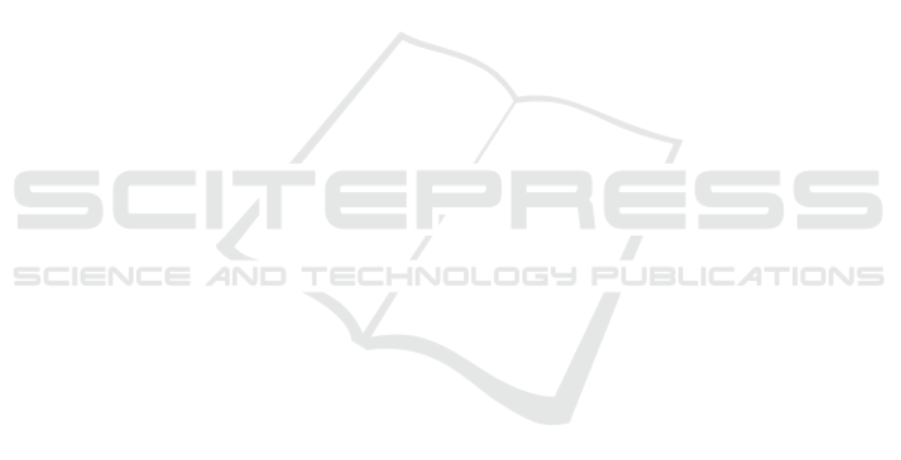
5.2 Future Work
In this paper, we propose a method targeting web UIs,
with a focus on the generation of initial test inputs for
fuzzing. This approach can be combined with existing
black-box-based fuzzing techniques.
Examples of black-box-based fuzzing techniques
include FuzzSim (Woo et al., 2013) and IoT
Fuzzer (Chen et al., 2018). By using the proposed
method as a basis, these existing black-box-based
fuzzing techniques can mutate the given test inputs to
continuously generate new test inputs. This approach
enables the verification of even more vulnerabilities.
Thus, the method proposed in this paper is useful in
that it can be integrated with existing mutation tech-
niques to increase the effectiveness of fuzzing efforts.
6 CONCLUSION
In this paper, we propose an automatic test input gen-
eration method for fuzzing the management interfaces
of IoT devices. In the proposed method, the auto-
mated web UI navigation function identifies the in-
put fields. The test input generation function creates
appropriate test inputs by analyzing the surrounding
information of each input field. By leveraging these
functions, we establish a method for automatically
generating test inputs specifically for the web UIs of
IoT devices. Furthermore, the proposed method re-
vises the generated test inputs by interpreting error
messages displayed in the web UI. The experimental
results demonstrate that test inputs that are suitable
for the input fields are successfully generated. Future
work will include the efficient mutation of the test in-
put for fuzzing.
ACKNOWLEDGEMENTS
The results of this research were obtained
in part through a contract research project
(JPJ012368C08101) sponsored by the National
Institute of Information and Communications
Technology (NICT).
REFERENCES
Chen, J., Diao, W., Zhao, Q., Zuo, C., Lin, Z., Wang,
X., Lau, W. C., Sun, M., Yang, R., and Zhang, K.
(2018). Iotfuzzer: Discovering memory corruptions
in iot through app-based fuzzing. In 25th Annual
Network and Distributed System Security Symposium,
NDSS.
Eceiza, M., Flores, J. L., and Iturbe, M. (2021). Fuzzing
the internet of things: A review on the techniques and
challenges for efficient vulnerability discovery in em-
bedded systems. IEEE Internet of Things Journal,
8(13):10390–10411.
Feng, X., Sun, R., Zhu, X., Xue, M., Wen, S., Liu, D.,
Nepal, S., and Xiang, Y. (2021). Snipuzz: Black-
box fuzzing of iot firmware via message snippet in-
ference. In Proceedings of the 2021 ACM SIGSAC
Conference on Computer and Communications Secu-
rity, page 337–350.
Jiang, A. Q., Sablayrolles, A., Mensch, A., Bamford,
C., Chaplot, D. S., de las Casas, D., Bressand, F.,
Lengyel, G., Lample, G., Saulnier, L., Lavaud, L. R.,
Lachaux, M.-A., Stock, P., Scao, T. L., Lavril, T.,
Wang, T., Lacroix, T., and Sayed, W. E. (2023). Mis-
tral 7b.
Kim, M., Kim, D., Kim, E., Kim, S., Jang, Y., and Kim, Y.
(2020). Firmae: Towards large-scale emulation of iot
firmware for dynamic analysis. In Proceedings of the
36th Annual Computer Security Applications Confer-
ence, page 733–745.
Redini, N., Continella, A., Das, D., De Pasquale, G., Spahn,
N., Machiry, A., Bianchi, A., Kruegel, C., and Vigna,
G. (2021). Diane: Identifying fuzzing triggers in apps
to generate under-constrained inputs for iot devices. In
2021 IEEE Symposium on Security and Privacy (SP),
pages 484–500.
Song, C., Yu, B., Zhou, X., and Yang, Q. (2019). Spfuzz:
A hierarchical scheduling framework for stateful net-
work protocol fuzzing. IEEE Access, 7:18490–18499.
Wei, J., Wang, X., Schuurmans, D., Bosma, M., Xia, F.,
Chi, E., Le, Q. V., Zhou, D., et al. (2022). Chain-of-
thought prompting elicits reasoning in large language
models. Advances in neural information processing
systems, 35:24824–24837.
Woo, M., Cha, S. K., Gottlieb, S., and Brumley, D. (2013).
Scheduling black-box mutational fuzzing. In Proceed-
ings of the 2013 ACM SIGSAC Conference on Com-
puter & Communications Security, page 511–522. As-
sociation for Computing Machinery.
You, M., Kim, Y., Kim, J., Seo, M., Son, S., Shin, S.,
and Lee, S. (2022). Fuzzdocs: An automated se-
curity evaluation framework for iot. IEEE Access,
10:102406–102420.
Zhang, H., Lu, K., Zhou, X., Yin, Q., Wang, P., and Yue,
T. (2021). Siotfuzzer: Fuzzing web interface in iot
firmware via stateful message generation. Applied Sci-
ences, 11(7).
Zheng, L., Chiang, W.-L., Sheng, Y., Zhuang, S., Wu, Z.,
Zhuang, Y., Lin, Z., Li, Z., Li, D., Xing, E. P., Zhang,
H., Gonzalez, J. E., and Stoica, I. (2023). Judging
llm-as-a-judge with mt-bench and chatbot arena.
Zheng, Y., Davanian, A., Yin, H., Song, C., Zhu, H.,
and Sun, L. (2019). FIRM-AFL: High-Throughput
greybox fuzzing of IoT firmware via augmented pro-
cess emulation. In 28th USENIX Security Symposium
(USENIX Security 19), pages 1099–1114.
IoTBDS 2025 - 10th International Conference on Internet of Things, Big Data and Security
304