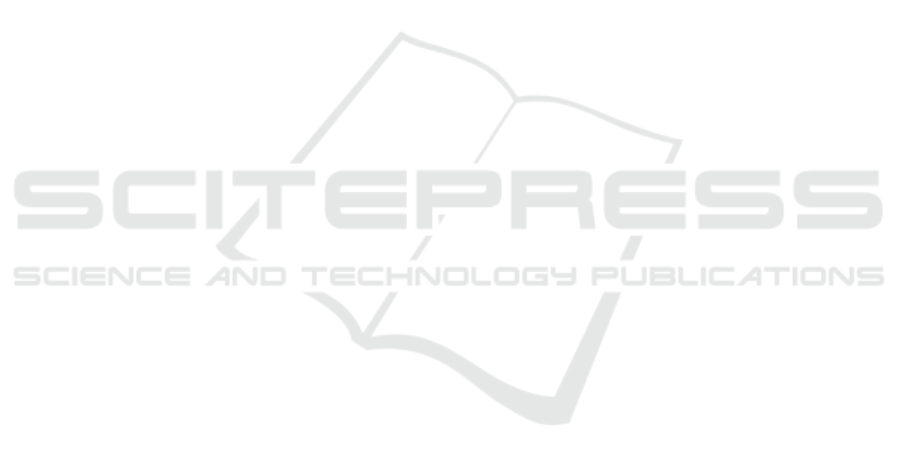
REFERENCES
Birawo, B. and Kasprowski, P. (2022). Review and eval-
uation of eye movement event detection algorithms.
Sensors.
Blanke, O. and Seeck, M. (2003). Direction of saccadic and
smooth eye movements induced by electrical stimu-
lation of the human frontal eye field: effect of orbital
position. Experimental Brain Research, 150:174–183.
Brien, D. C. et al. (2023). Classification and staging of
parkinson’s disease using video-based eye tracking.
Parkinsonism & Related Disorders, 110:105316.
Dar, A. H., Wagner, A. S., and Hanke, M. (2021). Remod-
nav: robust eye-movement classification for dynamic
stimulation. Behavior Research Methods.
de Villers-Sidani, E. et al. (2023). A novel tablet-based
software for the acquisition and analysis of gaze and
eye movement parameters: a preliminary validation
study in parkinson’s disease. Frontiers in Neurology,
14:1204733.
Eivazi, S., Santini, T., Keshavarzi, A., K
¨
ubler, T., and
Mazzei, A. (2019). Improving real-time cnn-based
pupil detection through domain-specific data augmen-
tation. In Proceedings of the 2019 Symposium on
Eye Tracking Research and Applications (ETRA ’19),
page 6, Denver, CO, USA. ACM.
Fawaz, H. I., Forestier, G., Weber, J., et al. (2019). Deep
learning for time series classification: a review. Data
Mining and Knowledge Discovery.
Frei, K. (2020). Abnormalities of smooth pursuit in parkin-
son’s disease: A systematic review. Clinical Parkin-
sonism & Related Disorders, 4:100085.
Haslwanter, T. and Clarke, A. H. (2010). Chapter 5—eye
movement measurement: Electro-oculography and
video-oculography. In Elsevier, editor, Vertigo and
Imbalance: Clinical Neurophysiology of the Vestibu-
lar System, volume 9, pages 61–79. Amsterdam, The
Netherlands.
Hatami, N., Gavet, Y., and Debayle, J. (2017). Classifi-
cation of time-series images using deep convolutional
neural networks. In Tenth International Conference
on Machine Vision (ICMV 2017), volume 10696, page
106960Y. International Society for Optics and Photon-
ics.
Hindle, J. V. (2010). Ageing, neurodegeneration and
parkinson’s disease. Age and Ageing, 39(2):156–161.
Jiang, M., Liu, Y., Cao, Y., Liu, Y., Wang, J., Li, P., Xia, S.,
et al. (2024). Auxiliary diagnostic method of parkin-
son’s disease based on eye movement analysis in a vir-
tual reality environment. Neuroscience Letters.
