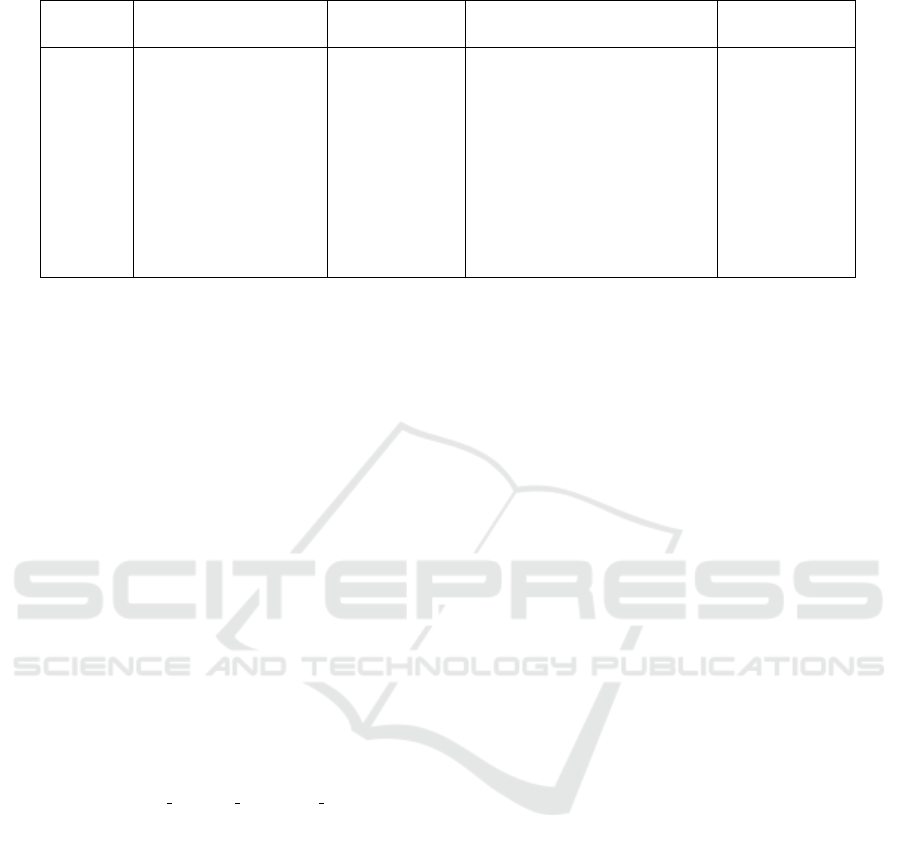
Table 3: Selected experts and profile.
Expert Education Area of ex-
pertise
Institution Experience
Expert 1 PhD in Computer
Science
Data Science Universidade de Pernam-
buco
20 years
Expert 2 Msc in Computer
Science
Data Science F
´
abrica de Neg
´
ocios 18 years
Expert 3 PhD in Computer
Science
Data Science Universidade Federal Rural
Pernambuco
20 years
Expert 4 Msc in Electrical En-
gineering
Data Science Universidade de Pernam-
buco
10 years
Expert 5 PhD in Computer
Science
Data Science Universidade Federal do
Vale do S˜ao Francisco
20 years
ACKNOWLEDGEMENTS
This paper was financed in part by the Coordenac¸
˜
ao
de Aperfeic¸oamento de Pessoal de N
´
ıvel Superior -
Brazil (CAPES) - Finance Code 001, Fundac¸
˜
ao de
Amparo a Ci
ˆ
encia e Tecnologia do Estado de Pernam-
buco (FACEPE), the Conselho Nacional de Desen-
volvimento Cient
´
ıfico e Tecnol
´
ogico (CNPq) - Brazil-
ian research agencies.
REFERENCES
Auto-Sklearn. Manual — autosklearn 0.15.0 docu-
mentation,automl.github.io. https://automl.github.io/
auto-sklearn/master/manual.html.
Auto-Sklearn. Parallel usage: Spawning workers from
the command line — autosklearn 0.15.0 doc-
umentation,” automl.github.io. https://automl.
github.io/auto-sklearn/master/examples/60search/
exampleparallel\ manual\ spawning\ cli.html\
sphx-glr-examples-60-search-example-parallel-
manual-spawning-cli-py.
Chambers, B. and Zaharia, M. (2018). Spark: The
Definitive Guide Big Data Processing Made Simple.
O’Reilly Media, Inc., 1st edition.
Dean, J. and Ghemawat, S. (2008). Mapreduce: simpli-
fied data processing on large clusters. Commun. ACM,
51(1):107–113.
Feurer, M., Eggensperger, K., Falkner, S., Lindauer, M., and
Hutter, F. (2022). Auto-sklearn 2.0: hands-free automl
via meta-learning. J. Mach. Learn. Res., 23(1).
Feurer, M., Klein, A., Eggensperger, K., Springenberg,
J. T., Blum, M., and Hutter, F. (2015). Efficient and
robust automated machine learning. In Proceedings
of the 28th International Conference on Neural Infor-
mation Processing Systems - Volume 2, NIPS’15, page
2755–2763, Cambridge, MA, USA. MIT Press.
FMD (2024). Fmdev. https://github.com/GPCDA/FMD.
Frank, C. (2013). The Big Data Long Tail — devx.com.
https://www.devx.com/blog/the-big-data-long-tail.
[Accessed May-09-2024].
Gonc¸alves, A., Maciel, A., and Rodrigues, R. (2017). De-
velopment of a data mining education framework for
visualization of data in distance learning environ-
ments. pages 547–550.
Gouveia, C. C. (2004). Teste de integrac¸
˜
ao para sistemas
baseados em componentes. Master’s thesis, Universi-
dade Federal da Para
´
ıba, Campina Grande, Para
´
ıba.
Hutter, F., Kotthoff, L., and Vanschoren, J. (2019). Auto-
mated Machine Learning: Methods, Systems, Chal-
lenges. Springer Publishing Company, Incorporated,
1st edition.
Jin, Z. and Offunt, A. J. (1998). Coupling-based criteria
for integration testing. SOFTWARE TESTING,
VERIFICATION AND RELIABILITY 8. Dispon
´
ıvel
em: https://onlinelibrary.wiley.com/doi/abs/10.
1002/%28SICI%291099-1689%281998090%298%
3A3%3C133%3A%3AAID-STVR162%3E3.0.CO%
3B2-M. Acesso em: 16 mai. 2024.
Junior, V., Ceci, F., WOSZEZENKI, C., and Goncalves,
A. (2017). Design science research methodology as
methodological strategy for technological research.
Espacios, 38:25.
Komer, B., Bergstra, J., and Eliasmith, C. (2014).
Hyperopt-sklearn: Automatic hyperparameter config-
uration for scikit-learn. In SciPy.
Lacerda, D., Dresch, A., Proenc¸a, A., and Antunes J
´
unior,
J. A. V. (2012). Design science research: A re-
search method to production engineering. Gest
˜
ao &
Produc¸
˜
ao, 20:741–761.
Le, T. T., Fu, W., and Moore, J. H. (2019). Scaling
tree-based automated machine learning to biomedical
big data with a feature set selector. Bioinformatics,
36(1):250–256.
Olson, R. S., Bartley, N., Urbanowicz, R. J., and Moore,
J. H. (2016). Evaluation of a tree-based pipeline opti-
mization tool for automating data science.
Rodrigues, J. B. (2020). An
´
alise de fatores relevantes no
desempenho de plataformas para processamento de
Big Data : Uma abordagem baseada em projeto de
Development of a Big Data Mechanism for AutoML
299