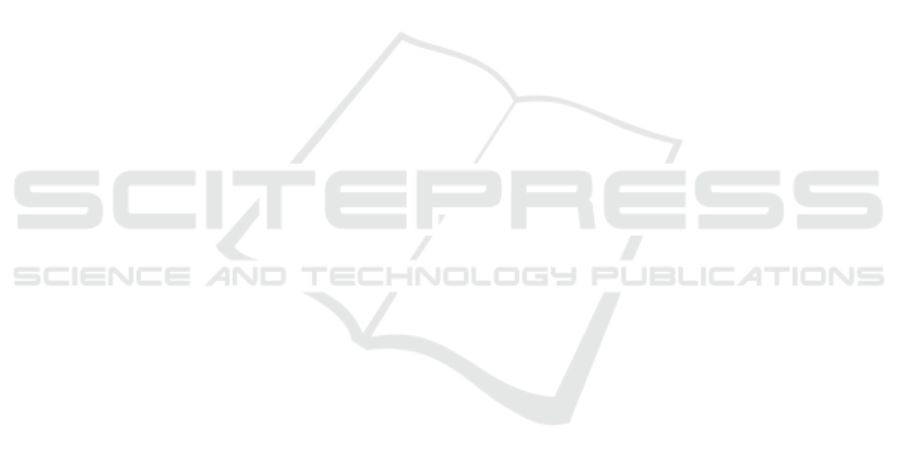
The use of the framework allowed us to automate
several repeatable tasks that are time consuming and
prone to errors, such as executing the algorithms with
correct parameters (Execution Module), collecting re-
sults from each execution (Data Import Module), pro-
cessing these results (Data Preprocessing Module),
generation of graphics (Data Visualization Module),
statistical analysis and linear regressions (Linear Re-
gression Module), and the data exporting to other for-
mats (Data Export Module). Furthermore, the modu-
lar design of the framework also allowed an easy in-
tegration of new algorithms.
Hence, OpenMP versions of two dynamic pro-
gramming algorithms (DP and UK) to compute the
edit distance between strings were also proposed and
evaluated. These algorithms were implemented in
C++ and executed in a multicore machine using the
proposed framework.
Our results with synthetic and real DNA se-
quences whose sizes ranged from 1000 to 30000 show
that our parallel versions are able to obtain very good
speedups (up to 5.88x, with 6 threads). Also, we show
that Parallel UK is the best choice for the majority of
the tests, producing the smallest execution time for
the synthetic and real sequences. Moreover, we show
that our framework generates automatically graphics,
simplifying the task of running experiments, and that
the linear regressions obtained with the framework
have very good statistical relevance (R
2
≥ 9.87), gen-
erating accurate execution time predictions.
As future work, we want to expand the linear re-
gression module of our framework, including addi-
tional plugins with machine learning strategies. In
addition, we want to incorporate other parallel ver-
sions of biological sequence comparison algorithms
for local and global alignment, such as MASA-
OpenCL (de Figueiredo Jr. et al., 2019), to our frame-
work. Finally, we intend to create a new module, that
will connect to the Data Export Module and use the
alignments produced by the executions in more com-
plex problems, such as Multiple Sequence Alignment.
REFERENCES
Berger, B., Waterman, M. S., and Yu, Y. W. (2021). Leven-
shtein distance, sequence comparison and biological
database search. IEEE Transactions on Information
Theory, 67(6):3287–3294.
Cock, P. J. A., Antao, T., Chang, J. T., Chapman, B. A., Cox,
C. J., Dalke, A., Friedberg, I., Hamelryck, T., Kauff,
F., Wilczynski, B., and de Hoon, M. J. L. (2009).
Biopython: freely available Python tools for compu-
tational molecular biology and bioinformatics. Bioin-
formatics, 25(11):1422–1423.
de Figueiredo Jr., M. A. C., de Oliveira Sandes, E. F., Ro-
drigues, G. N., Teodoro, G. L. M., and de Melo, A.
C. M. A. (2019). Masa-opencl: Parallel pruned com-
parison of long dna sequences with opencl. Con-
currency and Computation: Practice and Experience,
31(11):e5039. e5039 cpe.5039.
Figueiredo, M., Navarro, J. P., Sandes, E. F. O., Teodoro,
G., and Melo, A. C. M. A. (2021). Parallel fine-
grained comparison of long dna sequences in homo-
geneous and heterogeneous gpu platforms with prun-
ing. IEEE Transactions on Parallel and Distributed
Systems, 32(12):3053–3065.
Gundersen, O. (2021). The fundamental principles of repro-
ducibility. Philosophical Trans. of the Royal Society,
379:1–15.
Hall, P. A. V. and Dowling, G. R. (1980). Approx-
imate string matching. ACM Computing Surveys,
12(4):381–402.
Hidalgo, R., DeVito, A., Salah, N., S., V. A., and Mered-
ith, R. W. (2022). Inferring phylogenetic relationships
using the smith-waterman algorithm and hierarchical
clustering. In IEEE International Conference on Big
Data, pages 5910–5914. IEEE.
Huber, W., Carey, V. J., Gentleman, R., et al. (2015).
Orchestrating high-throughput genomic analysis with
bioconductor. Nature Methods, 12(2):115–121.
Levenshtein, V. I. (1966). Binary codes capable of correct-
ing deletions, insertions, and reversals. Soviet Physics
Doklady, 10:707–710.
Navarro, G. (2001). A guided tour to approximate string
matching. ACM Computing Surveys, 33(1):31–88.
Price, G., Nekrutenko, A., Gr
¨
uning, B. A., and Schatz,
M. C. (2024). The Galaxy platform for accessible,
reproducible, and collaborative data analyses: 2024
update. Nucleic Acids Research, 52(W1):W83–W94.
P
´
erez, F. and Granger, B. E. (2007). Ipython: A system for
interactive scientific computing. Computing in Sci-
ence & Engineering, 9(3):21–29.
Schmidt, B., Kallenborn, F., Chacon, A., and Hundt,
C. (2024). CUDASW++4.0: ultra-fast GPU-based
Smith-Waterman protein sequence database search.
BMC Bioinformatics, 25(1):342.
Sellers, P. (1974). On the theory and computation of evo-
lutionary distances. SIAM Journal of Applied Mathe-
matics, 26:787–793.
Teylo, L., Nunes, A. L., Melo, A. C. M. A., Boeres, C.,
Drummond, L. M. A., and Martins, N. F. (2021).
Comparing sars-cov-2 sequences using a commercial
cloud with a spot instance based dynamic scheduler.
In IEEE/ACM International Symposium on Cluster,
Cloud and Internet Computing, pages 24–256. IEEE.
Ukkonen, E. (1983). On approximate string matching. In
International Conference on Fundamentals of Com-
putation Theory, pages 487–495. Springer.
Uraki, R. e. a. (2023). Characterization of sars-cov-2 omi-
cron ba.2.75 clinical isolates. Nature Communica-
tions, 14(1):1620.
Wagner, R. A. and Fischer, M. (1974). The string-to-string
correction problem. Journal of the ACM, 1:168–173.
Wratten, L., Wilm, A., and Goke, J. (2021). Reproducible,
scalable, and shareable analysis pipelines with bioin-
formatics workflow managers. Nature Methods,
18:1161–1168.
BIOINFORMATICS 2025 - 16th International Conference on Bioinformatics Models, Methods and Algorithms
668