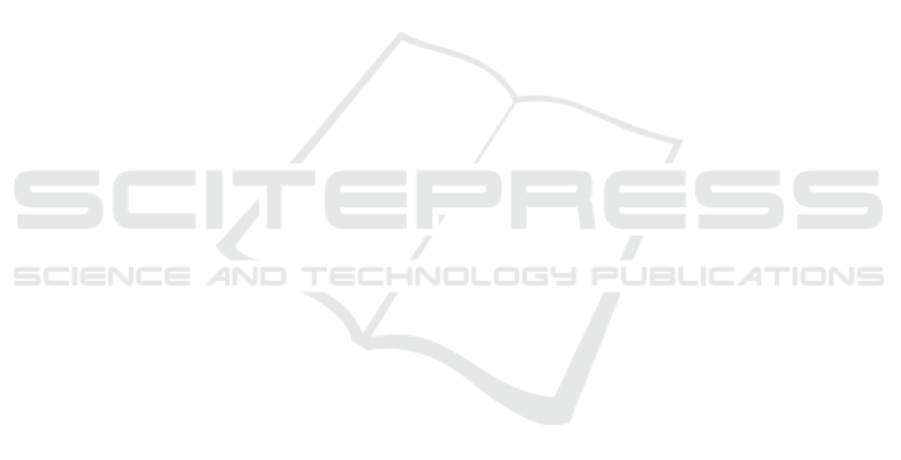
S., and Xue, N., editors, Proceedings of the 2024
Joint International Conference on Computational Lin-
guistics, Language Resources and Evaluation (LREC-
COLING 2024), pages 3046–3056, Torino, Italia.
ELRA and ICCL.
Da Corte, M. and Baptista, J. (2024b). Enhancing writ-
ing proficiency classification in developmental educa-
tion: The quest for accuracy. In Calzolari, N., Kan,
M.-Y., Hoste, V., Lenci, A., Sakti, S., and Xue, N.,
editors, Proceedings of the 2024 Joint International
Conference on Computational Linguistics, Language
Resources and Evaluation (LREC-COLING 2024),
pages 6134–6143, Torino, Italia. ELRA and ICCL.
Da Corte, M. and Baptista, J. (2024c). Leveraging NLP
and machine learning for English (l1) writing assess-
ment in developmental education. In Proceedings of
the 16th International Conference on Computer Sup-
ported Education (CSEDU 2024), 2-4 May, 2024,
Angers, France, volume 2, pages 128–140.
Da Corte, M. and Baptista, J. (2025). Toward consistency
in writing proficiency assessment: Mitigating classifi-
cation variability in developmental education. In Pro-
ceedings of CSEDU 2025, Porto, Portugal. (to ap-
pear).
Dem
ˇ
sar, J., Curk, T., Erjavec, A.,
ˇ
Crt Gorup, Ho
ˇ
cevar, T.,
Milutinovi
ˇ
c, M., Mo
ˇ
zina, M., Polajnar, M., Toplak,
M., Stari
ˇ
c, A.,
ˇ
Stajdohar, M., Umek, L.,
ˇ
Zagar, L.,
ˇ
Zbontar, J.,
ˇ
Zitnik, M., and Zupan, B. (2013). Orange:
Data Mining Toolbox in Python. Journal of Machine
Learning Research, 14:2349–2353.
Duch, D., May, M., and George, S. (2024). Empower-
ing students: A reflective learning analytics approach
to enhance academic performance. In 16th Inter-
national Conference on Computer Supported Educa-
tion (CSEDU 2024), pages 385–396. SCITEPRESS-
Science and Technology Publications.
Edgecombe, N. and Weiss, M. (2024). Promoting equity
in Developmental Education reform: A conversation
with Nikki Edgecombe and Michael Weiss. Center
for the Analysis of Postsecondary Readiness, page 1.
Feller, D. P., Sabatini, J., and Magliano, J. P. (2024). Dif-
ferentiating less-prepared from more-prepared college
readers. Discourse Processes, pages 1–23.
