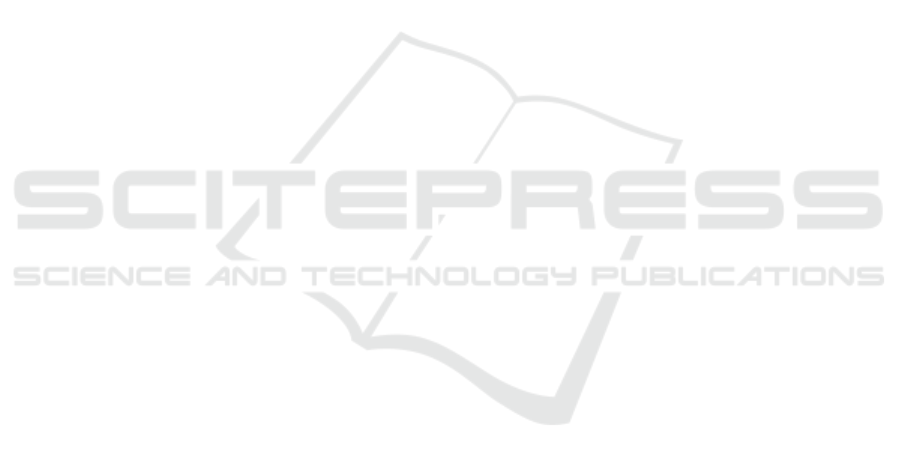
Kapadia, N., Gokhale, S., Nepomuceno, A., Cheng, W.,
Bothwell, S., Mathews, M., Shallat, J. S., Schultz, C.,
and Gupta, A. (2024). Evaluation of large language
model generated dialogues for an ai based vr nurse
training simulator. In Chen, J. Y. C. and Fragomeni,
G., editors, Virtual, Augmented and Mixed Reality,
pages 200–212, Cham. Springer Nature Switzerland.
Kern, A. C. and Ellermeier, W. (2020). Audio in vr: Effects
of a soundscape and movement-triggered step sounds
on presence. Frontiers in Robotics and AI, 7:20.
Klein, K., Sedlmair, M., and Schreiber, F. (2022). Immer-
sive analytics: An overview. it - Information Technol-
ogy, 64(4-5):155–168.
Kraus, M., Fuchs, J., Sommer, B., Klein, K., Engelke, U.,
Keim, D., and Schreiber, F. (2022). Immersive ana-
lytics with abstract 3d visualizations: A survey. Com-
puter Graphics Forum, 41(1):201–229.
Kraus, M., Klein, K., Fuchs, J., Keim, D. A., Schreiber,
F., and Sedlmair, M. (2021). The value of immersive
visualization. IEEE Computer Graphics and Applica-
tions, 41(4):125–132.
Le, M.-H., Chu, C.-B., Le, K.-D., Nguyen, T. V., Tran,
M.-T., and Le, T.-N. (2023). Vides: Virtual interior
design via natural language and visual guidance. In
2023 IEEE International Symposium on Mixed and
Augmented Reality Adjunct (ISMAR-Adjunct), pages
689–694.
Lee, J., Wang, J., Brown, E., Chu, L., Rodriguez, S. S., and
Froehlich, J. E. (2023). Towards designing a context-
aware multimodal voice assistant for pronoun disam-
biguation: A demonstration of gazepointar. In Adjunct
Proceedings of the 36th Annual ACM Symposium on
User Interface Software and Technology, UIST ’23
Adjunct, New York, NY, USA. Association for Com-
puting Machinery.
Lee, J., Wang, J., Brown, E., Chu, L. G. P., Rodriguez, S. S.,
and Froehlich, J. E. (2024). Gazepointar: A context-
aware multimodal voice assistant for pronoun disam-
biguation in wearable augmented reality. In Proceed-
ings of the 2024 CHI Conference on Human Factors
in Computing Systems.
Lewis, M., Liu, Y., Goyal, N., Ghazvininejad, M., Mo-
hamed, A., Levy, O., Stoyanov, V., and Zettlemoyer,
L. (2020). BART: Denoising sequence-to-sequence
pre-training for natural language generation, transla-
tion, and comprehension. In Jurafsky, D., Chai, J.,
Schluter, N., and Tetreault, J., editors, Proceedings of
the 58th Annual Meeting of the Association for Com-
putational Linguistics, pages 7871–7880, Online. As-
sociation for Computational Linguistics.
