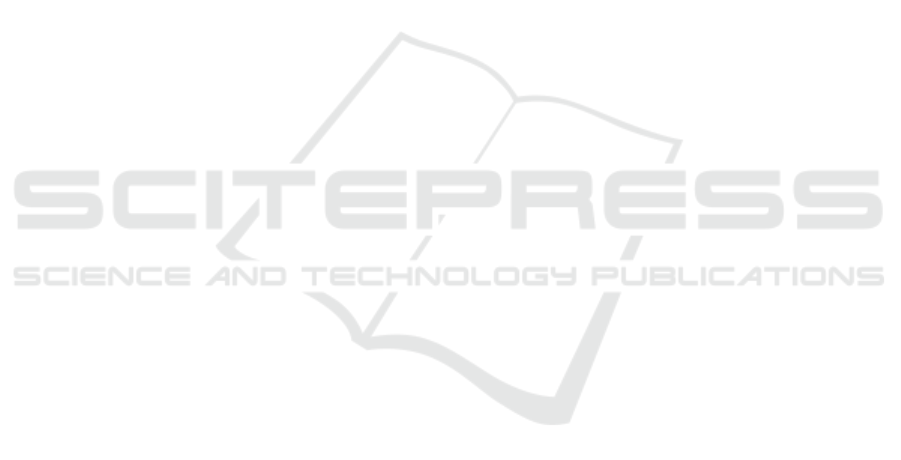
flow. Specifically, we propose a method to automat-
ically generate rotoscope animation by creating line
drawings from live-action footage and separating ma-
terials while identifying line art expressions and col-
oring regions to facilitate incorporation into produc-
tion. In the proposed system, rotoscope animation is
generated through the following steps:
1. Line drawing creation using SAM
2. Creating shadow regions by reducing colors in ba-
sic coloring areas
3. Finishing (coloring) and compositing
The reason for identifying coloring regions is that
generating only line drawings would require coloring
work for each frame. By identifying coloring regions
beforehand, colors can be applied to the entire anima-
tion at once. In anime production, cels are colored one
by one in the finishing process while referring to color
design, which specifies coloring instructions. In this
research, if color design is available, the finishing pro-
cess can be completed immediately. Afterwards, in
the shooting process that composites multiple materi-
als to create the final image or video, cels and back-
grounds are composited and the screen is adjusted.
It is important to emphasize here that the proposed
technology is not a mere style conversion method like
Diffutoon (Duan et al., 2024) or DomoAI (DOMOAI
PTE. LTD, 2024), but can also output intermediate
data such as line drawings in accordance with the ani-
mation production process. The existence of interme-
diate data allows retakes and re-editing, thus replacing
part of the existing animation production pipeline.
2 RELATED WORKS
We propose a novel automated rotoscoping method
that automatically generates line drawings and col-
oring regions suitable for anime production. In this
section, we classify related existing research from the
following three perspectives and analyze their advan-
tages and disadvantages. Then, we clarify the posi-
tioning and novelty of this research.
2.1 Conventional Rotoscoping Methods
Conventional rotoscoping has primarily been per-
formed through manual line tracing. While line
drawing extraction using edge detection like Canny
method (Canny, 1986) has been studied, it faces chal-
lenges in generating closed regions necessary for col-
oring and cannot reproduce anime-specific line art
expressions. Agarwala et al. proposed efficiency
improvements through keyframe interpolation (Agar-
wala et al., 2004), but the manual workload re-
mains substantial. Adobe After Effects’ Roto Brush
tool specializes in silhouette extraction (Dissanayake
et al., 2021; Torrejon et al., 2020) but is not suited for
hierarchical generation of line drawings and coloring
regions needed in anime production.
2.2 Image Anime-Stylization Using
Deep Learning
GAN-based methods like CartoonGAN (Chen et al.,
2018) and AnimeGAN (Chen et al., 2020), and Sta-
ble Diffusion-based methods (Rombach et al., 2022;
Esser et al., 2024) can generate high-quality anime-
style images. Nevertheless, these methods are not
suitable for animation production as they cannot
consider temporal coherence and shape consistency.
Among these methods, the latest stylization tech-
niques are Diffutoon (Duan et al., 2024) and Do-
moAI (DOMOAI PTE. LTD, 2024). These maintain
general temporal consistency and demonstrate high
quality as video generation AI. However, they can-
not separately output line drawings, coloring regions,
and shooting process effects, making integration into
commercial anime production workflows difficult.
2.3 Segmentation Technology and Its
Application to Anime
As emphasized by animator Tatsuyuki Tanaka, anime
expression consists of “symbolic expressions of sim-
ple lines and color separation” (Tanaka, 2021), unlike
realistic paintings. To capture these symbolic expres-
sions, segmentation at the semantic level becomes
crucial. In recent years, deep learning-based seg-
mentation technology has rapidly advanced, enabling
high-precision segmentation. The Segment Anything
Model (SAM) (Kirillov et al., 2023) is a prime ex-
ample, being a versatile model capable of accurately
segmenting various objects at the pixel level.
In Tous’s research (Tous, 2024), they use SAM
to segment various visual features of characters (hair,
skin, clothes, etc.) and combine it with a method
called DeAOT to automatically generate retro-style
rotoscope animations. However, it specializes in
styles composed of limited colors and expressions
like retro games, and since it does not consider gen-
eral anime production or line drawing generation, it
is not suitable for delicate expressions like Japanese
anime created in a line-expression culture.
As shown in Table 1, existing methods do not ad-
equately consider the hierarchical generation of line
drawings and coloring regions necessary for anime
GRAPP 2025 - 20th International Conference on Computer Graphics Theory and Applications
364