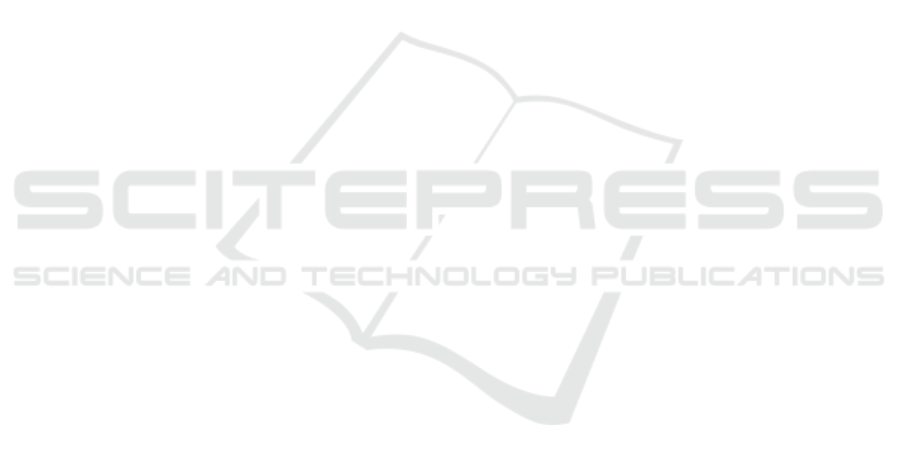
Devlin, J., Chang, M.-W., Lee, K., and Toutanova, K.
(2018). Bert: Pre-training of deep bidirectional trans-
formers for language understanding. arXiv preprint
arXiv:1810.04805.
Enamoto, L., Santos, A. R., Maia, R., Weigang, L., and
Filho, G. P. R. (2022). Multi-label legal text classifi-
cation with bilstm and attention. International Journal
of Computer Applications in Technology, 68(4):369–
378.
Feuerriegel, S., Hartmann, J., Janiesch, C., and Zschech, P.
(2024). Generative ai. Business & Information Sys-
tems Engineering, 66(1):111–126.
Frank, E. and Paynter, G. W. (2004). Predicting library of
congress classifications from library of congress sub-
ject headings. Journal of the American Society for
Information Science and Technology, 55(3):214–227.
Gao, A. (2023). Prompt engineering for large language
models. Available at SSRN 4504303.
Giglou, H. B., D’Souza, J., and Auer, S. (2024).
Llms4synthesis: Leveraging large language
models for scientific synthesis. arXiv preprint
arXiv:2409.18812.
Golub, K., Hagelb
¨
ack, J., and Ard
¨
o, A. (2020). Automatic
classification of swedish metadata using dewey deci-
mal classification: a comparison of approaches. Jour-
nal of Data and Information Science, 5(1):18–38.
Hacker, P., Engel, A., and Mauer, M. (2023). Regulating
chatgpt and other large generative ai models. In Pro-
ceedings of the 2023 ACM Conference on Fairness,
Accountability, and Transparency, pages 1112–1123.
Herrmannova, D. and Knoth, P. (2016). An analysis
of the microsoft academic graph. D-lib Magazine,
22(9/10):37.
Huang, Z., Xu, W., and Yu, K. (2015). Bidirectional
lstm-crf models for sequence tagging. arXiv preprint
arXiv:1508.01991.
Jiang, M., D’Souza, J., Auer, S., and Downie, J. S. (2020).
Improving scholarly knowledge representation: Eval-
uating bert-based models for scientific relation classi-
fication. In Digital Libraries at Times of Massive So-
cietal Transition: 22nd International Conference on
Asia-Pacific Digital Libraries, ICADL 2020, Kyoto,
Japan, November 30–December 1, 2020, Proceedings
22, pages 3–19. Springer.
Kalyan, K. S. (2023). A survey of gpt-3 family large lan-
guage models including chatgpt and gpt-4. Natural
Language Processing Journal, page 100048.
