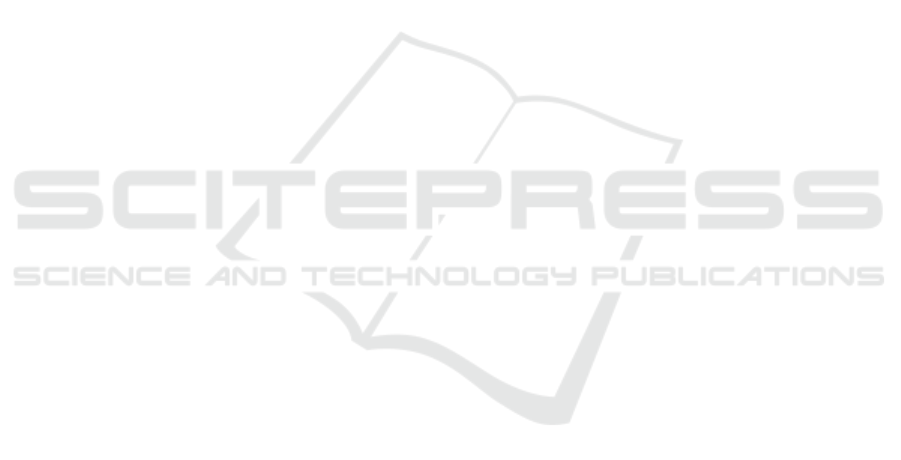
Strube, M., editors, Proceedings of the 53rd Annual
Meeting of the Association for Computational Lin-
guistics and the 7th International Joint Conference on
Natural Language Processing (Volume 2: Short Pa-
pers), pages 781–787, Beijing, China. Association for
Computational Linguistics.
Li, X., Bing, L., Zhang, W., Li, Z., and Lam, W. (2020). Un-
supervised cross-lingual adaptation for sequence tag-
ging and beyond. arXiv preprint arXiv:2010.12405.
Lin, N., Fu, Y., Lin, X., Zhou, D., Yang, A., and Jiang,
S. (2023). Cl-xabsa: Contrastive learning for cross-
lingual aspect-based sentiment analysis. IEEE/ACM
Transactions on Audio, Speech, and Language Pro-
cessing, 31:2935–2946.
Lin, N., Zeng, M., Liao, X., Liu, W., Yang, A., and Zhou,
D. (2024). Addressing class-imbalance challenges
in cross-lingual aspect-based sentiment analysis: Dy-
namic weighted loss and anti-decoupling. Expert Sys-
tems with Applications, 257:125059.
Loshchilov, I. and Hutter, F. (2017). Decoupled weight de-
cay regularization. arXiv preprint arXiv:1711.05101.
Mao, Y., Shen, Y., Yang, J., Zhu, X., and Cai, L. (2022).
Seq2Path: Generating sentiment tuples as paths of a
tree. In Muresan, S., Nakov, P., and Villavicencio,
A., editors, Findings of the Association for Compu-
tational Linguistics: ACL 2022, pages 2215–2225,
Dublin, Ireland. Association for Computational Lin-
guistics.
OpenAI (2024). GPT-4o. Accessed November 2024.
Pontiki, M., Galanis, D., Papageorgiou, H., Androutsopou-
los, I., Manandhar, S., AL-Smadi, M., Al-Ayyoub, M.,
Zhao, Y., Qin, B., De Clercq, O., Hoste, V., Apid-
ianaki, M., Tannier, X., Loukachevitch, N., Kotel-
nikov, E., Bel, N., Jim
´
enez-Zafra, S. M., and Eryi
˘
git,
G. (2016). SemEval-2016 task 5: Aspect based sen-
timent analysis. In Bethard, S., Carpuat, M., Cer,
D., Jurgens, D., Nakov, P., and Zesch, T., editors,
Proceedings of the 10th International Workshop on
Semantic Evaluation (SemEval-2016), pages 19–30,
San Diego, California. Association for Computational
Linguistics.
Pontiki, M., Galanis, D., Pavlopoulos, J., Papageorgiou,
H., Androutsopoulos, I., and Manandhar, S. (2014).
SemEval-2014 task 4: Aspect based sentiment analy-
sis. In Nakov, P. and Zesch, T., editors, Proceedings
of the 8th International Workshop on Semantic Evalu-
ation (SemEval 2014), pages 27–35, Dublin, Ireland.
Association for Computational Linguistics.
Raffel, C., Shazeer, N., Roberts, A., Lee, K., Narang, S.,
Matena, M., Zhou, Y., Li, W., and Liu, P. J. (2020).
