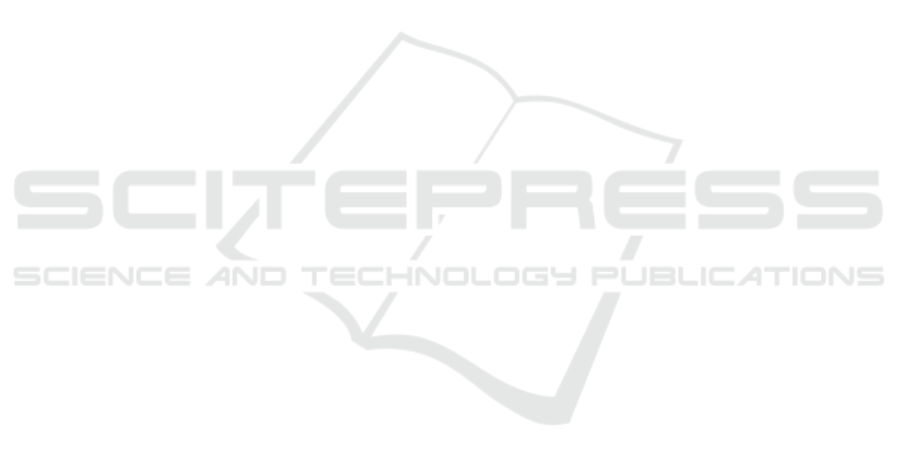
In another context, Regal et al. (2023) highlighted
in their analysis how clustering can capture the di-
verse socio-economic and functional characteristics
of urban regions, easing more tailored and efficient
delivery strategies. This work aligns with the need for
approaches that integrate socio-economic and geospa-
tial data to address the complexities of urban logistics.
The analysis of Kang (2020) complements the dis-
cussion by examining the spatial evolution of ware-
house and logistics center locations, emphasizing
their tendency to move from urban centers to the pe-
riphery despite the significant growth of online shop-
ping and the demand for instant delivery services.
Regarding machine learning algorithms, Sarkar
(2024), and Wangwattanakool and Laesanklang
(2024) explored customer segmentation and delivery
zone partitioning, both using advanced clustering al-
gorithms. Their research demonstrates how clustering
can be leveraged not only to understand customer be-
havior but also to establish delivery zones. K-means
algorithm is shown to be effective for these tasks. Be-
sides, Dupas et al. (2024) propose a K-means cluster-
ing approach to allocate customers to depots and opti-
mize vehicle routing, evaluating both the operational
efficiency and the impact of last-mile depot locations.
Lastly, Zheng et al. (2023) applied fuzzy cluster-
ing analysis to optimize logistics distribution based on
customer demand attributes.
Overall, these studies have demonstrated how
clustering urban areas and clients is a meaningful
method for a first-base idea for business sizing and
logistics planning. Most of them focus on clustering
customer demand based on geographical coordinates
or on client profiles.
This paper proposes a clustering approach for
food and parcel delivery services in Spain, that in-
corporates socio-economic indicators and urban net-
work features, based on the amount of demand from
specific small areas within a city. By analyzing the
clustering differences and similarities in operation
zones concerning the group of indicators used, our
study provides insights into depot performance and
decision-making in last-mile delivery planning.
2 DATA DRIVEN APPROACH
Building depot clustering algorithms requires know-
ing specific metrics of stores performance. Using de-
livery electric and combustion motorbike data from
food and parcel delivery services, in a prior work the
process to link that data to specific depots and extract
performance metrics and statistics of each service was
made (Arregui et al., 2024). The result was the de-
tection of many food and parcel delivery services in
Spain, the motorbikes that operate within each ser-
vice, geolocated trips, and delivery data of each mo-
torbike. The data used in this work is from 2024 and
overall, we have detected 854 parcel and 579 food de-
livery services.
Additionally, this data is enhanced with open data
population indicators and geospatial information of
the urban areas.
2.1 Depot’s Performance Metrics
After the corresponding data cleaning and processing,
the obtained daily performance metrics of each depot
are the following:
• Number of bikes
• Time of use of each bike
• Distance covered by each bike
• Number of deliveries per km
• Consumption per km
• Average delivery radius
• Maximum delivery radius
• Average time per trip
• Number of deliveries per trip
• Total number of deliveries
With this data, we are capable of knowing the de-
livery demand in every area of a city and the fleet met-
rics, therefore we are able to use this information as
a benchmark for future service planning. The depot
clustering analysis increases the usability of this in-
formation, and it relies on socio-economic and net-
work features.
2.2 Socio-Economic Data
In Spain, the National Statistics Institute (Instituto
Nacional de Estad
´
ıstica, 2024) offers insights into
many social, demographic, and economic indicators
with a high granularity. These indicators show, for
instance, inhabitants, genre, origin, educational level,
working status, marital status, and housing. The data
is updated to the year 2022. The full list of indicators
is depicted in Table 1.
2.3 Network Features
The heterogeneity of urban areas can be captured us-
ing Open Street Map (OSM) data enhanced with ele-
vation data. We can obtain interesting geospatial indi-
cators to cluster the services based on metrics such as
Planning Delivery Services: Depot Clustering Based on Socio-Economic Indicators and Geospatial Metrics
173