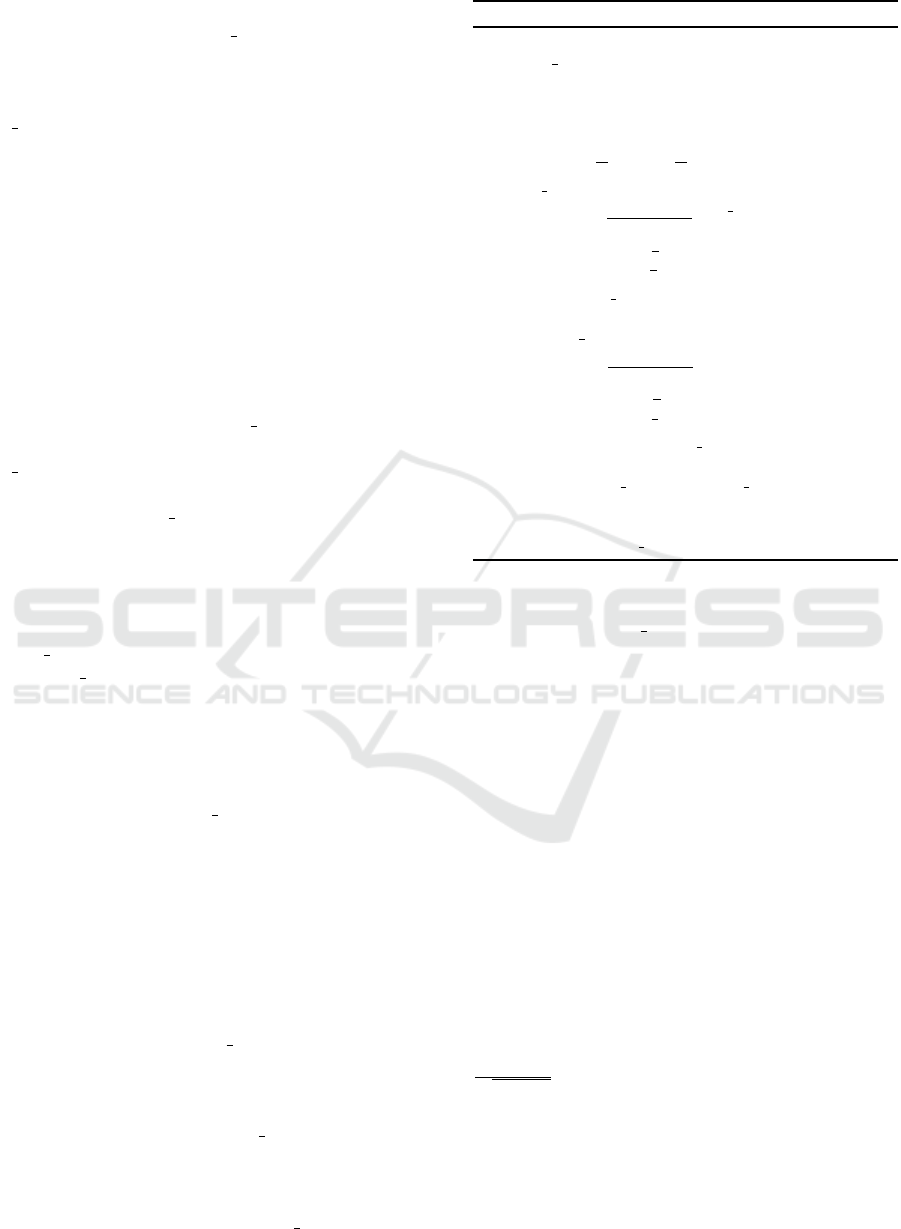
(x,y, z) point from every point cloud frame p in P and
computes its index (r,c) in r map using the spherical
projection described in Eq. 1 and 2 (lines 3-7). In line
8, the algorithm finds the nearest neighbor of (x,y,z)
by performing a query in the kd-tree at index (r, c) of
r map. If a nearest neighbor is found within the given
distance threshold D
t
, the algorithm updates the fre-
quency of that nearest neighbor in the kd-tree (lines
9-10). Otherwise, the algorithm inserts a new 4-tuple
value (x, y,z,1) into the kd-tree (lines 11-13) to indi-
cate that this (x,y,z) point has not been observed in
any previous frames.