Li, Z., Babar, P. P., Barry, M., and Peiris, R. L. (2024). Ex-
ploring the use of large language model-driven chat-
bots in virtual reality to train autistic individuals in
job communication skills. In Extended Abstracts of
the CHI Conference on Human Factors in Computing
Systems, CHI EA ’24, New York, NY, USA. Associa-
tion for Computing Machinery.
Lo Duca., A. (2023). Towards a framework for ai-assisted
data storytelling. In Proceedings of the 19th Inter-
national Conference on Web Information Systems and
Technologies - WEBIST, pages 512–519. INSTICC,
SciTePress.
Lo Duca., A. (2024). Using retrieval augmented generation
to build the context for data-driven stories. In Pro-
ceedings of the 19th International Joint Conference
on Computer Vision, Imaging and Computer Graph-
ics Theory and Applications - IVAPP, pages 690–696.
INSTICC, SciTePress.
Marriott, K., Chen, J., Hlawatsch, M., Itoh, T., Nacenta,
M. A., Reina, G., and Stuerzlinger, W. (2018). Immer-
sive analytics: Time to reconsider the value of 3d for
information visualisation. Immersive analytics, pages
25–55.
McCormack, J., Roberts, J. C., Bach, B., Freitas, C. D. S.,
Itoh, T., Hurter, C., and Marriott, K. (2018). Multisen-
sory immersive analytics. Immersive analytics, pages
57–94.
Munzner, T. (2014). Visualization Analysis and Design. A
K Peters Visualization Series, CRC Press, 1;1st; edi-
tion.
Nath, M. and Ethirajan, L. (2023). Infographics genera-
tor: A smart application for visual summarization. In
2023 16th International Conference on Developments
in eSystems Engineering (DeSE), pages 630–635.
Numan, N., Giunchi, D., Congdon, B., and Steed, A.
(2023). Ubiq-genie: Leveraging external frameworks
for enhanced social vr experiences. In 2023 IEEE
Conference on Virtual Reality and 3D User Interfaces
Abstracts and Workshops (VRW), pages 497–501.
Pinargote, A., Calder
´
on, E., Cevallos, K., Carrillo, G.,
Chiluiza, K., and Echeverr
´
ıa, V. (2024). Automating
data narratives in learning analytics dashboards using
genai. In LAK Workshops, pages 150–161.
Rombach, R., Blattmann, A., Lorenz, D., Esser, P., and
Ommer, B. (2022). High-resolution image synthesis
with latent diffusion models. In Proceedings of the
IEEE/CVF conference on computer vision and pattern
recognition, pages 10684–10695.
Salehi, P., Hassan, S. Z., Baugerud, G. A., Powell, M.,
Cano, M. C. L., Johnson, M. S., Røed, R. K.,
Johansen, D., Sabet, S. S., Riegler, M. A., and
Halvorsen, P. (2024). Immersive virtual reality in
child interview skills training: A comparison of 2d
and 3d environments. In Proceedings of the 16th In-
ternational Workshop on Immersive Mixed and Virtual
Environment Systems, MMVE ’24, page 1–7, New
York, NY, USA. Association for Computing Machin-
ery.
Shao, H., Martinez-Maldonado, R., Echeverria, V., Yan, L.,
and Gasevic, D. (2024). Data storytelling in data visu-
alisation: Does it enhance the efficiency and effective-
ness of information retrieval and insights comprehen-
sion? In Proceedings of the 2024 CHI Conference on
Human Factors in Computing Systems, CHI ’24, New
York, NY, USA. Association for Computing Machin-
ery.
Shi, C., Yang, C., Liu, Y., Shui, B., Wang, J., Jing, M.,
Xu, L., Zhu, X., Li, S., Zhang, Y., Liu, G., Nie, X.,
Cai, D., and Yang, Y. (2024). Chartmimic: Evaluating
lmm’s cross-modal reasoning capability via chart-to-
code generation. arXiv preprint arXiv:2406.09961.
Generative Artificial Intelligence for Immersive Analytics
945