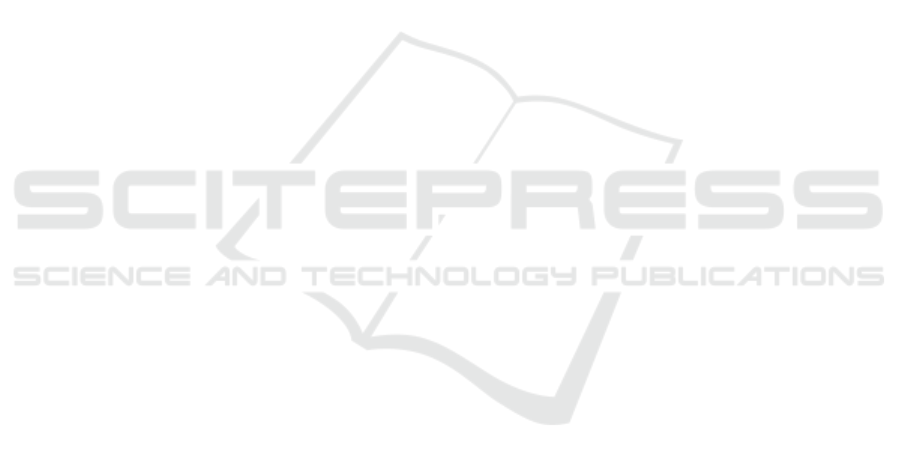
Light projection-induced illusion for controlling ob-
ject color. In 2018 IEEE Conference on Virtual Reality
and 3D User Interfaces (VR), pages 499–500. IEEE.
Amano, T., Komura, K., Sasabuchi, T., Nakano, S., and
Yamashita, S. (2012). Appearance control for human
material perception manipulation. In Proceedings of
the 21st international conference on pattern recogni-
tion (ICPR2012), pages 13–16. IEEE.
Berard, F. and Louis, T. (2017). The object inside: As-
sessing 3d examination with a spherical handheld
perspective-corrected display. In Proceedings of the
2017 CHI Conference on Human Factors in Comput-
ing Systems, pages 4396–4404.
Bertin, J. (1983). Semiology of graphics.
Bokaris, P.-A., Gouiff
`
es, M., Jacquemin, C., Chomaz, J.-
M., and Tr
´
emeau, A. (2015). One-frame delay for
dynamic photometric compensation in a projector-
camera system. In 2015 IEEE International Confer-
ence on Image Processing (ICIP), pages 2675–2679.
IEEE.
Douglas, D. B., Petricoin, E. F., Liotta, L., and Wilson, E.
(2016). D3d augmented reality imaging system: proof
of concept in mammography. Medical Devices: Evi-
dence and Research, pages 277–283.
Drebin, R. A., Carpenter, L., and Hanrahan, P. (1988). Vol-
ume rendering. ACM Siggraph Computer Graphics,
22(4):65–74.
Ensley, M. (1995). Toward a theory of situation awareness
in dynamic systems. Human factors, 37:85–104.
Gibson, E. J. and Pick, A. D. (2000). An ecological ap-
proach to perceptual learning and development. Ox-
ford University Press, USA.
Grundh
¨
ofer, A. and Bimber, O. (2006). Real-time adaptive
radiometric compensation. In ACM SIGGRAPH 2006
Research posters, pages 56–es.
Grundh
¨
ofer, A. and Iwai, D. (2015). Robust, error-tolerant
photometric projector compensation. IEEE Transac-
tions on Image Processing, 24(12):5086–5099.
Hurter, C., Taylor, R., Carpendale, S., and Telea, A. (2014).
Color tunneling: Interactive exploration and selection
in volumetric datasets. In 2014 IEEE Pacific Visual-
ization Symposium, pages 225–232. IEEE.
Iwai, D. and Sato, K. (2011). Document search support by
making physical documents transparent in projection-
based mixed reality. Virtual reality, 15:147–160.
