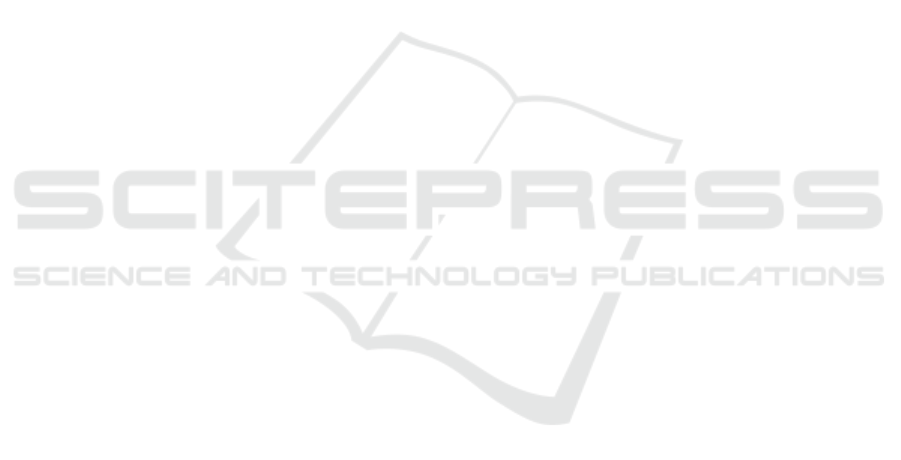
Liu, L., Zheng, Y., Tang, D., Yuan, Y., Fan, C., and Zhou,
K. (2019). Neuroskinning: Automatic skin binding
for production characters with deep graph networks.
ACM Transactions on Graphics (ToG), 38(4):1–12.
Mallya, A., Wang, T.-C., and Liu, M.-Y. (2022). Implicit
warping for animation with image sets. Advances
in Neural Information Processing Systems, 35:22438–
22450.
Ni, B., Peng, H., Chen, M., Zhang, S., Meng, G., Fu, J.,
Xiang, S., and Ling, H. (2022). Expanding language-
image pretrained models for general video recogni-
tion. In European Conference on Computer Vision,
pages 1–18. Springer.
Ni, H., Shi, C., Li, K., Huang, S. X., and Min, M. R.
(2023). Conditional image-to-video generation with
latent flow diffusion models. In Proceedings of the
IEEE/CVF Conference on Computer Vision and Pat-
tern Recognition, pages 18444–18455.
Patel, P., Gupta, H., and Chaudhuri, P. (2016). TraceMove:
A data-assisted interface for sketching 2D character
animation. In VISIGRAPP (1: GRAPP), pages 191–
199.
Poole, B., Jain, A., Barron, J. T., and Mildenhall, B. (2022).
DreamFusion: Text-to-3D using 2D diffusion. arXiv.
Poursaeed, O., Kim, V., Shechtman, E., Saito, J., and Be-
longie, S. (2020). Neural puppet: Generative layered
cartoon characters. In Proceedings of the IEEE/CVF
Winter Conference on Applications of Computer Vi-
sion, pages 3346–3356.
Radford, A., Kim, J. W., Hallacy, C., Ramesh, A., Goh, G.,
Agarwal, S., Sastry, G., Askell, A., Mishkin, P., Clark,
J., et al. (2021). Learning transferable visual models
from natural language supervision. In International
conference on machine learning, pages 8748–8763.
PMLR.
Rai, G., Gupta, S., and Sharma, O. (2024). Sketchanim:
Real-time sketch animation transfer from videos. In
Proceedings of the ACM SIGGRAPH/Eurographics
Symposium on Computer Animation, pages 1–11.
Santosa, S., Chevalier, F., Balakrishnan, R., and Singh, K.
(2013). Direct space-time trajectory control for visual
media editing. In Proceedings of the SIGCHI Confer-
ence on Human Factors in Computing Systems, pages
1149–1158.
Siarohin, A., Lathuili
`
ere, S., Tulyakov, S., Ricci, E., and
Sebe, N. (2019). First order motion model for image
animation. Advances in Neural Information Process-
ing Systems, 32.
Siarohin, A., Woodford, O. J., Ren, J., Chai, M., and
Tulyakov, S. (2021). Motion representations for ar-
ticulated animation. In Proceedings of the IEEE/CVF
Conference on Computer Vision and Pattern Recogni-
tion, pages 13653–13662.
