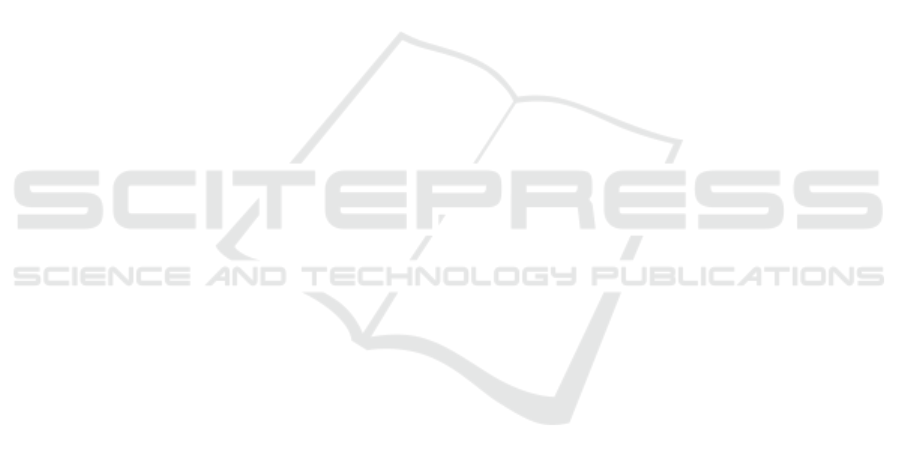
REFERENCES
Arnold, T., Ballier, N., Gaillat, T., and Liss
´
on, P. (2018).
Predicting CEFRL levels in learner English on the
basis of metrics and full texts. arXiv preprint
arXiv:1806.11099.
Barnett, E. A., Kopko, E., Cullinan, D., and Belfield, C.
(2020). Who should take college-level courses? Im-
pact findings from an evaluation of a multiple mea-
sures assessment strategy. Center for the Analysis of
Postsecondary Readiness.
Bickerstaff, S., Beal, K., Raufman, J., Lewy, E. B., and
Slaughter, A. (2022). Five principles for reforming
developmental education: A review of the evidence.
Center for the Analysis of Postsecondary Readiness,
page 1.
Chen, X. and Meurers, D. (2016). CTAP: A Web-Based
Tool Supporting Automatic Complexity Analysis. In
Proceedings of the Workshop on Computational Lin-
guistics for Linguistic Complexity (CL4LC), pages
113–119, Osaka, Japan. The COLING 2016 Organiz-
ing Committee.
Cohen, J. (1988). Statistical power analysis. Hillsdale, NJ:
Erlbaum.
Da Corte, M. and Baptista, J. (2022). A phraseology ap-
proach in developmental education placement. In Pro-
ceedings of Computational and Corpus-based Phrase-
ology, EUROPHRAS 2022, Malaga, Spain, pages 79–
86.
Da Corte, M. and Baptista, J. (2024a). Charting the linguis-
tic landscape of developing writers: An annotation
scheme for enhancing native language proficiency. In
Calzolari, N., Kan, M.-Y., Hoste, V., Lenci, A., Sakti,
S., and Xue, N., editors, Proceedings of the 2024
Joint International Conference on Computational Lin-
guistics, Language Resources and Evaluation (LREC-
COLING 2024), pages 3046–3056, Torino, Italia.
ELRA and ICCL.
Da Corte, M. and Baptista, J. (2024b). Enhancing writ-
ing proficiency classification in developmental educa-
tion: The quest for accuracy. In Calzolari, N., Kan,
M.-Y., Hoste, V., Lenci, A., Sakti, S., and Xue, N.,
editors, Proceedings of the 2024 Joint International
Conference on Computational Linguistics, Language
Resources and Evaluation (LREC-COLING 2024),
pages 6134–6143, Torino, Italia. ELRA and ICCL.
Da Corte, M. and Baptista, J. (2024c). Leveraging NLP
and machine learning for English (l1) writing assess-
ment in developmental education. In Proceedings of
the 16th International Conference on Computer Sup-
ported Education (CSEDU 2024), 2-4 May, 2024,
Angers, France, volume 2, pages 128–140.
