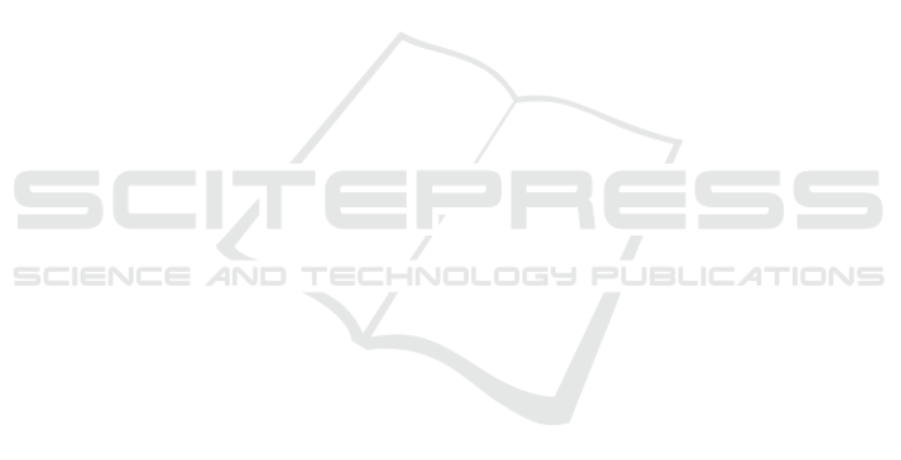
Herweg, N. A. and Kahana, M. (2018). Spatial representa-
tions in the human brain. Frontiers in Human Neuro-
science.
Honey, C. J., Thesen, T., Donner, T. H., Silbert, L. J., Carl-
son, C. E., Devinsky, O., Doyle, W. K., Rubin, N.,
Heeger, D. J., and Hasson, U. (2012). Slow cortical
dynamics and the accumulation of information over
long timescales. Neuron, 76(2):423–434.
Hromatka, B. S., Tung, J. Y., Kiefer, A. K., Do, C. B.,
Hinds, D. A., and Eriksson, N. (2015). Genetic vari-
ants associated with motion sickness point to roles
for inner ear development, neurological processes and
glucose homeostasis. Human Molecular Genetics,
24(9):2700–2708.
Hughes, M., Sulikowski, D., and Burke, D. (2014). Cor-
relations between spatial skills: A test of the hunter-
gatherer hypothesis. Journal of Evolutionary Psychol-
ogy, 12(1):19–44.
Irwin, J. (1952). The pathology of seasickness. The Lancet,
2:907–909.
Kaliuzhna, M., Prsa, M., and Blanke, O. (2015). Vestibu-
lar reweighting and multisensory integration in cyber-
sickness. PLoS ONE, 10:e0138563.
Kaplan, J., Ventura, J., Bakshi, A., Pierobon, A., Lackner,
J. R., and DiZio, P. (2017). The influence of sleep
deprivation and oscillating motion on sleepiness, mo-
tion sickness, and cognitive and motor performance.
Autonomic Neuroscience, 202:86–96.
Kobayashi, N., Iinuma, R., Suzuki, Y., Shimada, T., and
Ishikawa, M. (2015). Using bio-signals to evaluate
multi-discomfort in image viewing: Balancing visu-
ally induced motion sickness and field of view. In
37th Annual International Conference of the IEEE En-
gineering in Medicine and Biology Society (EMBC).
Koch, K. et al. (2006). How much the eye tells the brain.
Current Biology.
Kok, P., Mostert, P., and de Lange, F. P. (2017). Prior
expectations induce prestimulus sensory templates.
Proceedings of the National Academy of Sciences,
114(39):10473–10478.
Kumar, S. and Satsangi, P. (1992). System dynamics simu-
lation of hopfield neural networks. International Jour-
nal of Systems Science.
Laughlin, S. B. (2001). Energy as a constraint on the cod-
ing and processing of sensory information. Current
Opinion in Neurobiology, 11(4):475–480.
