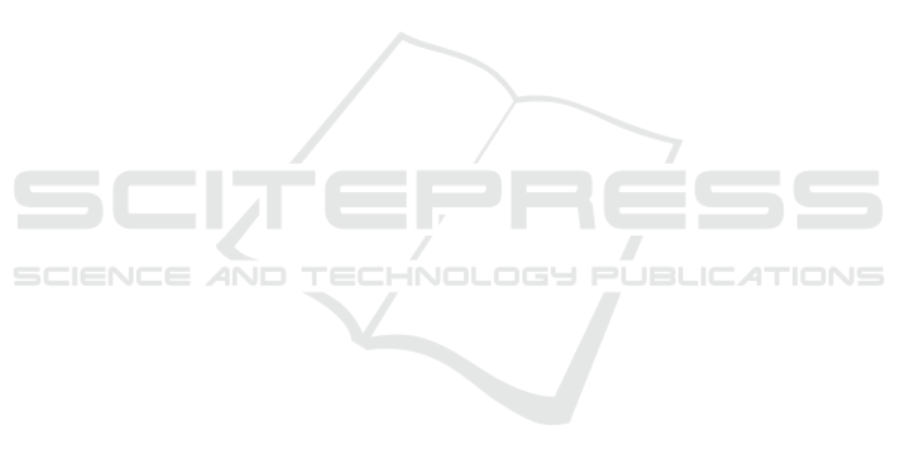
computer vision and pattern recognition, pages 605–
613.
Fey, M. and Lenssen, J. E. (2019). Fast graph represen-
tation learning with PyTorch Geometric. In ICLR
Workshop on Representation Learning on Graphs and
Manifolds.
Floater, M. S. and Surazhsky, T. (2006). Parameterization
for curve interpolation. In Studies in Computational
Mathematics, volume 12, pages 39–54. Elsevier.
Gadelha, M., Wang, R., and Maji, S. (2018). Multireso-
lution tree networks for 3D point cloud processing.
In Proceedings of the European Conference on Com-
puter Vision (ECCV), pages 103–118.
Goodfellow, I., Pouget-Abadie, J., Mirza, M., Xu, B.,
Warde-Farley, D., Ozair, S., Courville, A., and Ben-
gio, Y. (2020). Generative adversarial networks. Com-
munications of the ACM, 63(11):139–144.
Hamilton, W. L., Ying, R., and Leskovec, J. (2017). In-
ductive representation learning on large graphs. In
Proceedings of the 31st International Conference on
Neural Information Processing Systems, pages 1025–
1035.
Han, Z., Wang, X., Liu, Y.-S., and Zwicker, M. (2019).
Multi-angle point cloud-VAE: Unsupervised feature
learning for 3D point clouds from multiple angles by
joint self-reconstruction and half-to-half prediction. In
2019 IEEE/CVF International Conference on Com-
puter Vision (ICCV), pages 10441–10450. IEEE.
Huang, J. and Menq, C. (2002). Combinatorial manifold
mesh reconstruction and optimization from unorga-
nized points with arbitrary topology. Computer-Aided
Design, 34(2):149–165.
Huang, Z., Carr, N., and Ju, T. (2019). Variational implicit
point set surfaces. ACM Transactions on Graphics
(TOG), 38(4):1–13.
Huang, Z., Zou, M., Carr, N., and Ju, T. (2017). Topology-
controlled reconstruction of multi-labelled domains
from cross-sections. ACM Transactions on Graphics
(TOG), 36(4):1–12.
Kazhdan, M., Bolitho, M., and Hoppe, H. (2006). Poisson
surface reconstruction. In Eurographics.
Kipf, T. N. and Welling, M. (2017). Semi-supervised classi-
fication with graph convolutional networks. In 5th In-
ternational Conference on Learning Representations,
ICLR 2017.
