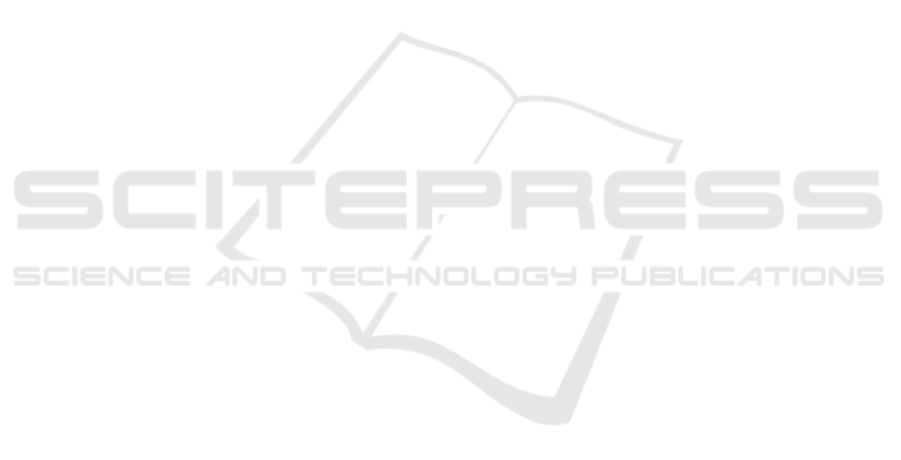
also established the fact that impacts of predictors on
the crime rates were in fact non linear and spatial.
(Mandalapu et al., 2023) conducted an extensive
review of various papers and found that traditional
clustering algorithms, such as K-Means, often fell
short in addressing the dynamic and noisy nature of
real-world crime data, particularly when temporal di-
mensions were involved. The maximum accuracy
these models could achieve was about 80%. Crime
incidents are influenced by both spatial proximity and
time intervals and thus deep learning models like
CNN or LSTM that simultaneously handle these di-
mensions performed significantly better. They found
the accuracy of such models reaching almost 95% de-
pending on the quality of the datasets.
(Marchant et al., 2018) noted that the Bayesian
framework improved criminal data analysis by using
a probabilistic model for capturing the dependencies
between crime rates and socio-environmental factors.
It also helped in incorporating the uncertainty asso-
ciated with predictions. It covered parametric and
non-parametric approaches, resulting in the capabil-
ity to model spatial dependencies adequately to fore-
cast crime rates. The authors considered investigation
of property crimes including theft, assault and drug
related offences and established that crime rates are
critically dependent on other demographic traits and
environmental features such as population density. In
conclusion, the Bayesian application was beneficial
for comprehensive and diverse crime analysis.
(Birant and Kut, 2007) proposed the ST-DBSCAN
algorithm which had the ability of discovering clus-
ters according to non-spatial, spatial and temporal val-
ues of the objects and was particularly effective for
processing very large datasets. They introduced the
novel concept of density factor which enabled the
algorithm to handle noisy data even when clusters
of different densities were present. It had a much
faster runtime (factor between 1.5 and 3 times) than
other clustering algorithms such as CLARANS (Ng
and Han, 1994) and DBCLASD (Xu et al., 1998) and
the factor only increased with the size of the datasets
used. Thus ST-DBSCAN became a strong candidate
for clustering using spatial–temporal data.
(Ramirez-Alcocer et al., 2019) demonstrated that
the use of Long Short-Term Memory (LSTM) net-
works delivered strong results for predicting future
crime hotspots as it was adept at handling sequential
data. The study showed the feasibility of employing
LSTM models trained on extensive datasets of his-
torical crime records. Their deep learning approach
achieved a high performance in the final model with
a validation accuracy of 87.84% and an average loss
function of 0.0376.
(Rai et al., 2022) demonstrated an effective ap-
proach by utilising LSTM in tandem with BERT, a
language model, to extract deeper contextual and lin-
guistic insights. The authors developed a model that
automatically classified news articles as either fake or
real based on their titles. This combination not only
enhanced the predictive accuracy to 88.75%, but also
enabled a more nuanced understanding of the textual
elements in the datasets.
Crime prediction involving the incorporation of
legal language models has become more popular re-
cently with different studies having researched on it.
(Paul et al., 2023) proposed InLegalBERT, inspired
by the work of (Beltagy et al., 2019) called SciBERT
that was pre-trained on scientific publications. InLe-
galBERT is a legal aligned BERT model pre-trained
on Indian legal documents. This study showed that
the proposed model could understand legal terms and
its context for the tasks relevant to the Indian legal
system such as of categorisation of crimes as per the
Indian Penal Code (IPC). The authors also noted that
warming on domain-specific texts improved the fine-
tuning results in legal NLP tasks substantially.
(Bogomolov et al., 2014) examined the correlation
between crime and demographic characteristics using
aggregated human behavioural data captured from the
mobile network infrastructure in combination with
basic demographic information. They achieved an ac-
curacy of almost 70% when predicting hotspots for
real crime data in London. This proved that using de-
mographic factors have the potential to help predict-
ing urban crime issues effectively.
(Fan et al., 2024) highlights the significance of
RAG in enhancing the capabilities of generative AI
by supplying reliable and up-to-date external knowl-
edge, which is particularly beneficial in the context of
AI-Generated Content (AIGC). The paper emphasises
the potential of raLLMs to mitigate common issues
faced by traditional LLMs, such as hallucinations and
outdated internal knowledge, by leveraging retrieval
mechanisms.
(VM et al., 2024) divides the process of fine tun-
ing into several stages. First training data in the target
domain was gathered and the text was then broken
into chunks and tokens with a suitable tokenizer to
convert the text into embeddings. The training cov-
ered the next token prediction strategy and optimised
the weights derived from the accumulated responses
given a trained-task oriented set of data set. The au-
thors highlighted that although the fine-tuning pro-
cess helped to improve the model, it raised a number
of issues including the availability and quality of the
data, costs and ethical issues, which are all critical and
should be discussed in detail.
ICAART 2025 - 17th International Conference on Agents and Artificial Intelligence
716