Kindlmann, G. (2002). Transfer functions in direct vol-
ume rendering: Design, interface, interaction. Course
notes of ACM SIGGRAPH, 3.
Klein, G. (2008). Naturalistic decision making. Human
factors, 50(3):456–460.
Metz, C. E. and Doi, K. (1979). Transfer function analysis
of radiographic imaging systems. Physics in Medicine
& Biology, 24(6):1079.
Milgram, P. and Kishino, F. (1994). A taxonomy of mixed
reality visual displays. IEICE TRANSACTIONS on In-
formation and Systems, 77(12):1321–1329.
Mittelst
¨
adt, S., Stoffel, A., and Keim, D. A. (2014). Meth-
ods for compensating contrast effects in information
visualization. In Computer Graphics Forum, vol-
ume 33, pages 231–240. Wiley Online Library.
Mori, S., Ikeda, S., and Saito, H. (2017). A survey of di-
minished reality: Techniques for visually concealing,
eliminating, and seeing through real objects. IPSJ
Transactions on Computer Vision and Applications,
9:1–14.
Nishino, H., Hatano, E., Seo, S., Nitta, T., Saito, T., Naka-
mura, M., Hattori, K., Takatani, M., Fuji, H., Taura,
K., et al. (2018). Real-time navigation for liver surgery
using projection mapping with indocyanine green flu-
orescence: development of the novel medical imaging
projection system. Annals of surgery, 267(6):1134–
1140.
Park, J., Jung, D., and Moon, B. (2022). Projector com-
pensation framework using differentiable rendering.
IEEE Access, 10:44461–44470.
Post, M., Fieguth, P., Naiel, M. A., Azimifar, Z., and Lamm,
M. (2018). Fast radiometric compensation for nonlin-
ear projectors. Journal of Computational Vision and
Imaging Systems, 4(1):3–3.
Rieger, T., Heilmann, L., and Manzey, D. (2021). Visual
search behavior and performance in luggage screen-
ing: effects of time pressure, automation aid, and tar-
get expectancy. Cognitive Research: Principles and
Implications, 6:1–12.
Sharma, G., Wu, W., and Dalal, E. N. (2005). The
ciede2000 color-difference formula: Implementation
notes, supplementary test data, and mathematical ob-
servations. Color Research & Application, 30(1):21–
30.
Sheldon, M. R., Fillyaw, M. J., and Thompson, W. D.
(1996). The use and interpretation of the friedman test
in the analysis of ordinal-scale data in repeated mea-
sures designs. Physiotherapy Research International,
1(4):221–228.
Shepard, D. (1968). A two-dimensional interpolation func-
tion for irregularly-spaced data. In Proceedings of the
1968 23rd ACM national conference, pages 517–524.
Sutherland, J., Belec, J., Sheikh, A., Chepelev, L., Al-
thobaity, W., Chow, B. J., Mitsouras, D., Christensen,
A., Rybicki, F. J., and La Russa, D. J. (2019). Apply-
ing modern virtual and augmented reality technolo-
gies to medical images and models. Journal of digital
imaging, 32:38–53.
Traor
´
e, M., Hurter, C., and Telea, A. (2018). Interactive
obstruction-free lensing for volumetric data visualiza-
tion. IEEE transactions on visualization and computer
graphics, 25(1):1029–1039.
Treisman, A. M. and Gelade, G. (1980). A feature-
integration theory of attention. Cognitive psychology,
12(1):97–136.
Wang, X., Besanc¸on, L., Rousseau, D., Sereno, M., Ammi,
M., and Isenberg, T. (2020). Towards an understand-
ing of augmented reality extensions for existing 3d
data analysis tools. In Proceedings of the 2020 CHI
Conference on Human Factors in Computing Systems,
pages 1–13.
Yoshida, T., Horii, C., and Sato, K. (2003). A virtual color
reconstruction system for real heritage with light pro-
jection. In Proceedings of VSMM, volume 3, pages
1–7. Citeseer.
IVAPP 2025 - 16th International Conference on Information Visualization Theory and Applications
954