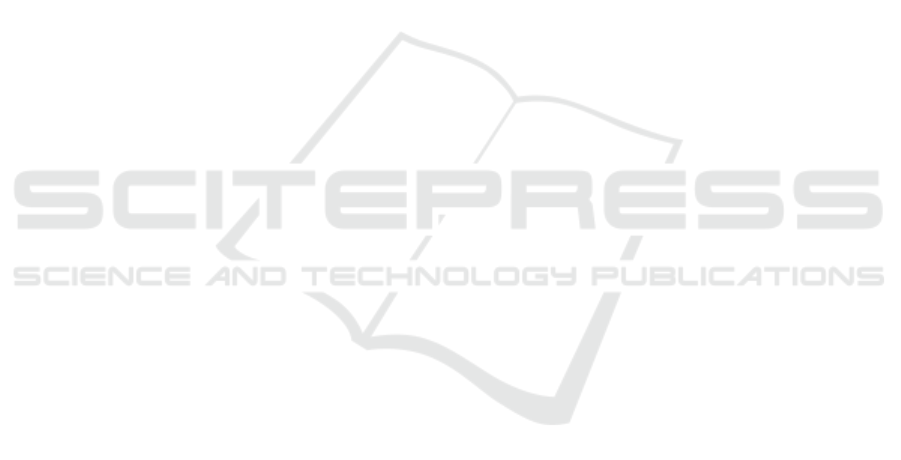
Fowler, C., Doyle, M. J., and Manzke, M. (2014). Adap-
tive bvh: an evaluation of an efficient shared data
structure for interactive simulation. In Proceedings of
the 30th Spring Conference on Computer Graphics,
SCCG ’14, page 37–45, New York, NY, USA. Asso-
ciation for Computing Machinery.
Fuetterling, V., Lojewski, C., Pfreundt, F.-J., and Ebert, A.
(2016). Parallel spatial splits in bounding volume hi-
erarchies. In Proceedings of the 16th Eurographics
Symposium on Parallel Graphics and Visualization,
EGPGV ’16, page 21–30, Goslar, DEU. Eurograph-
ics Association.
Ganestam, P., Barringer, R., Doggett, M., and Akenine-
M
¨
oller, T. (2015). Bonsai: Rapid bounding volume hi-
erarchy generation using mini trees. Journal of Com-
puter Graphics Techniques (JCGT), 4(3):23–42.
Ganestam, P. and Doggett, M. (2016). Sah guided spatial
split partitioning for fast bvh construction. Computer
Graphics Forum, 35(2):285–293.
Goldsmith, J. and Salmon, J. (1987). Automatic creation
of object hierarchies for ray tracing. IEEE Computer
Graphics and Applications, 7(5):14–20.
Karras, T. (2012). Maximizing parallelism in the construc-
tion of bvhs, octrees, and k-d trees. In Proceed-
ings of the Fourth ACM SIGGRAPH / Eurographics
Conference on High-Performance Graphics, EGGH-
HPG’12, page 33–37, Goslar, DEU. Eurographics As-
sociation.
Lauterbach, C., Garland, M., Sengupta, S., Luebke, D., and
Manocha, D. (2009). Fast bvh construction on gpus.
Computer Graphics Forum, 28(2):375–384.
MacDonald, J. D. and Booth, K. S. (1990). Heuristics for
ray tracing using space subdivision. The Visual Com-
puter, 6:153–166.
Meagher, D. (1980). Octree encoding: A new technique for
the representation, manipulation and display of arbi-
trary 3-d objects by computer. Technical report, IPL:
Image Processing Laboratory, Electrical and Systems
Engineering Department, Rensselaer Polytechnic In-
stitute, Troy, New York 12181.
Meister, D. and Bittner, J. (2018). Parallel reinsertion for
bounding volume hierarchy optimization. Computer
Graphics Forum, 37(2):463–473.
Meister, D., Ogaki, S., Benthin, C., Doyle, M. J., Guthe, M.,
and Bittner, J. (2021). A Survey on Bounding Volume
Hierarchies for Ray Tracing. Computer Graphics Fo-
rum.
Pantaleoni, J. and Luebke, D. (2010). Hlbvh: hierarchical
lbvh construction for real-time ray tracing of dynamic
geometry. In Proceedings of the Conference on High
Performance Graphics, HPG ’10, page 87–95, Goslar,
DEU. Eurographics Association.
Pharr, M. (2018). Guest editor’s introduction: Special issue
on production rendering. ACM Trans. Graph., 37(3).
Stich, M., Friedrich, H., and Dietrich, A. (2009). Spatial
splits in bounding volume hierarchies. In Proceed-
ings of the Conference on High Performance Graph-
ics 2009, HPG ’09, page 7–13, New York, NY, USA.
Association for Computing Machinery.
Usha, R., Pandey, P., and Mangala, N. (2020). A com-
prehensive comparison and analysis of openacc and
openmp 4.5 for nvidia gpus. In 2020 IEEE High Per-
formance Extreme Computing Conference (HPEC),
pages 1–6.
Wald, I. (2007). On fast construction of sah-based bound-
ing volume hierarchies. In 2007 IEEE Symposium on
Interactive Ray Tracing, pages 33–40.
Wald, I. (2012). Fast construction of sah bvhs on the in-
tel many integrated core (mic) architecture. IEEE
Transactions on Visualization and Computer Graph-
ics, 18(1):47–57.
Wald, I., Woop, S., Benthin, C., Johnson, G. S., and Ernst,
M. (2014). Embree: a kernel framework for efficient
cpu ray tracing. ACM Trans. Graph., 33(4).
GRAPP 2025 - 20th International Conference on Computer Graphics Theory and Applications
354