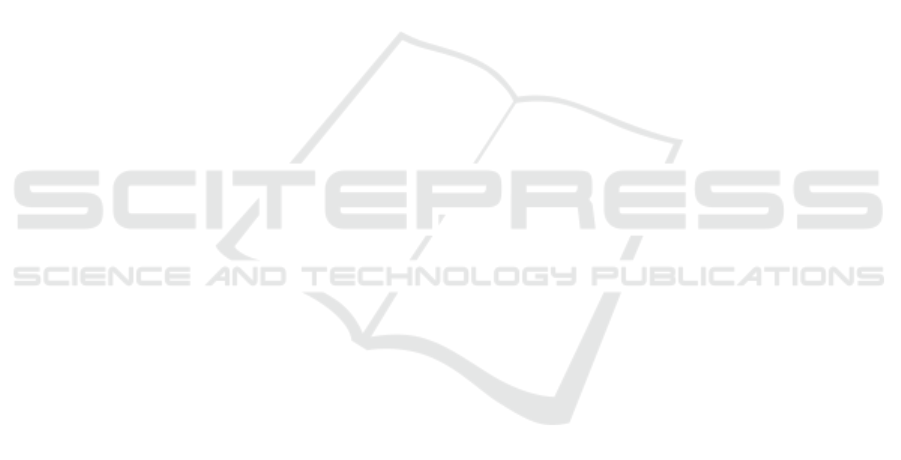
more than other domains, such as general natural lan-
guage processing, where large, well-curated datasets
are available.
As such, developing machine learning applica-
tions for healthcare needs more consideration. De-
veloping a machine learning model that makes cor-
rect predictions for one dataset might not be enough
to build general real-world applications. Overall, to
ensure the development and integration of machine
learning into healthcare applications, more collabo-
ration, more standards, and more data collection are
needed.
ACKNOWLEDGMENT
This research is funded by the German Federal Min-
istry of Education and Research (BMBF) under the
grant numbers 16KISR003 and 13GW0598C and by
the Susanne Bunnenberg Heart Foundation. We are
grateful for the funding received.
REFERENCES
Al-Zaiti, S., Martin-Gill, C., Zègre-Hemsey, J., Bouzid, Z.,
Faramand, Z., Alrawashdeh, M., Gregg, R., Helman,
S., Riek, N., Kraevsky-Phillips, K., Clermont, G., Ak-
cakaya, M., Sereika, S., Dam, P., Smith, S., Birnbaum,
Y., Saba, S., Sejdic, E., and Callaway, C. (2023). Ma-
chine learning for ecg diagnosis and risk stratification
of occlusion myocardial infarction. Nature Medicine,
29:1–10.
Alaa, A. M., Bolton, T., Di Angelantonio, E., Rudd, J. H. F.,
and van der Schaar, M. (2019). Cardiovascular disease
risk prediction using automated machine learning: A
prospective study of 423,604 uk biobank participants.
PLOS ONE, 14(5):1–17.
Chekroud, A. M., Hawrilenko, M., Loho, H., Bondar,
J., Gueorguieva, R., Hasan, A., Kambeitz, J., Cor-
lett, P. R., Koutsouleris, N., Krumholz, H. M., Krys-
tal, J. H., and Paulus, M. (2024). Illusory gener-
alizability of clinical prediction models. Science,
383(6679):164–167.
Dexter, G. P., Grannis, S. J., Dixon, B. E., and Kasthuri-
rathne, S. N. (2020). Generalization of Machine
Learning Approaches to Identify Notifiable Condi-
tions from a Statewide Health Information Exchange.
AMIA Joint Summits on Translational Science pro-
ceedings. AMIA Joint Summits on Translational Sci-
ence, 2020:152–161.
Johnson, A., Pollard, T., and Mark, R. (2023). Mimic-iii
clinical database.
Kumari, J., Kumar, E., and Kumar, D. (2023). A structured
analysis to study the role of machine learning and deep
learning in the healthcare sector with big data analyt-
ics. Archives of Computational Methods in Engineer-
ing, 30(6):3673–3701.
Lin, Y.-W., Zhou, Y., Faghri, F., Shaw, M. J., and Campbell,
R. H. (2019). Analysis and prediction of unplanned
intensive care unit readmission using recurrent neu-
ral networks with long short-term memory. PloS one,
14(7):e0218942.
Massey, F. J. (1951). The kolmogorov-smirnov test for
goodness of fit. Journal of the American Statistical
Association, 46(253):68–78.
Moazemi, S., Kalkhoff, S., Kessler, S., Boztoprak, Z., Het-
tlich, V., Liebrecht, A., Bibo, R., Dewitz, B., Licht-
enberg, A., Aubin, H., et al. (2022). Evaluating a
recurrent neural network model for predicting read-
mission to cardiovascular icus based on clinical time
series data. Engineering Proceedings, 18(1):1.
Müller, M. (2007). Dynamic time warping. Information
retrieval for music and motion, pages 69–84.
Panch, T., Mattie, H., and Celi, L. A. (2019). The “in-
convenient truth” about ai in healthcare. NPJ digital
medicine, 2(1):1–3.
Shapiro, D., Lee, K., Asmussen, J., Bourquard, T., and
Lichtarge, O. (2023). Evolutionary action–machine
learning model identifies candidate genes associated
with early-onset coronary artery disease. Journal of
the American Heart Association, 12(17):e029103.
Tonneau, M., Phan, K., Manem, V. S. K., Low-Kam, C.,
Dutil, F., Kazandjian, S., Vanderweyen, D., Panasci,
J., Malo, J., Coulombe, F., Gagné, A., Elkrief, A.,
Belkaïd, W., Di Jorio, L., Orain, M., Bouchard, N.,
Muanza, T., Rybicki, F. J., Kafi, K., Huntsman, D.,
Joubert, P., Chandelier, F., and Routy, B. (2023). Gen-
eralization optimizing machine learning to improve
CT scan radiomics and assess immune checkpoint
inhibitors’ response in non-small cell lung cancer:
a multicenter cohort study. Frontiers in Oncology,
13:1196414.
WELCH, B. L. (1947). The generalization of ‘student’s’
problem when several different population varlances
are involved. Biometrika, 34(1-2):28–35.
Wells, B. J., Chagin, K. M., Nowacki, A. S., and Kattan,
M. W. (2013). Strategies for Handling Missing Data
in Electronic Health Record Derived Data. eGEMs,
1(3):1035.
HEALTHINF 2025 - 18th International Conference on Health Informatics
260