Kuhn, H. W. and Tucker, A. W. (2014). Nonlinear program-
ming. In Traces and emergence of nonlinear program-
ming, pages 247–258. Springer.
Lazar, R., Dym, N., Kushinsky, Y., Huang, Z., Ju, T., and
Lipman, Y. (2018). Robust optimization for topo-
logical surface reconstruction. ACM Transactions on
Graphics (TOG), 37(4):1–10.
Lin, C.-H., Kong, C., and Lucey, S. (2018). Learning ef-
ficient point cloud generation for dense 3D object re-
construction. In proceedings of the AAAI Conference
on Artificial Intelligence, volume 32.
Liu, L., Bajaj, C., Deasy, J. O., Low, D. A., and Ju,
T. (2008). Surface reconstruction from non-parallel
curve networks. In Computer Graphics Forum, vol-
ume 27, pages 155–163. Wiley Online Library.
Memari, P. and Boissonnat, J.-D. (2008). Provably good 2D
shape reconstruction from unorganized cross-sections.
In Computer Graphics Forum, volume 27, pages
1403–1410. Wiley Online Library.
Park, J. J., Florence, P., Straub, J., Newcombe, R., and
Lovegrove, S. (2019). DeepSDF: Learning continuous
signed distance functions for shape representation. In
The IEEE Conference on Computer Vision and Pattern
Recognition (CVPR).
Peng, S., Jiang, C., Liao, Y., Niemeyer, M., Pollefeys, M.,
and Geiger, A. (2021). Shape as points: A differen-
tiable poisson solver. Advances in Neural Information
Processing Systems, 34:13032–13044.
Sarmad, M., Lee, H. J., and Kim, Y. M. (2019). RL-GAN-
Net: A reinforcement learning agent controlled gan
network for real-time point cloud shape completion.
In Proceedings of the IEEE/CVF Conference on Com-
puter Vision and Pattern Recognition, pages 5898–
5907.
Sawdayee, H., Vaxman, A., and Bermano, A. H.
(2022). Orex: Object reconstruction from planner
cross-sections using neural fields. arXiv preprint
arXiv:2211.12886.
Sharma, O. and Agarwal, N. (2017). Signed distance based
3D surface reconstruction from unorganized planar
cross-sections. Computers & Graphics, 62:67–76.
Van der Maaten, L. and Hinton, G. (2008). Visualizing data
using t-SNE. Journal of machine learning research,
9(11).
Vaswani, A., Shazeer, N., Parmar, N., Uszkoreit, J., Jones,
L., Gomez, A. N., Kaiser, Ł., and Polosukhin, I.
(2017). Attention is all you need. In Advances in
neural information processing systems, pages 5998–
6008.
Velickovic, P., Cucurull, G., Casanova, A., Romero, A., Liò,
P., and Bengio, Y. (2018). Graph attention networks.
In 6th International Conference on Learning Repre-
sentations, ICLR 2018.
Wen, X., Li, T., Han, Z., and Liu, Y.-S. (2020). Point cloud
completion by skip-attention network with hierarchi-
cal folding. In Proceedings of the IEEE/CVF Con-
ference on Computer Vision and Pattern Recognition,
pages 1939–1948.
Yin, K., Huang, H., Cohen-Or, D., and Zhang, H. (2018).
P2p-net: Bidirectional point displacement net for
shape transform. ACM Transactions on Graphics
(TOG), 37(4):1–13.
Zhou, K., Huang, X., Li, Y., Zha, D., Chen, R., and Hu, X.
(2020). Towards deeper graph neural networks with
differentiable group normalization. Advances in Neu-
ral Information Processing Systems, 33:4917–4928.
Zou, M., Holloway, M., Carr, N., and Ju, T. (2015).
Topology-constrained surface reconstruction from
cross-sections. ACM Transactions on Graphics
(TOG), 34(4):1–10.
GRAPP 2025 - 20th International Conference on Computer Graphics Theory and Applications
150