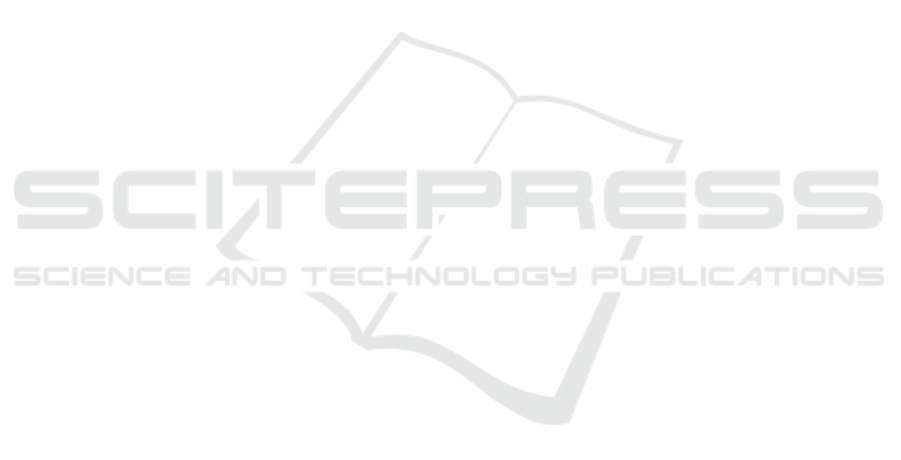
Conference on Computer Vision and Pattern Recogni-
tion (CVPR), pages 1534–1543.
Bian, C., Yuan, C., Wang, J., Li, M., Yang, X., and and,
S. Y. (2020). Uncertainty-aware domain alignment
for anatomical structure segmentation. Medical Im-
age Analysis, 64:101732.
Bischke, B., Helber, P., Borth, D., and Dengel, A. (2018).
Segmentation of imbalanced classes in satellite im-
agery using adaptive uncertainty weighted class loss.
In IGARSS 2018 - 2018 IEEE International Geo-
science and Remote Sensing Symposium, pages 6191–
6194.
Blundell, C., Cornebise, J., Kavukcuoglu, K., and Wierstra,
D. (2015). Weight uncertainty in neural networks.
Chang, A. X., Funkhouser, T., Guibas, L., Hanrahan, P.,
Huang, Q., Li, Z., Savarese, S., Savva, M., Song, S.,
Su, H., Xiao, J., Yi, L., and Yu, F. (2015). Shapenet:
An information-rich 3d model repository.
Chaudhury, A., Boudon, F., and Godin, C. (2020). 3d plant
phenotyping: All you need is labelled point cloud
data. In Workshop on Computer Vision Problems in
Plant Phenotyping, pages 244–260.
Gal, Y. and Ghahramani, Z. (2016). Dropout as a bayesian
approximation: Representing model uncertainty in
deep learning.
Ghahremani, M., Williams, K., Corke, F. M. K., Tiddeman,
B., Liu, Y., and Doonan, J. H. (2021). Deep segmen-
tation of point clouds of wheat. Frontiers in Plant
Science, 12.
Girardeau-Montaut, D. (2012). CloudCompare - Open
Source project — cloudcompare.org. https://www.
cloudcompare.org/. [Accessed 17-11-2023].
Haas, J. and Rabus, B. (2021). Uncertainty estimation
for deep learning-based segmentation of roads in syn-
thetic aperture radar imagery. Remote Sensing, 13(8).
Hu, H., Wang, F., Su, J., Zhou, H., Wang, Y., Hu, L., Zhang,
Y., and Zhang, Z. (2023). Gam : Gradient attention
module of optimization for point clouds analysis. In
AAAI Conference on Artificial Intelligence.
Kong, L., Kamarthi, H., Chen, P., Prakash, B. A., and
Zhang, C. (2023). Uncertainty quantification in deep
learning. In Proceedings of the 29th ACM SIGKDD
Conference on Knowledge Discovery and Data Min-
ing, KDD ’23, page 5809–5810, New York, NY, USA.
Association for Computing Machinery.
Landgraf, S., Wursthorn, K., Hillemann, M., and Ulrich,
M. (2024). Dudes: Deep uncertainty distillation using
ensembles for semantic segmentation. PFG – Journal
of Photogrammetry, Remote Sensing and Geoinforma-
tion Science, 92(2):101–114.