Kinney, R., Anastasiades, C., Authur, R., Beltagy, I., Bragg,
J., Buraczynski, A., Cachola, I., Candra, S., Chan-
drasekhar, Y., Cohan, A., et al. (2023). The se-
mantic scholar open data platform. arXiv preprint
arXiv:2301.10140.
Liu, S., Yu, S., Lin, Z., Pathak, D., and Ramanan, D. (2024).
Language models as black-box optimizers for vision-
language models. In Proceedings of the IEEE/CVF
Conference on Computer Vision and Pattern Recogni-
tion, pages 12687–12697.
Mahapatra, R., Gayan, M., Jamatia, B., et al. (2024). Artifi-
cial intelligence tools to enhance scholarly communi-
cation: An exploration based on a systematic review.
Morgan, J. and Chiang, M. (2024). Ollama. https://ollama.
com. Online; accessed 6 August 2024.
Mosca, E., Abdalla, M. H. I., Basso, P., Musumeci, M., and
Groh, G. (2023). Distinguishing fact from fiction: A
benchmark dataset for identifying machine-generated
scientific papers in the llm era. In Proceedings of the
3rd Workshop on Trustworthy Natural Language Pro-
cessing (TrustNLP 2023), pages 190–207.
Murphy, K. P. (2022). Probabilistic machine learning: an
introduction. MIT press.
Nah, F., Cai, J., Zheng, R., and Pang, N. (2023). An activity
system-based perspective of generative ai: Challenges
and research directions. AIS Transactions on Human-
Computer Interaction, 15(3):247–267.
Pal, S., Bhattacharya, M., Islam, M. A., and Chakraborty, C.
(2024). Ai-enabled chatgpt or llm: a new algorithm is
required for plagiarism-free scientific writing. Inter-
national Journal of Surgery, 110(2):1329–1330.
Perełkiewicz, M. and Po
´
swiata, R. (2024). A review of the
challenges with massive web-mined corpora used in
large language models pre-training. arXiv preprint
arXiv:2407.07630.
Pertsas, V., Kasapaki, M., and Constantopoulos, P. (2024).
An annotated dataset for transformer-based scholarly
information extraction and linguistic linked data gen-
eration. In Proceedings of the 9th Workshop on Linked
Data in Linguistics@ LREC-COLING 2024, pages
84–93.
Rabby, G., Auer, S., D’Souza, J., and Oelen, A. (2024).
Fine-tuning and prompt engineering with cognitive
knowledge graphs for scholarly knowledge organiza-
tion. arXiv preprint arXiv:2409.06433.
Rous, B. (2012). Major update to acm’s computing clas-
sification system. Communications of the ACM,
55(11):12–12.
Scott, M. L. (1998). Dewey decimal classification. Li-
braries Unlimited.
Shahi, G. K. and Hummel, O. (2024). Enhancing re-
search information systems with identification of do-
main experts. In Proceedings of the Bibliometric-
enhanced Information Retrieval Workshop (BIR) at
the European Conference on Information Retrieval
(ECIR 2024), CEUR Workshop Proceedings. CEUR-
WS.org.
Shahi, G. K. and Nandini, D. (2020). FakeCovid – a
multilingual cross-domain fact check news dataset for
covid-19. In Proceedings of the 14th International
AAAI Conference on Web and Social Media.
Team, G., Mesnard, T., Hardin, C., Dadashi, R., Bhupati-
raju, S., Pathak, S., Sifre, L., Rivi
`
ere, M., Kale, M. S.,
Love, J., et al. (2024). Gemma: Open models based
on gemini research and technology. arXiv preprint
arXiv:2403.08295.
Touvron, H., Lavril, T., Izacard, G., Martinet, X., Lachaux,
M.-A., Lacroix, T., Rozi
`
ere, B., Goyal, N., Hambro,
E., Azhar, F., et al. (2023). Llama: Open and ef-
ficient foundation language models. arXiv preprint
arXiv:2302.13971.
Wang, J. (2009). An extensive study on automated
dewey decimal classification. Journal of the Ameri-
On the Effectiveness of Large Language Models in Automating Categorization of Scientific Texts
553