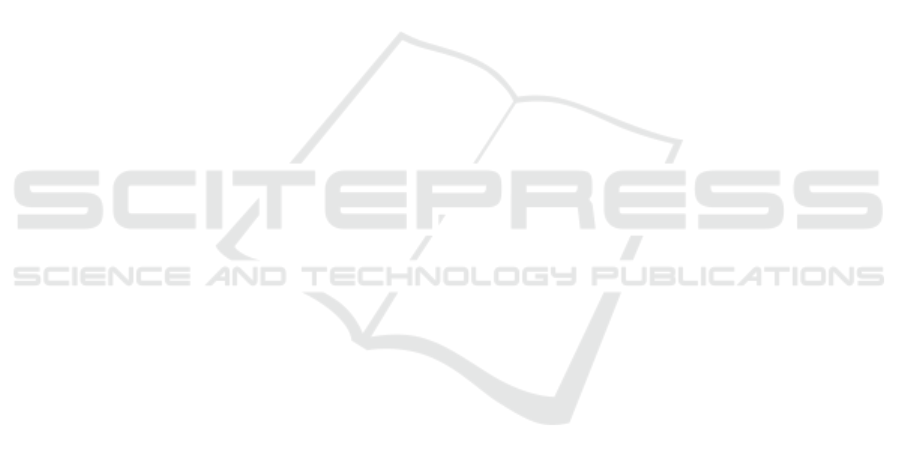
ACKNOWLEDGEMENTS
Authors thank MCTI, ANEEL, CESP and Auren for
financial support (Project CTP - 1283 - Plataforma
Tecnol
´
ogica para Digitalizac¸
˜
ao da Portabilidade e
Agregac¸
˜
ao da Medic¸
˜
ao no Ambiente Varejista de En-
ergia) and research grant 03b1629f-e9d9-40bb-bc95-
89d7362e28c2.
REFERENCES
Adewole, K. S. and Torra, V. (2024). Energy disaggregation
risk resilience through microaggregation and discrete
fourier transform. Information Sciences, 662:120211.
Berghel, S., Bohannon, P., Desfontaines, D., Estes, C.,
Haney, S., Hartman, L., Hay, M., Machanavajjhala,
A., Magerlein, T., Miklau, G., Pai, A., Sexton, W., and
Shrestha, R. (2022). Tumult analytics: a robust, easy-
to-use, scalable, and expressive framework for differ-
ential privacy. arXiv preprint arXiv:2212.04133.
Casacuberta, S., Shoemate, M., Vadhan, S., and Wagaman,
C. (2022). Widespread underestimation of sensitivity
in differentially private libraries and how to fix it.
Dwork, C. (2006). Differential privacy. pages 1–12.
Dwork, C., Kenthapadi, K., McSherry, F., Mironov, I., and
Naor, M. (2006a). Our data, ourselves: Privacy via
distributed noise generation. pages 486–503.
Dwork, C., McSherry, F., Nissim, K., and Smith, A.
(2006b). Calibrating noise to sensitivity in private data
analysis. pages 265–284.
ENISA (2023). Cybersecurity and privacy in ai – fore-
casting demand on electricity grids. Technical report.
ENISA.
Gaboardi, M., Hay, M., and Vadhan, S. (2020). A program-
ming framework for opendp. Manuscript, May.
Garrido, G. M., Near, J., Muhammad, A., He, W., Matzutt,
R., and Matthes, F. (2021). Do i get the privacy i need?
benchmarking utility in differential privacy libraries.
arXiv preprint arXiv:2109.10789.
Ghosh, S., Alam, M., Dey, S., and Mukhopadhyay, D.
(2024). “hello? is there anybody in there?” leak-
age assessment of differential privacy mechanisms
in smart metering infrastructure. In P
¨
opper, C.
and Batina, L., editors, Applied Cryptography and
Network Security, pages 163–189. Springer Nature
Switzerland.
