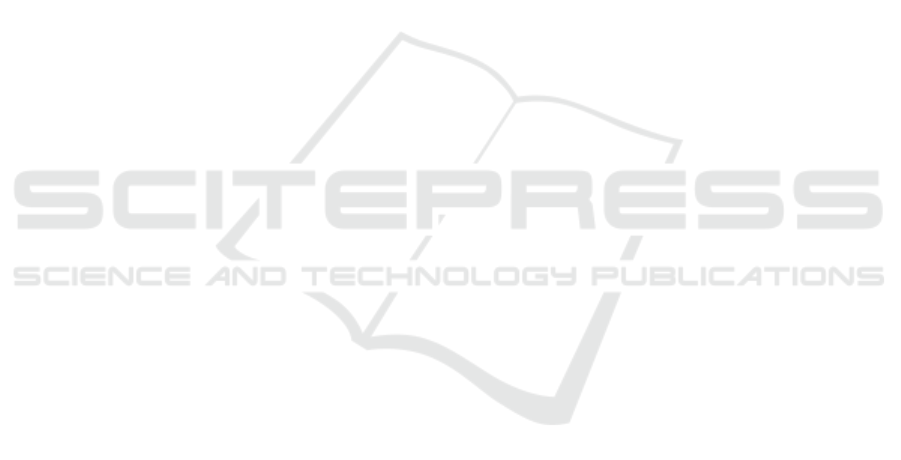
evaluate losses that exceed the predetermined confi-
dence level, it may show an optimistic tendency rather
than a conservative one, which should prevail in risk
management.
In 2000’s a new risk measure called Conditional
Value at Risk (CVaR) was proposed by (Rockafellar
and Uryasev, 2000). CVaR is the average of the ex-
pected returns that exceed VaR’s for a given confi-
dence level. CVaR consists of the expectancy of the
tail values of the probability distribution that repre-
sent the worst-case return scenarios (Rockafellar and
Uryasev, 2000). Accordingly, CVaR is able to mea-
sure the ”tail losses” under a more robust approach.
Additionally, CVaR exhibits superior properties com-
pared to VaR, since (Artzner et al., 1999) stated that
CVaR can be considered as a coherent risk measure by
having a group of properties which includes subaddi-
tivity: CVaR
α
(R
1
+ R
2
) ≤ CVaR
α
(R
1
) + CVaR
α
(R
2
)
- that is a property that VaR does not meet.
The objective functions comprehended in op-
timization problems originated from MPT can be
placed within the concept of utility functions, espe-
cially since the predominance of Utility Theory as the
main method of decision-making under uncertainty at
that time. Utility Theory is based on the assumption
of the rational investor, and has served as the primary
lens through which the behavior of economic agents
has been interpreted. However, an alternative method
has been disseminated in decision-making analysis
since (Kahneman and Tversky, 1979). That paper in-
troduced Prospect Theory, fundamentally altering the
decision-making analysis by demonstrating that peo-
ple weight losses more heavily than gains in general
situations, contradicting the assumption of the ratio-
nal investor.
Prospect Theory diverges from Utility Theory by
considering that decisions are affected by other bi-
ases instead of the assumption taken in the con-
cept of rational investor. (Kahneman and Tversky,
1979) posited that the value function in Prospect The-
ory is characterized by a deviation from a reference
point, exhibiting concavity for gains and convexity
for losses and having greater weight on the convex
part than on the concave one. Further refinements by
(Kahneman and Tversky, 1992) incorporated nonlin-
ear preferences, a concept of loss aversion and a cu-
mulative function which allows applications for con-
tinuous variables.
Other researches in the literature have tested the
effectiveness of Prospect Theory in explaining in-
vestor behavior, as well as in explaining portfolio re-
turns or even in understanding market tendencies.
Subsequent researches have extensively tested the
applicability of Prospect Theory in explaining in-
vestor behavior and portfolio returns across various
market sectors. For instance, (Benartzi and Thaler,
1995) examined the equity premium puzzle — why
American stocks outperformed bonds throughout the
20th century — by presenting two main arguments.
The first argument is based on Prospect Theory, as-
serting that investors are more sensitive to losses than
to gains. In other words, investors tend to treat the
possibility of losses more severely and seek greater
possibilities of gains. The second argument addresses
a distinct concept known as ”myopic loss aversion”,
which means that people tend to show more con-
cern about their portfolios in a short-period and do
not show the same concern for long-term results.
The paper presented a piecewise linear optimization
model accordingly and the results defined a period
of investor’s indifference towards their portfolios that
proper justified the “equity premium puzzle” from the
authors’ perspective.
Further studies, such as those by (Benartzi and
Thaler, 1995) and (Barberis et al., 2001) have demon-
strated that asset prices that were influenced by loss
aversion were closely aligned with historical data
while showed minimal correlation with consumption
growth. The broad dissemination of Prospect Theory
influenced (Barberis and Thaler, 2003) to critique and
highlight the theoretical distinctions between rational
and non-rational investor profiles, identifying chal-
lenges in arbitrage limits and noting a lack of practical
applications at that time.
A few years later, (De Giorgi et al., 2010) argued
that the financial market would not need to adopt the
equilibrium hypotheses if agents had heterogeneous
preferences in accordance with Cumulative Prospect
Theory (Kahneman and Tversky, 1992). The paper
contradicted traditional financial models in which this
concept of equilibrium was fundamental.
Specifically in portfolio problems, Prospect The-
ory has been applied as an alternative approach to
explain returns, risk and the overall decision-making
by investors in different scenarios. (Best and Grauer,
2016) proposed a multi-period problem for maximiz-
ing returns, in which the concept of loss aversion was
applied and the loss aversion coefficient from (Kahne-
man and Tversky, 1992) was used in a portfolio with
different assets and rates. The paper also considers
the concept of kink, that is the non-differentiable seg-
ment that connects the gain-curve to the loss-curve of
the Prospect Theory’s value function. Relevant op-
portunities in optimization have arisen regarding the
concept of kink. There has been particular interest in
this application, specially by the fact it has been quite
challenging. (Best and Zhang, 2011) and (Best et al.,
2014) are examples of this application.
FEMIB 2025 - 7th International Conference on Finance, Economics, Management and IT Business
52