Lennie, P. (2003). The cost of cortical computation. Current
Biology.
Levy, W. B. and Calvert, V. G. (2021). Communication
consumes 35 times more energy than computation in
the human cortex.
Lin, J., Duh, H., and Parker, D. (2017). Effects of rest
frames on cybersickness in a virtual environment.
IEEE Transactions on Visualization and Computer
Graphics, 23(4):1255–1264.
Lin, J. J., Abi-Rached, H., Kim, D. H., Parker, D. E., and
Furness, T. A. (2002). A ’natural’ independent visual
background reduced simulator sickness. In Proceed-
ings of the Human Factors and Ergonomics Society
Annual Meeting.
Liu, C.-C. and Chen, C. (2011). Set based boltzmann ma-
chine and hopfield neural networks. NANO.
Lu, Y. (2020). Hippocampal spatial representation: Inte-
grating environmental and self-motion signals. Fron-
tiers in Cellular Neuroscience.
Ma, J., Zhang, Y., Lin, Y., and Dai, Z. (2021). Cost-
efficiency trade-offs of the human brain network re-
vealed by a multiobjective evolutionary algorithm.
Neuroimage, 236.
Makransky, G., Terkildsen, T. S., and Mayer, R. E. (2019).
Adding immersive virtual reality to a science lab sim-
ulation causes more presence but less learning. Learn-
ing and Instruction, 60:225–236.
Matsangas, P. and McCauley, M. E. (2014). Yawning as
a behavioral marker of mild motion sickness and so-
pite syndrome. Aviation, Space, and Environmental
Medicine, 85(6).
Monaco, J. e. a. (2019). Spatial synchronization codes from
coupled rate-phase neurons. PLOS Computational Bi-
ology.
Monteiro, D., Liang, H.-N., Abel, A., Baghaei, N., and
Monteiro, R. d. C. (2018a). Evaluating engagement
of virtual reality games based on first and third per-
son perspective using eeg and subjective metrics. In
2018 IEEE International Conference on Artificial In-
telligence and Virtual Reality (AIVR), pages 53–60.
Monteiro, D., Liang, H.-N., Tang, X., and Irani, P. (2021).
Using trajectory compression rate to predict changes
in cybersickness in virtual reality games. In 2021
IEEE International Symposium on Mixed and Aug-
mented Reality (ISMAR), pages 138–146.
Monteiro, D., Liang, H.-N., Wang, J., Chen, H., and
Baghaei, N. (2020). An in-depth exploration of the ef-
fect of 2d/3d views and controller types on first person
shooter games in virtual reality. In 2020 IEEE Inter-
national Symposium on Mixed and Augmented Reality
(ISMAR), pages 713–724.
Monteiro, D., Liang, H.-N., Zhao, Y., and Abel, A. (2018b).
Comparing event related arousal-valence and focus
among different viewing perspectives in vr gaming. In
Advances in Brain Inspired Cognitive Systems. BICS
2018. Lecture Notes in Computer Science, volume
10989. Springer, Cham.
Monteiro, D., Wang, A., Wang, L., et al. (2024). Effects
of audience familiarity on anxiety in a virtual reality
public speaking training tool. Universal Access in the
Information Society, 23:23–34.
Murthy, G. R. and Gabbouj, M. (2015). On the design of
hopfield neural networks: Synthesis of hopfield type
associative memories. IJCNN.
Nalivaiko, E., Gavgani, A. M., and Walker, F. R. (2018).
Cybersickness-related changes in brain hemodynam-
ics: A pilot study comparing transcranial doppler and
near-infrared spectroscopy assessments during a vir-
tual ride on a roller coaster. Physiology & Behavior,
191:56–64.
Nalivaiko, E., Rudd, J., and So, R. (2004). Motion sick-
ness, nausea, and temperature changes: A hypothesis.
Autonomic Neuroscience, 116:77–82.
The Exhausted Brain Theory: An Energy-Based Framework for Understanding Visually Induced Motion Sickness
637