Next, the algorithm computes the frequency
threshold and separates the background points based
on that threshold (lines 16-34). To do so, an empty
map f r is initialized (line 16), where the key rep-
resents the frequency of the (x , y,z) points across
all frames, and the value indicates how many times
that frequency occurs in the r map. The algorithm
then iterates through each tuple (x,y,z,c) from the
r map and increments the frequency count for c in
the map f r (lines 17-19). Subsequently, the fre-
quency threshold f r threshold is computed by ana-
lyzing the key-value pairs of the map f r and iden-
tifying the point where the slope of the frequency
curve of point frequencies becomes positive (lines 20-
27). Finally, in lines 28-34, the algorithm marks the
(x,y, z) points with a frequency greater than or equal
to f r threshold as background points, stores them in
the set bg points, and returns the set.
Once the background points are identified, filter-
ing them from a point cloud frame p is a straightfor-
ward process. Algorithm 2 describes the filtering pro-
cedure. The algorithm takes a point cloud frame p,
from which the background points need to be filtered,
a set of (x,y,z) points bg points identified as back-
grounds points, LiDAR vertical resolution φ
l
, LiDAR
horizontal resolution θ
l
, LiDAR vertical field of view
V , LiDAR horizontal field of view H, and a nearest
neighbor distance threshold D
t
and outputs a set of
non-background (x, y,z) points. The algorithm works
as follows:
Initially, the background points are transformed
into 2-D space using the same spherical projection
method as described in Algorithm 1, and they are
stored in the range map r map (lines 1-8). Sub-
sequently, for each point (x,y, z) in the point cloud
frame p, the algorithm computes its spherical pro-
jection (r,c) and searches for the nearest neighbor in
the kd-tree indexed at (r, c) in r map (lines 9-14). If
no nearest neighbor is found within the specified dis-
tance threshold D
t
, the point (x,y, z) is classified as
a non-background point (lines 15-17). Consequently,
the algorithm adds it to the set nonbg points (line 16).
Algorithm 2: Background Filtering Algorithm.
Require: a point cloud frame p, a set of (x, y,z) points
bg points, LiDAR vertical resolution φ
l
, LiDAR
horizontal resolution θ
l
, LiDAR V-FoV V , LiDAR
H-FoV H, nearest neighbor distance threshold D
t
Ensure: A set of (x, y,z) points, which are kept as
non-background points.
1: rows ←
V
φ
l
,cols ←
H
θ
l
2: r map(1 : rows,1 : cols) ←
/
0
3: for each point (x,y,z) ∈ bg points do
4: d ←
p
x
2
+ y
2
+ z
2
5: r ← ⌊arccos (
z
d
)/φ
l
⌋
6: c ← ⌊arctan (
x
y
)/θ
l
⌋
7: insert(r map(r,c),(x,y,z))
8: end for
9: nonbg points ←
/
0
10: for each point (x,y,z) ∈ p do
11: d ←
p
x
2
+ y
2
+ z
2
12: r ← ⌊arccos (
z
d
)/φ
l
⌋
13: c ← ⌊arctan (
x
y
)/θ
l
⌋
14: (x
n
,y
n
,z
n
) ← NN(r map(r,c),(x, y,z), D
t
)
15: if (x
n
,y
n
,z
n
) =
/
0 then
16: nonbg points ← nonbg points ∪{(x,y,z)}
17: end if
18: end for
19: return nonbg points
After processing all points in frame p, the algorithm
returns the set nonbg points as the output (line 19).
3.3 Network-Aware Compression
Background filtering achieves significant temporal
compression (discussed in Section 4.5) by removing
repeated background points across multiple frames.
To further reduce the size of the data, we perform
spatial compression on the filtered point cloud frames
by using 3-D plane fitting method. Since most of the
real-world surfaces are 3-D planes e.g., sides of cars,
ground, etc., all points that lie on the same plane can
be encoded by using that plane. We can also approxi-
mate non-plane surfaces by using a set of planes.
As discussed in (Feng et al., 2020), a plane in 3-D
Cartesian space can be represented as:
x + ay + bz −c = 0, (3)
where (1,a,b) is the normal vector of the plane and
|c|
√
1+a
2
+b
2
is the distance from the origin i.e., center
of the LiDAR. Hence, we can encode all points on the
same plane with just three coefficients. Since the ex-
act position of each point on the plane is not explicitly
encoded, we need to perform a ray casting process to
find the intersection of a ray and the plane to recon-
struct the position of a point.
VEHITS 2025 - 11th International Conference on Vehicle Technology and Intelligent Transport Systems
140