Dem
ˇ
sar, J., Curk, T., Erjavec, A.,
ˇ
Crt Gorup, Ho
ˇ
cevar, T.,
Milutinovi
ˇ
c, M., Mo
ˇ
zina, M., Polajnar, M., Toplak,
M., Stari
ˇ
c, A.,
ˇ
Stajdohar, M., Umek, L.,
ˇ
Zagar, L.,
ˇ
Zbontar, J.,
ˇ
Zitnik, M., and Zupan, B. (2013). Orange:
Data Mining Toolbox in Python. Journal of Machine
Learning Research, 14:2349–2353.
dos Santos, S. and Junior, G. (2024). Opportunities and
challenges of AI to support student assessment in
computing education: A systematic literature review.
CSEDU (2), pages 15–26.
Dowell, N. and Kovanovic, V. (2022). Modeling educa-
tional discourse with natural language processing. Ed-
ucation, 64:82.
Duch, D., May, M., and George, S. (2024). Empower-
ing students: A reflective learning analytics approach
to enhance academic performance. In 16th Inter-
national Conference on Computer Supported Educa-
tion (CSEDU 2024), pages 385–396. SCITEPRESS-
Science and Technology Publications.
Edgecombe, N. and Weiss, M. (2024). Promoting equity in
developmental education reform: A conversation with
Nikki Edgecombe and Michael Weiss. Center for the
Analysis of Postsecondary Readiness, page 1.
Fleiss, J. L. and Cohen, J. (1973). The equivalence of
weighted kappa and the intraclass correlation coeffi-
cient as measures of reliability. Educational and psy-
chological measurement, 33(3):613–619.
Freelon, D. (2013). Recal OIR: ordinal, interval, and ratio
intercoder reliability as a web service. International
Journal of Internet Science, 8(1):10–16.
Gallardo, K. (2021). The Importance of Assessment Lit-
eracy: Formative and Summative Assessment Instru-
ments and Techniques, pages 3–25. Springer Singa-
pore, Singapore.
Ganga, E. and Mazzariello, A. (2019). Modernzing college
course placement by using multiple measures. Educa-
tion Commission of the States, pages 1–9.
Giordano, J. B., Hassel, H., Heinert, J., and Phillips, C.
(2024). Reaching All Writers: A Pedagogical Guide
for Evolving College Writing Classrooms, chapter
Chapter 2, pages 24–62. University Press of Colorado.
G
¨
otz, S. and Granger, S. (2024). Learner corpus research
for pedagogical purposes: An overview and some re-
search perspectives. International Journal of Learner
Corpus Research, 10(1):1–38.
Hirokawa, S. (2018). Key attribute for predicting student
academic performance. In Proceedings of the 10th In-
ternational Conference on Education Technology and
Computers, pages 308–313.
Huang, Z. (2023). An intelligent scoring system for En-
glish writing based on artificial intelligence and ma-
chine learning. International Journal of System As-
surance Engineering and Management, pages 1–8.
Hughes, S. and Li, R. (2019). Affordances and limitations
of the ACCUPLACER automated writing placement
tool. Assessing Writing, 41:72–75.
Kopko, E., Brathwaite, J., and Raufman, J. (2022). The next
phase of placement reform: Moving toward equity-
centered practice. research brief. Center for the Anal-
ysis of Postsecondary Readiness.
Lattek, S. M., Rieckhoff, L., V
¨
olker, G., Langesee, L.-M.,
and Clauss, A. (2024). Systematization of competence
assessment in higher education: Methods and instru-
ments. In CSEDU (2), pages 317–326.
Link, S. and Koltovskaia, S. (2023). Automated Scoring of
Writing, pages 333–345. Springer International Pub-
lishing, Cham.
Toward Consistency in Writing Proficiency Assessment: Mitigating Classification Variability in Developmental Education
149