Exploring the limits of transfer learning with a uni-
fied text-to-text transformer. The Journal of Machine
Learning Research, 21(1):5485–5551.
Shazeer, N. and Stern, M. (2018). Adafactor: Adaptive
learning rates with sublinear memory cost. In Dy, J. G.
and Krause, A., editors, Proceedings of the 35th In-
ternational Conference on Machine Learning, ICML
2018, Stockholmsm
¨
assan, Stockholm, Sweden, July
10-15, 2018, volume 80 of Proceedings of Machine
Learning Research, pages 4603–4611. PMLR.
ˇ
Sm
´
ıd, J., Priban, P., and Kral, P. (2024). LLaMA-
based models for aspect-based sentiment analysis. In
De Clercq, O., Barriere, V., Barnes, J., Klinger, R.,
Sedoc, J., and Tafreshi, S., editors, Proceedings of the
14th Workshop on Computational Approaches to Sub-
jectivity, Sentiment, & Social Media Analysis, pages
63–70, Bangkok, Thailand. Association for Computa-
tional Linguistics.
Tang, Y., Tran, C., Li, X., Chen, P.-J., Goyal, N., Chaud-
hary, V., Gu, J., and Fan, A. (2020). Multilin-
gual translation with extensible multilingual pretrain-
ing and finetuning. arXiv preprint arXiv:2008.00401.
Vaswani, A., Shazeer, N., Parmar, N., Uszkoreit, J., Jones,
L., Gomez, A. N., Kaiser, L. u., and Polosukhin,
I. (2017). Attention is all you need. In Guyon,
I., Luxburg, U. V., Bengio, S., Wallach, H., Fer-
gus, R., Vishwanathan, S., and Garnett, R., editors,
Advances in Neural Information Processing Systems,
volume 30, page 6000–6010. Curran Associates, Inc.
Wan, H., Yang, Y., Du, J., Liu, Y., Qi, K., and Pan,
J. Z. (2020). Target-aspect-sentiment joint detec-
tion for aspect-based sentiment analysis. Proceed-
ings of the AAAI Conference on Artificial Intelligence,
34(05):9122–9129.
Wang, W. and Pan, S. J. (2018). Transition-based adver-
sarial network for cross-lingual aspect extraction. In
IJCAI, pages 4475–4481.
Wolf, T., Debut, L., Sanh, V., Chaumond, J., Delangue, C.,
Moi, A., Cistac, P., Rault, T., Louf, R., Funtowicz,
M., Davison, J., Shleifer, S., von Platen, P., Ma, C.,
Jernite, Y., Plu, J., Xu, C., Le Scao, T., Gugger, S.,
Drame, M., Lhoest, Q., and Rush, A. (2020). Trans-
formers: State-of-the-art natural language processing.
In Liu, Q. and Schlangen, D., editors, Proceedings of
the 2020 Conference on Empirical Methods in Nat-
ural Language Processing: System Demonstrations,
pages 38–45, Online. Association for Computational
Linguistics.
Xianlong, L., Yang, M., and Wang, Y. (2023). Tagging-
assisted generation model with encoder and decoder
supervision for aspect sentiment triplet extraction. In
Bouamor, H., Pino, J., and Bali, K., editors, Proceed-
ings of the 2023 Conference on Empirical Methods in
Natural Language Processing, pages 2078–2093, Sin-
gapore. Association for Computational Linguistics.
Xue, L., Constant, N., Roberts, A., Kale, M., Al-Rfou,
R., Siddhant, A., Barua, A., and Raffel, C. (2021).
mT5: A massively multilingual pre-trained text-to-
text transformer. In Toutanova, K., Rumshisky,
A., Zettlemoyer, L., Hakkani-Tur, D., Beltagy, I.,
Bethard, S., Cotterell, R., Chakraborty, T., and Zhou,
Y., editors, Proceedings of the 2021 Conference of the
North American Chapter of the Association for Com-
putational Linguistics: Human Language Technolo-
gies, pages 483–498, Online. Association for Compu-
tational Linguistics.
Zhang, W., Deng, Y., Li, X., Yuan, Y., Bing, L., and Lam,
W. (2021a). Aspect sentiment quad prediction as para-
phrase generation. In Moens, M.-F., Huang, X., Spe-
cia, L., and Yih, S. W.-t., editors, Proceedings of
the 2021 Conference on Empirical Methods in Nat-
Advancing Cross-Lingual Aspect-Based Sentiment Analysis with LLMs and Constrained Decoding for Sequence-to-Sequence Models
765