
Li, C., Zhang, J., Sang, L., Gong, L., Wang, L., Wang,
A., and Wang, Y. (2020). Deep learning-based secu-
rity verification for a random number generator using
white chaos. Entropy, 22(10):1134.
Lv, N., Chen, T., Zhu, S., Yang, J., Ma, Y., Jing, J., and Lin,
J. (2020). High-efficiency min-entropy estimation
based on neural network for random number genera-
tors. Security and Communication Networks, 2020:1–
18.
Massey, J. (1969). Shift-register synthesis and bch de-
coding. IEEE transactions on Information Theory,
15(1):122–127.
Pseudorandom Number Generators, Perfect Learning and Model Visualization with Neural Networks: Expanding on LFSRs and Geffe
127