Filighera, A., Steuer, T., and Rensing, C. (2019). Automatic
text difficulty estimation using embeddings and neu-
ral networks. In Transforming Learning with Mean-
ingful Technologies: 14th European Conference on
Technology Enhanced Learning, EC-TEL 2019, Delft,
The Netherlands, September 16–19, 2019, Proceed-
ings 14, pages 335–348. Springer.
Fleiss, J. L. and Cohen, J. (1973). The equivalence of
weighted Kappa and the intraclass correlation coeffi-
cient as measures of reliability. Educational and psy-
chological measurement, 33(3):613–619.
Freelon, D. (2013). Recal OIR: ordinal, interval, and ratio
intercoder reliability as a web service. International
Journal of Internet Science, 8(1):10–16.
Ganga, E. and Mazzariello, A. (2019). Modernzing college
course placement by using multiple measures. Educa-
tion Commission of the States, pages 1–9.
Giordano, J. B., Hassel, H., Heinert, J., and Phillips, C.
(2024). Reaching All Writers: A Pedagogical Guide
for Evolving College Writing Classrooms, chapter
Chapter 2, pages 24–62. University Press of Colorado.
G
¨
otz, S. and Granger, S. (2024). Learner corpus research
for pedagogical purposes: An overview and some re-
search perspectives. International Journal of Learner
Corpus Research, 10(1):1–38.
Hirokawa, S. (2018). Key attribute for predicting student
academic performance. In Proceedings of the 10th In-
ternational Conference on Education Technology and
Computers, pages 308–313.
Huang, Z. (2023). An intelligent scoring system for En-
glish writing based on artificial intelligence and ma-
chine learning. International Journal of System As-
surance Engineering and Management, pages 1–8.
Hughes, S. and Li, R. (2019). Affordances and limitations
of the ACCUPLACER automated writing placement
tool. Assessing Writing, 41:72–75.
Johnson, M. S., Liu, X., and McCaffrey, D. F. (2022). Psy-
chometric methods to evaluate measurement and al-
gorithmic bias in automated scoring. Journal of Edu-
cational Measurement, 59(3):338–361.
Kim, Y.-S. G., Schatschneider, C., Wanzek, J., Gatlin, B.,
and Al Otaiba, S. (2017). Writing evaluation: Rater
and task effects on the reliability of writing scores
for children in grades 3 and 4. Reading and writing,
30:1287–1310.
Kochmar, E., Gooding, S., and Shardlow, M. (2020). De-
tecting multiword expression type helps lexical com-
plexity assessment. arXiv preprint arXiv:2005.05692.
Kosiewicz, H., Morales, C., and Cortes, K. E. (2023). The
“missing English learner”’ in higher education: How
identification, assessment, and placement shape the
educational outcomes of English learners in commu-
nity colleges. In Higher Education: Handbook of The-
ory and Research: Volume 39, pages 1–55. Springer.
Kyle, K., Crossley, S. A., and Verspoor, M. (2021). Mea-
suring longitudinal writing development using indices
of syntactic complexity and sophistication. Studies in
Second Language Acquisition, 43(4):781–812.
Laporte, E. (2018). Choosing features for classifying mul-
tiword expressions. In Sailer, M. and Markantonatou,
S., editors, Multiword expressions: In-sights from a
multi-lingual perspective, pages 143–186. Language
Science Press, Berlin.
Leal, S. E., Duran, M. S., Scarton, C. E., Hartmann, N. S.,
and Alu
´
ısio, S. M. (2023). NILC-Metrix: assess-
ing the complexity of written and spoken language in
Brazilian Portuguese. Language Resources and Eval-
uation, pages 1–38.
Lee, B. W. and Lee, J. H.-J. (2023). Prompt-based learn-
ing for text readability assessment. In In Proceed-
ings of the 18th Workshop on Innovative Use of NLP
for Building Educational Applications (BEA 2023),
pages 1–19. Toronto, Canada. Association forCompu-
tational Linguistics.
Link, S. and Koltovskaia, S. (2023). Automated Scoring of
Writing, pages 333–345. Springer International Pub-
lishing, Cham.
Refining English Writing Proficiency Assessment and Placement in Developmental Education Using NLP Tools and Machine Learning
301