Leibig, C., Allken, V., Ayhan, M. S., Berens, P., and Wahl,
S. (2016). Leveraging uncertainty information from
deep neural networks for disease detection. Scientific
Reports, 7.
Li, D., Shi, G., Li, J., Chen, Y., Zhang, S., Xiang, S., and
Jin, S. (2022). Plantnet: A dual-function point cloud
segmentation network for multiple plant species. IS-
PRS Journal of Photogrammetry and Remote Sensing,
184:243–263.
Lin, Q., Chen, X., Chen, C., Pekaslan, D., and Garibaldi,
J. M. (2023). Fuzzy uncertainty-based out-of-
distribution detection algorithm for semantic segmen-
tation. In 2023 IEEE International Conference on
Fuzzy Systems (FUZZ), pages 1–6.
Minervini, M., Scharr, H., and Tsaftaris, S. (2015). Im-
age analysis: The new bottleneck in plant phenotyping
[applications corner]. IEEE Signal Processing Maga-
zine, 32:126–131.
Mochida, K., Koda, S., Inoue, K., Hirayama, T., Tanaka, S.,
Nishii, R., and Melgani, F. (2018). Computer vision-
based phenotyping for improvement of plant produc-
tivity: a machine learning perspective. GigaScience,
8(1):giy153.
Mukhoti, J., Kirsch, A., van Amersfoort, J. R., Torr, P. H. S.,
and Gal, Y. (2021). Deep deterministic uncertainty:
A new simple baseline. 2023 IEEE/CVF Conference
on Computer Vision and Pattern Recognition (CVPR),
pages 24384–24394.
Qi, C., Yi, L., Su, H., and Guibas, L. J. (2017). Pointnet++:
Deep hierarchical feature learning on point sets in a
metric space. In NIPS, page 5105–5114.
Qi, C. R., Su, H., Mo, K., and Guibas, L. J. (2016). Pointnet:
Deep learning on point sets for 3d classification and
segmentation. arXiv preprint arXiv:1612.00593.
Robbins, H. E. (1951). A stochastic approximation method.
Annals of Mathematical Statistics, 22:400–407.
Sch
¨
onberger, J. and Frahm, J.-M. (2016). Structure-from-
motion revisited.
Sch
¨
onberger, J., Zheng, E., Pollefeys, M., and Frahm, J.-
M. (2016). Pixelwise view selection for unstructured
multi-view stereo. volume 9907.
Shen, J., Wu, T., Zhao, J., Wu, Z., Huang, Y., Gao, P., and
Zhang, L. (2024). Organ segmentation and phenotypic
trait extraction of cotton seedling point clouds based
on a 3d lightweight network. Agronomy, 14(5).
Sirohi, K., Marvi, S., B
¨
uscher, D., and Burgard, W. (2022).
Uncertainty-aware panoptic segmentation.
Sun, Y., Lu, B., Liu, Y., Yang, Z., Behera, A., Song, R.,
Yuan, H., and Jiang, H. (2024). Exploiting label un-
certainty for enhanced 3d object detection from point
clouds. IEEE Transactions on Intelligent Transporta-
tion Systems, 25(6):6074–6089.
Tang, P., Yang, P., Nie, D., Wu, X., Zhou, J., and Wang,
Y. (2022). Unified medical image segmentation by
learning from uncertainty in an end-to-end manner.
Knowledge-Based Systems, 241:108215.
Turgut, K., Dutagaci, H., and Rousseau, D. (2022). Ros-
esegnet: An attention-based deep learning architec-
ture for organ segmentation of plants. Biosystems En-
gineering, 221:138–153.
Wang, J., Ancha, S., Chen, Y.-T., and Held, D. (2020).
Uncertainty-aware self-supervised 3d data associa-
tion. In 2020 IEEE/RSJ International Conference on
Intelligent Robots and Systems (IROS), pages 8125–
8132.
Zhou, W., Wang, Q., Jin, W., Shi, X., and He, Y. (2024).
Graph transformer for 3d point clouds classification
and semantic segmentation. Computers & Graphics,
124:104050.
Uncertainty and Feature-Based Weighted Loss for 3D Wheat Part Segmentation
641