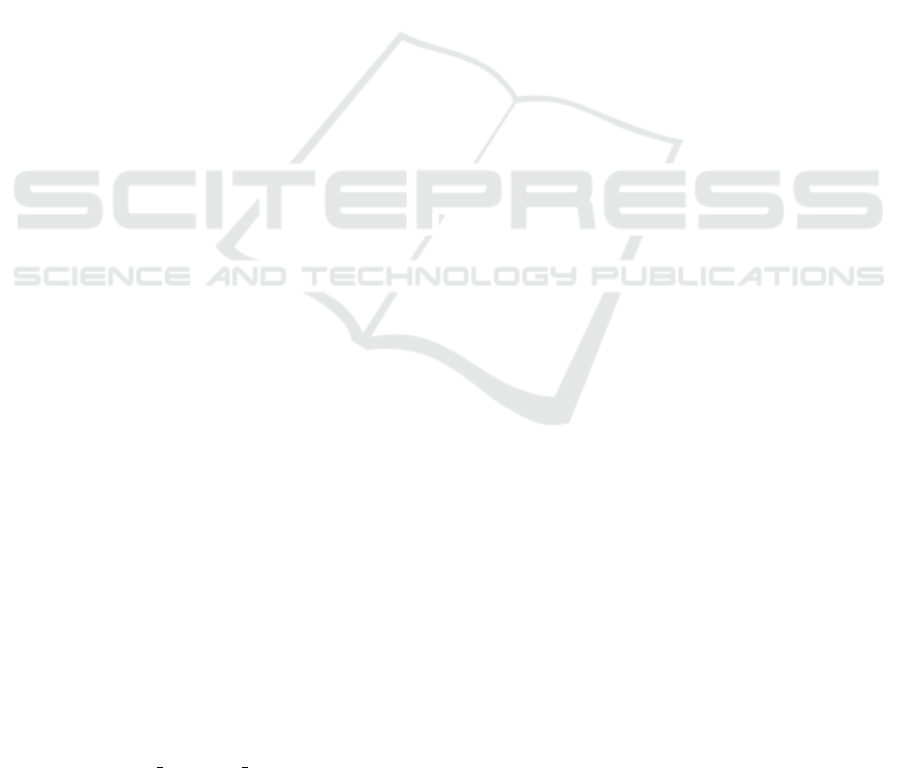
and IT Energy-aware Technologies, number 9, pages
24–32, Athens, Greece. IARIA XPS Press.
Ganin, Y., Ustinova, E., Ajakan, H., Germain, P.,
Larochelle, H., Laviolette, F., March, M., and Lem-
pitsky, V. (2016). Domain-adversarial training of neu-
ral networks. Journal of machine learning research,
17(59):1–35.
Haarnoja, T., Zhou, A., Abbeel, P., and Levine, S. (2018).
Soft actor-critic: Off-policy maximum entropy deep
reinforcement learning with a stochastic actor. In
International conference on machine learning, pages
1861–1870. PMLR.
Henderson, P., Islam, R., Bachman, P., Pineau, J., Precup,
D., and Meger, D. (2018). Deep reinforcement learn-
ing that matters. In Proceedings of the AAAI confer-
ence on artificial intelligence, volume 32.
Kumar, A., Zhou, A., Tucker, G., and Levine, S. (2020).
Conservative q-learning for offline reinforcement
learning. Advances in Neural Information Processing
Systems, 33:1179–1191.
Nair, A., McGrew, B., Andrychowicz, M., Zaremba, W.,
and Abbeel, P. (2018). Overcoming exploration in re-
inforcement learning with demonstrations. In 2018
IEEE international conference on robotics and au-
tomation (ICRA), pages 6292–6299. IEEE.
Nguyen, T. T., Nguyen, N. D., and Nahavandi, S. (2020).
Deep reinforcement learning for multiagent systems:
A review of challenges, solutions, and applications.
IEEE transactions on cybernetics, 50(9):3826–3839.
Omitaomu, O. A. and Niu, H. (2021). Artificial intelligence
techniques in smart grid: A survey. Smart Cities,
4(2):548–568.
Pan, S. J. and Yang, Q. (2010). A survey on transfer learn-
ing. IEEE Transactions on knowledge and data engi-
neering, 22(10):1345–1359.
Parisotto, E., Ba, J. L., and Salakhutdinov, R. (2015). Actor-
mimic: Deep multitask and transfer reinforcement
learning. arXiv preprint arXiv:1511.06342.
Tan, M., Tian, A., and Denoyer, L. (2022). Regularized
soft actor-critic for behavior transfer learning. In 2022
IEEE Conference on Games (CoG), pages 516–519.
IEEE.
Taylor, M. E. and Stone, P. (2009). Transfer learning for
reinforcement learning domains: A survey. Journal of
Machine Learning Research, 10(7).
Towers, M., Kwiatkowski, A., Terry, J., Balis, J. U.,
De Cola, G., Deleu, T., Goul
˜
ao, M., Kallinteris, A.,
Krimmel, M., KG, A., et al. (2024). Gymnasium: A
standard interface for reinforcement learning environ-
ments. arXiv preprint arXiv:2407.17032.
Veith, E. M., Logemann, T., Wellßow, A., and Balduin, S.
(2024). Play with me: Towards explaining the benefits
of autocurriculum training of learning agents. In 2024
IEEE PES Innovative Smart Grid Technologies Eu-
rope (ISGT EUROPE), pages 1–5, Dubrovnik, Croa-
tia. IEEE.
vwxyzjn (2024). sac continuous action.py. https://github.
com/vwxyzjn/cleanrl/blob/master/cleanrl. Accessed:
2024-05-28.
Weiss, K., Khoshgoftaar, T. M., and Wang, D. (2016).
A survey of transfer learning. Journal of Big data,
3(1):1–40.
Wolgast, T. and Nieße, A. (2024). Learning the opti-
mal power flow: Environment design matters. arXiv
preprint arXiv:2403.17831.
Xu, Z., Yang, D., Tang, J., Tang, Y., Yuan, T., Wang,
Y., and Xue, G. (2020). An actor-critic-based trans-
fer learning framework for experience-driven net-
working. IEEE/ACM Transactions on Networking,
29(1):360–371.
Zhu, Z., Lin, K., Jain, A. K., and Zhou, J. (2023). Trans-
fer learning in deep reinforcement learning: A survey.
IEEE Transactions on Pattern Analysis and Machine
Intelligence.
ICAART 2025 - 17th International Conference on Agents and Artificial Intelligence
692