Smith, H. J., Zheng, Q., Li, Y., Jain, S., and Hodgins, J. K.
(2023). A method for animating children’s drawings
of the human figure. ACM Transactions on Graphics,
42(3):1–15.
Su, Q., Bai, X., Fu, H., Tai, C.-L., and Wang, J. (2018). Live
sketch: Video-driven dynamic deformation of static
drawings. In Proceedings of the 2018 chi conference
on human factors in computing systems, pages 1–12.
Tang, Z., Yang, Z., Zhu, C., Zeng, M., and Bansal, M.
(2024). Any-to-any generation via composable dif-
fusion. Advances in Neural Information Processing
Systems, 36.
Tanveer, M., Wang, Y., Wang, R., Zhao, N., Mahdavi-
Amiri, A., and Zhang, H. (2024). Anamodiff: 2d ana-
logical motion diffusion via disentangled denoising.
arXiv preprint arXiv:2402.03549.
Tao, J., Wang, B., Xu, B., Ge, T., Jiang, Y., Li, W., and
Duan, L. (2022). Structure-aware motion transfer
with deformable anchor model. In Proceedings of the
IEEE/CVF Conference on Computer Vision and Pat-
tern Recognition, pages 3637–3646.
Tian, Y., Ren, J., Chai, M., Olszewski, K., Peng, X.,
Metaxas, D. N., and Tulyakov, S. (2021). A good
image generator is what you need for high-resolution
video synthesis. arXiv preprint arXiv:2104.15069.
Wang, J., Xu, Y., Shum, H.-Y., and Cohen, M. F. (2004).
Video tooning. In ACM SIGGRAPH 2004 Papers,
pages 574–583.
Wang, J., Yuan, H., Chen, D., Zhang, Y., Wang, X., and
Zhang, S. (2023). Modelscope text-to-video technical
report. arXiv preprint arXiv:2308.06571.
Wang, Y., Yang, D., Bremond, F., and Dantcheva, A.
(2022). Latent image animator: Learning to animate
images via latent space navigation. arXiv preprint
arXiv:2203.09043.
Wu, C., Huang, L., Zhang, Q., Li, B., Ji, L., Yang, F.,
Sapiro, G., and Duan, N. (2021). Godiva: Generating
open-domain videos from natural descriptions. arXiv
preprint arXiv:2104.14806.
Xing, J., Wei, L.-Y., Shiratori, T., and Yatani, K. (2015).
Autocomplete hand-drawn animations. ACM Trans-
actions on Graphics (TOG), 34(6):1–11.
Xing, J., Xia, M., Zhang, Y., Chen, H., Wang, X., Wong,
T.-T., and Shan, Y. (2023). Dynamicrafter: Animat-
ing open-domain images with video diffusion priors.
arXiv preprint arXiv:2310.12190.
Xing, X., Wang, C., Zhou, H., Zhang, J., Yu, Q., and Xu,
D. (2024). Diffsketcher: Text guided vector sketch
synthesis through latent diffusion models. Advances
in Neural Information Processing Systems, 36.
Xu, Z., Zhou, Y., Kalogerakis, E., Landreth, C., and Singh,
K. (2020). Rignet: Neural rigging for articulated char-
acters. arXiv preprint arXiv:2005.00559.
Yan, W., Zhang, Y., Abbeel, P., and Srinivas, A. (2021).
Videogpt: Video generation using vq-vae and trans-
formers. arXiv preprint arXiv:2104.10157.
Zhao, J. and Zhang, H. (2022). Thin-plate spline motion
model for image animation. In Proceedings of the
IEEE/CVF Conference on Computer Vision and Pat-
tern Recognition, pages 3657–3666.
Zhou, D., Wang, W., Yan, H., Lv, W., Zhu, Y., and
Feng, J. (2022). Magicvideo: Efficient video gen-
eration with latent diffusion models. arXiv preprint
arXiv:2211.11018.
Zhu, J., Ma, H., Chen, J., and Yuan, J. (2023). Motion-
videogan: A novel video generator based on the mo-
tion space learned from image pairs. IEEE Transac-
tions on Multimedia.
GRAPP 2025 - 20th International Conference on Computer Graphics Theory and Applications
160