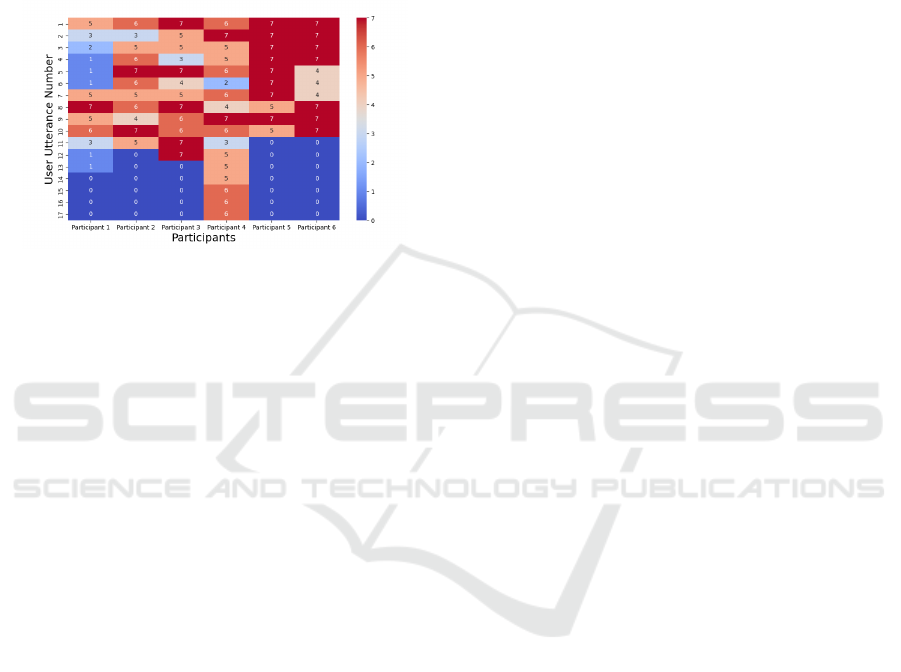
sine similarity threshold, suggesting the need for user-
adjustable thresholds for personalization.
Figure 6 presents a heatmap showing the appro-
priateness of assistance for user utterances across par-
ticipants. Assistance starts from the fourth utterance,
with warm-colored areas indicating appropriateness.
Participants 5 and 6 had relatively suitable frequen-
cies, while Participant 1 scored lower for early utter-
ances, highlighting insufficient assistance at the start.
Figure 6: Heatmap of Q9. Adequacy of assisted or unas-
sisted for each user statement (7-point Likert scale) for each
participant.
5.4 RQ4: Do the SCAINs Outputs Align
with the Users’ Intuition?
Specific qualitative evaluations are shown in the Ap-
pendix. Agreement with user intuition varied from
case to case. Confirming the conversations in the
case studies, SCAINs were more likely to occur when
short utterances or directives were used. For exam-
ple, “Let’s do that” and “Indeed”. While the system
was able to detect utterances that could be interpreted
differently depending on the context alone, there were
differences among users in terms of the effectiveness
and frequency of the assistance in resolving misun-
derstandings.
6 CONCLUSIONS
This paper introduced the SCAINs algorithm into a
chat system to identify utterances influencing inter-
pretation and highlight potential misunderstandings
caused by missing contextual utterances.
Case study results showed SCAINs occurrences
for all participants in an AI-driven, multimodal dis-
cussion task, with the system providing a seamless
experience. Feedback on SCAINs frequency was pos-
itive despite case-specific variations. Future work
will focus on enhancing prompt and threshold settings
and assessing the system’s effectiveness in human-to-
human interactions.
ACKNOWLEDGEMENTS
This work was supported by JST CREST Grant Num-
ber JPMJCR19A1, Japan.
REFERENCES
Feng, X. et al. (2022). A survey on dialogue summariza-
tion: Recent advances and new frontiers. In Proceed-
ings of the Thirty-First International Joint Conference
on Artificial Intelligence, IJCAI-22, pages 5453–5460.
International Joint Conferences on Artificial Intelli-
gence Organization.
Kishinami, Y. et al. (2023). Topic transition modelling in
human-to-human conversations. In Proceedings of the
29th Annual Meeting of the Association for Natural
Language Processing, pages 408–413.
Konigari, R. et al. (2021). Topic shift detection for mixed
initiative response. In Proceedings of the 22nd Annual
Meeting of the Special Interest Group on Discourse
and Dialogue, pages 161–166. Association for Com-
putational Linguistics.
Maekawa, T. and Imai, M. (2023). Identifying statements
crucial for awareness of interpretive nonsense to pre-
vent communication breakdowns. In Proceedings of
the 2023 Conference on Empirical Methods in Natu-
ral Language Processing, pages 12550–12566. Asso-
ciation for Computational Linguistics.
Nakanishi, K. et al. (2024). Extending contextual under-
standing techniques to account for missed listenings.
In Proceedings of the 38th Annual Conference of JSAI.
Neelakantan, A. et al. (2022). Text and code embeddings
by contrastive pre-training. ArXiv, abs/2201.10005.
Nihei, F. and Nakano, Y. (2020). Evaluating a multimodal
meeting summary browser that equipped an important
utterance detection model based on multimodal infor-
mation. In Proceedings of the 34th Annual Conference
of JSAI.
OpenAI (2023). Gpt-4 technical report. ArXiv,
abs/2303.08774.
Rossignac-Milon, M. et al. (2021). Merged minds: general-
ized shared reality in dyadic relationships. Journal of
Personality and Social Psychology, 120:882–911.
Sugiyama, H. et al. (2023). Empirical analysis of train-
ing strategies of transformer-based japanese chit-chat
systems. In 2022 IEEE Spoken Language Technology
Workshop (SLT), pages 685–691.
Sumita, K. et al. (1988). Information of the interpretation
for context understanding. In Proceedings of the 37th
National Convention of IPSJ, pages 1129–1130.
Tsuchiya, A. et al. (2024). Scains presenter: Preventing
miscommunication by detecting context-dependent
utterances in spoken dialogue. In Proceedings of
the 29th International Conference on Intelligent User
Interfaces, IUI ’24, page 549–565. Association for
Computing Machinery.
ICAART 2025 - 17th International Conference on Agents and Artificial Intelligence
1170