Koch, N. A., Voss, P., Cisneros-Franco, J. M., et al. (2024).
Eye movement function captured via an electronic
tablet informs on cognition and disease severity in
parkinson’s disease. Scientific Reports, 14:9082.
Lal, V. and Truong, D. (2019). Eye movement abnormalities
in movement disorders, volume 1, pages 54–63.
Li, H., Zhang, X., Yang, Y., and Xie, A. (2023). Abnormal
eye movements in parkinson’s disease: From experi-
mental study to clinical application. In Parkinsonism
& Related Disorders. Elsevier.
Ma, W., Li, M., Wu, J., Zhang, Z., Jia, F., Zhang, M.,
Bergman, H., Li, X., Ling, Z., and Xu, X. (2022).
Multiple step saccades in simply reactive saccades
could serve as a complementary biomarker for the
early diagnosis of parkinson’s disease. Frontiers in
Aging Neuroscience, 14:912967.
M
¨
uller, M. (2007). Dynamic time warping. Springer.
Pretegiani, E. and Optican, L. M. (2017). Eye movements
in parkinson’s disease and inherited parkinsonian syn-
dromes. Frontiers in Neurology, 8:592.
Przybyszewski, A. W.,
´
Sledzianowski, A., Chudzik, A.,
Szlufik, S., and Koziorowski, D. (2023). Ma-
chine learning and eye movements give insights into
neurodegenerative disease mechanisms. Sensors,
23:2145.
Quan, S., Sun, M., Zeng, X., Wang, X., and Zhu, Z. (2023).
Time series classification based on multi-dimensional
feature fusion. IEEE Access.
Rascol, O. et al. (1989). Abnormal ocular movements
in parkinson’s disease: Evidence for involvement of
dopaminergic systems. Brain, 112:1193–121.
Senin, P. (2008). Dynamic time warping algorithm review.
In Department of Information and Computer Science.
ResearchGate.
Sirocchi, C., Bogliolo, A., and Montagna, S. (2024).
Medical-informed machine learning: integrating prior
knowledge into medical decision systems. BMC Med-
ical Informatics and Decision Making.
Tinelli, M., Kanavos, P., and Grimaccia, F. (2016). The
value of early diagnosis and treatment in Parkinson’s
disease: A literature review of the potential clinical
and socioeconomic impact of targeting unmet needs
in Parkinson’s disease. London.
Tolosa, E., Garrido, A., Scholz, S. W., and Poewe, W.
(2021). Challenges in the diagnosis of parkinson’s dis-
ease. Lancet Neurology, 20:385–397.
Turcano, P., Chen, J. J., Bureau, B. L., and Savica, R.
(2019). Early ophthalmologic features of parkinson’s
disease: a review of preceding clinical and diagnostic
markers. Journal of Neurology, 266:2103–2111.
Wang, Z. and Oates, T. (2015). Encoding time series as
images for visual inspection and classification using
tiled convolutional neural networks. In Workshops at
the Twenty-Ninth AAAI Conference on Artificial Intel-
ligence. cdn.aaai.org.
White, O. B., Saint-Cyr, J. A., Tomlinson, R. D., and
Sharpe, J. A. (1983). Ocular motor deficits in parkin-
son’s disease. Brain, 106:571–587.
Yang, Z. Y., Zhang, Y., and Yu, L. N. (2024). Predicting
bank users’ time deposits based on lstm-stacked mod-
eling. Acadlore Transactions on Machine Learning.
Zhang, J., Zhang, B., Ren, Q., et al. (2021). Eye movement
especially vertical oculomotor impairment as an aid
to assess parkinson’s disease. Neurological Sciences,
42:2337–2345.
S¸ tef
˘
anescu, E., Chelaru, V. F., Chira, D., and Mures¸anu,
D. (2024). Eye tracking assessment of parkinson’s
disease: a clinical retrospective analysis. Journal of
Medicine and Life.
HEALTHINF 2025 - 18th International Conference on Health Informatics
272