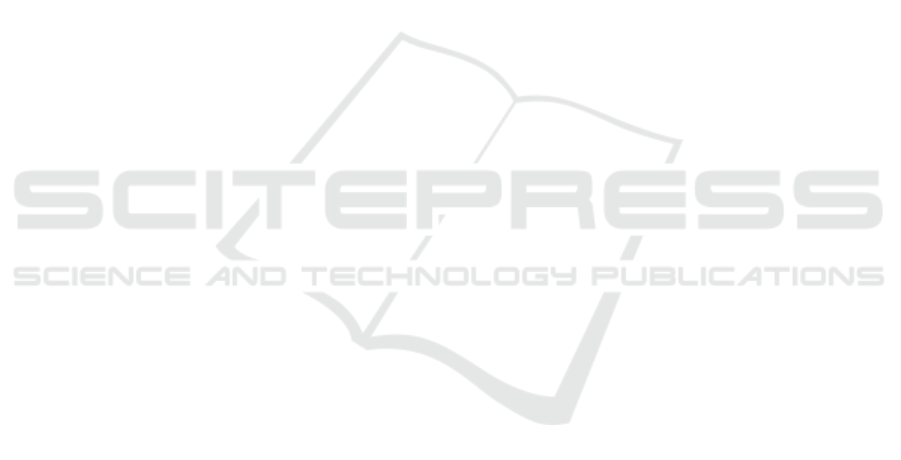
machine, and they could take breaks, whose duration
was not counted in the task execution time. Partici-
pants were instructed not to communicate with each
other, and they were also informed that they were not
being evaluated in any way via the empirical study.
To make the environment as friendly as possible, ses-
sions were supervised by another Master’s student.
Different participants obtained similar results for
common methods. Also the mean times taken by par-
ticipants to complete all the tasks assigned were sim-
ilar. No participant was consistently better or worse
than the others: as in real organizations each devel-
oper performed better than colleagues in some tasks
and worse in others.
Models of task completion time as a function of
code measures were built using several techniques,
namely Support Vector Regression (SVR), Random
Forests (RF) and Neural Networks (NN). Models used
up to four code features, to avoid overfitting. The ob-
tained models were evaluated based on the Mean Ab-
solute Residual (MAR), also known as Mean Abso-
lute Error, and the mean of relative residuals, which
is the ratio of MAR and the mean of the considered
property (in this case, the task completion time).
The models built with different techniques were
similarly accurate. The greatest majority of the ob-
tained models had relative errors in the 27%–32%
range. This result indicates that there is a correla-
tion between the measured characteristics of code and
understandability; however, the extent of the error in-
dicates that understandability depends also on other
factors, not quantified by the considered measures.
3 THE REPLICATION
The replicated empirical study took place in April
2024 at the University of Cyprus in Nicosia, Cyprus,
with the participation of undergraduate students at-
tending the Bachelor program of Computer Science
at the Department of Computer Science. The third
and fourth year students were informed of the empir-
ical study via email, and interested students indicated
their willingness to participate. The prospective par-
ticipants were informed that no personal data would
be used in any way (actually, no personal data were
collected). Only the results of the tasks would be
used, with no connection to the identity or other char-
acteristics of who carried out the task. All participants
gave their consent to the above. The empirical study
was not linked to any specific course in the Bachelor
program or any grading process, but a small monetary
reward was given to each participant regardless of the
result of the empirical study.
The supplemental material of the new empirical
study is available online at https://anonymous.
4open.science/r/code-understandability-replicated-
experiment-0E6B/README.md. Since the original
study was already provided with the replication
package, our supplemental material includes only the
raw data collected and the results of the experiment.
The Java projects and the Java methods used in the
new empirical study are as in the original one.
The original study suggests that there can be mul-
tiple factors that affect code understandability, beyond
those represented by the considered static code met-
rics. These additional factors are out of the scope
of the new study, which replicates the original study,
seeking confirmation of the previous findings.
3.1 Organization of the Empirical Study
The empirical study was executed in one four-hour
session, with all seven participants: participants were
all third and fourth year undergraduate students that
had regularly followed the Computer Science Bache-
lor’s program, consisting of compulsory and elective
courses. Concerning their prior knowledge, all partic-
ipants had attended a course on object-oriented pro-
gramming, one course on software engineering (and
in total three courses that used Java as main program-
ming language); however, participants had attended a
different set of elective courses based on their pref-
erences and the year of study, so their programming
competences might differ. One of the authors was re-
sponsible for conducting the empirical study and was
present in the room when it took place, but did not
intervene in any of the assigned tasks.
At the beginning of the four-hour session, instruc-
tions were given to the participants to replicate the set-
tings of the original empirical study. The participants
were given access to the Open Source Java projects
(JSON-Java and Jsoniter) used in the original empir-
ical study, and were asked to perform maintenance
tasks, i.e., bug fixing on the code: each participant
was given eight methods to fix (four methods from
each software project), whereas some methods were
assigned to more than one participant. 14 methods
from each project were used in total.
The participants were requested to document the
time needed to correctly complete the tasks. Specifi-
cally, they were asked to document the time spent to
identify and fix the bug. To check whether a bug was
correctly fixed, unit tests were used, as in the original
empirical study. Participants were also free to take
breaks that did not count towards the task completion
time. The Eclipse IDE was used as in the original
study and participants were free to use resources on
A Replicated Study on Factors Affecting Software Understandability
585