Holohan, N., Braghin, S., Aonghusa, P. M., and Levacher,
K. (2019). Diffprivlib: the ibm differential privacy
library. ArXiv e-prints, 1907.02444 [cs.CR].
Janghyun, K., Barry, H., Tianzhen, H., et al. (2022). A
review of preserving privacy in data collected from
buildings with differential privacy. Journal of Build-
ing Engineering, 56:104724.
Jin, J., McMurtry, E., Rubinstein, B. I. P., and Ohrimenko,
O. (2022). Are we there yet? timing and floating-point
attacks on differential privacy systems. In IEEE Symp.
on Security and Privacy (SP), pages 473–488.
Leukam Lako, F., Lajoie-Mazenc, P., and Laurent, M.
(2021). Privacy-preserving publication of time-series
data in smart grid. Security and Communication Net-
works, 2021(1):6643566.
Marks, J., Montano, B., Chong, J., Raavi, M., Islam, R.,
Cerny, T., and Shin, D. (2021). Differential privacy
applied to smart meters: A mapping study. pages 761–
770. Association for Computing Machinery.
McElroy, T., Roy, A., and Hore, G. (2023). Flip: a utility
preserving privacy mechanism for time series. Journal
of Machine Learning Research, 24(111):1–29.
Mironov, I. (2012). On significance of the least signifi-
cant bits for differential privacy. In Proceedings of
the 2012 ACM conference on Computer and commu-
nications security, pages 650–661.
Near, J. P., Darais, D., Lefkovitz, N., Howarth, G., et al.
(2023). Guidelines for evaluating differential privacy
guarantees (nist sp 800-226). Technical report, Na-
tional Institute of Standards and Technology.
Ngong, I. C., Stenger, B., Near, J. P., and Feng, Y.
(2023). Evaluating the usability of differential pri-
vacy tools with data practitioners. arXiv preprint
arXiv:2309.13506.
OpenMined (2020). Pydp: The python differential privacy
library. https://github.com/OpenMined/PyDP. Ac-
cessed: 2024-08-07.
Paix
˜
ao, A. C. P., Camargo, G. F. L., and Braga, A. M.
(2025). Testing open-source libraries for private
counts and averages on energy metering time series.
20th European Dependable Computing Conference.
Peralta-Peterson, M. and Kotevska, O. (2021). Effective-
ness of privacy techniques in smart metering systems.
pages 675–678.
Roman, A.-S. (2023). Evaluating the privacy and utility of
time-series data perturbation algorithms. Mathemat-
ics, 11(5):1260.
Roman, A.-S., Genge, B., Duka, A.-V., and Haller, P.
(2021). Privacy-preserving tampering detection in au-
tomotive systems. Electronics, 10(24):3161.
Shaham, S., Ghinita, G., Krishnamachari, B., and Shahabi,
C. (2024). Differentially private publication of elec-
tricity time series data in smart grids. arXiv preprint
arXiv:2408.16017.
Stoltzfus, J. C. (2015). Student’s t-test for independent sam-
ples. Int’l Journal of Academic Medicine, 1:27–28.
Wiese, F., Schlecht, I., Bunke, W.-D., Gerbaulet, C., Hirth,
L., Jahn, M., Kunz, F., Lorenz, C., M
¨
uhlenpfordt, J.,
Reimann, J., et al. (2019). Open power system data–
frictionless data for electricity system modelling. Ap-
plied Energy, 236:401–409.
Zhang, S., Hagermalm, A., Slavnic, S., Schiller, E. M., and
Almgren, M. (2023). Evaluation of open-source tools
for differential privacy. Sensors, 23(14).
Zhao, J., Jung, T., Wang, Y., and Li, X. (2014). Achiev-
ing differential privacy of data disclosure in the smart
grid. pages 504–512.
IoTBDS 2025 - 10th International Conference on Internet of Things, Big Data and Security
296