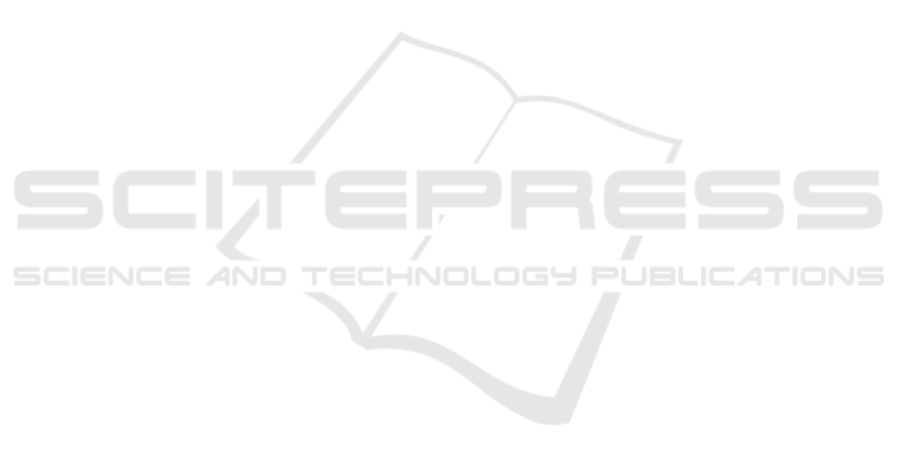
Cerezo, R., Bogar
´
ın, A., Esteban, M., and Romero, C.
(2020). Process mining for self-regulated learning
assessment in e-learning. Journal of Computing in
Higher Edu., 32(1):74–88.
Cole, J. and Foster, H. (2007). Using Moodle: Teaching
with the popular open source course management sys-
tem. ”O’Reilly Media, Inc.”.
Costa, J., Azevedo, A., and Rodrigues, L. (2020). Edu-
cational process mining based on moodle courses: a
review of literature. In 20th Conf. of the Portuguese
Association for Information Systems (CAPSI).
Etinger, D., Orehovacki, T., and Babic, S. (2018). Ap-
plying process mining techniques to learning manage-
ment systems for educational process model discovery
and analysis. In Intelligent Human Systems Integra-
tion (IHSI 2018), pages 420–425. Springer.
Fastiggi, W. (2019). Applying bloom’s taxonomy to the
classroom. Technology for Learners.
Ga
ˇ
sevi
´
c, D., Dawson, S., and Siemens, G. (2015). Let’s
not forget: Learning analytics are about learning.
TechTrends, 59:64–71.
Joudieh, N., Eteokleous, N., Champagnat, R., Rabah, M.,
and Nowakowski, S. (2023). Employing a process
mining approach to recommend personalized adap-
tive learning paths in blended-learning environments.
In 12th Int. Conf. in Open and Distance Learning,
Athens, Greece, volume 12.
Joudieh, N., Trabelsi, M., Champagnat, R., Rabah, M., and
Eteokleous, N. (2024). Using trace clustering to group
learning scenarios: An adaptation of fss-encoding to
moodle logs use case. In Proceedings of the 16th Int.
Conf. on Computer Supported Edu., pages 247–254.
Juha
ˇ
n
´
ak, L., Zounek, J., and Rohl
´
ıkov
´
a, L. (2019). Us-
ing process mining to analyze students’ quiz-taking
behavior patterns in a learning management system.
Computers in Human Behavior, 92:496–506.
Li, T., Fan, Y., Srivastava, N., Zeng, Z., Li, X., Khosravi, H.,
Tsai, Y.-S., Swiecki, Z., and Ga
ˇ
sevi
´
c, D. (2024). Ana-
lytics of planning behaviours in self-regulated learn-
ing: Links with strategy use and prior knowledge.
In Proceedings of the 14th Learning Analytics and
Knowledge Conf., page 438–449.
Maldonado, J., P
´
erez-Sanagust
´
ın, M., Kizilcec, R. F.,
Morales, N., and Munoz-Gama, J. (2018). Mining
theory-based patterns from big data: Identifying self-
regulated learning strategies in massive open online
courses. Computers in Human Behavior, 80:179–196.
Nammakhunt, A., Porouhan, P., and Premchaiswadi, W.
(2023). Creating and collecting e-learning event logs
to analyze learning behavior of students through pro-
cess mining. Int. Journal of Information and Edu.
Technology, 13(2):211–222.
Osakwe, I., Chen, G., Fan, Y., Rakovic, M., Singh, S.,
Molenaar, I., and Ga
ˇ
sevi
´
c, D. (2024). Measurement of
self-regulated learning: Strategies for mapping trace
data to learning processes and downstream analysis
implications. In Proceedings of the 14th Learning An-
alytics and Knowledge Conf., page 563–575.
Real, E. M., Pimentel, E. P., and Braga, J. C. (2021). Anal-
ysis of learning behavior in a programming course us-
ing process mining and sequential pattern mining. In
2021 IEEE Frontiers in Edu. Conf. (FIE), pages 1–9.
Reimann, P., Markauskaite, L., and Bannert, M. (2014). e
research and learning theory: What do sequence and
process mining methods contribute? British Journal
of Educational Technology, 45(3):528–540.
Romero, C., Cerezo, R., Bogar
´
ın, A., and S
´
anchez-
Santill
´
an, M. (2016). Educational Process Mining:
A Tutorial and Case Study Using Moodle Data Sets,
chapter 1, pages 1–28. John Wiley and Sons, Ltd.
Rotelli, D. and Monreale, A. (2023). Processing and under-
standing moodle log data and their temporal dimen-
sion. Journal of Learning Analytics, 10:126–141.
Shabatura, J. (2022). Using bloom’s taxonomy to write ef-
fective learning outcomes. University of Arkansas.
Song, Y., Oliveira, E., Kirley, M., and Thompson, P. (2024).
A case study on university student online learning pat-
terns across multidisciplinary subjects. In Proceed-
ings of the 14th Learning Analytics and Knowledge
Conf., page 936–942.
Suriadi, S., Andrews, R., ter Hofstede, A., and Wynn, M.
(2017). Event log imperfection patterns for process
mining: Towards a systematic approach to cleaning
event logs. Information Systems, 64:132–150.
Umer, R., Susnjak, T., Mathrani, A., and Suriadi, S.
(2022). Data quality challenges in educational process
mining: Building process-oriented event logs from
process-unaware online learning systems. Int. Jour-
nal of Business Information Systems, 39:569 – 592.
Van der Aalst, W. (2016). Process mining: data science in
action. Springer.
Wafda, F., Usagawa, T., and Mahendrawathi, E. (2022).
Systematic literature review on process mining in
learning management system. IEEE Int. Conf. on In-
dustry 4.0, Artificial Intelligence, and Communica-
tions Technology (IAICT), pages 160–166.
Yu, H., Harper, S., and Vigo, M. (2021). Modeling micro-
interactions in self-regulated learning: A data-driven
methodology. Int. Journal of Human-Computer Stud-
ies, 151:102625.
´
Alvarez, P., Fabra, J., Hern
´
andez, S., and Ezpeleta, J.
(2016). Alignment of teacher’s plan and students’ use
of lms resources: Analysis of moodle logs. In 15th
Int. Conf. on Information Technology Based Higher
Education and Training (ITHET), pages 1–8.
Moodle2EventLog: A Tool for Pedagogically-Driven Log Enrichment and Analysis
463